the Creative Commons Attribution 4.0 License.
the Creative Commons Attribution 4.0 License.
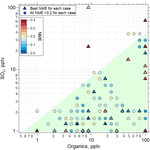
The potential role of organics in new particle formation and initial growth in the remote tropical upper troposphere
Agnieszka Kupc
Christina J. Williamson
Anna L. Hodshire
Jan Kazil
T. Paul Bui
Maximilian Dollner
Karl D. Froyd
Kathryn McKain
Andrew Rollins
Gregory P. Schill
Alexander Thames
Bernadett B. Weinzierl
Jeffrey R. Pierce
Charles A. Brock
Global observations and model studies indicate that new particle formation (NPF) in the upper troposphere (UT) and subsequent particles supply 40 %–60 % of cloud condensation nuclei (CCN) in the lower troposphere, thus affecting the Earth's radiative budget. There are several plausible nucleation mechanisms and precursor species in this atmospheric region, which, in the absence of observational constraints, lead to uncertainties in modeled aerosols. In particular, the type of nucleation mechanism and concentrations of nucleation precursors, in part, determine the spatial distribution of new particles and resulting spatial distribution of CCN from this source. Although substantial advances in understanding NPF have been made in recent years, NPF processes in the UT in pristine marine regions are still poorly understood and are inadequately represented in global models.
Here, we evaluate commonly used and state-of-the-art NPF schemes in a Lagrangian box model to assess which schemes and precursor concentrations best reproduce detailed in situ observations. Using measurements of aerosol size distributions (0.003 < Dp < 4.8 µm) in the remote marine troposphere between ∼0.18 and 13 km altitude obtained during the NASA Atmospheric Tomography (ATom) mission, we show that high concentrations of newly formed particles in the tropical UT over both the Atlantic and Pacific oceans are associated with outflow regions of deep convective clouds. We focus analysis on observations over the remote Pacific Ocean, which is a region less perturbed by continental emissions than the Atlantic. Comparing aerosol size distribution measurements over the remote Pacific with box model simulations for 32 cases shows that none of the NPF schemes most commonly used in global models, including binary nucleation of sulfuric acid and water (neutral and ion-assisted) and ternary involving sulfuric acid, water, and ammonia, are consistent with observations, regardless of precursor concentrations. Through sensitivity studies, we find that the nucleation scheme among those tested that is able to explain most consistently (21 of 32 cases) the observed size distributions is that of Riccobono et al. (2014), which involves both organic species and sulfuric acid. The method of Dunne et al. (2016), involving charged sulfuric acid–water–ammonia nucleation, when coupled with organic growth of the nucleated particles, was most consistent with the observations for 5 of 32 cases. Similarly, the neutral sulfuric acid–water–ammonia method of Napari (2002), when scaled with a tuning factor and with organic growth added, was most consistent for 6 of 32 cases. We find that to best reproduce both nucleation and growth rates, the mixing ratios of gas-phase organic precursors generally need to be at least twice that of SO2, a proxy for dimethyl sulfide (DMS). Unfortunately, we have no information on the nature of oxidized organic species that participated in NPF in this region. Global models rarely include organic-driven nucleation and growth pathways in UT conditions where globally significant NPF takes place, which may result in poor estimates of NPF and CCN abundance and contribute to uncertainties in aerosol–cloud–radiation effects. Furthermore, our results indicate that the organic aerosol precursor vapors may be important in the tropical UT above marine regions, a finding that should guide future observational efforts.
- Article
(3175 KB) - Full-text XML
-
Supplement
(13040 KB) - BibTeX
- EndNote
A majority of particles found in the atmosphere are formed through gas-to-particle conversion (i.e., nucleation) from clustering of low-volatility vapors (Gordon et al., 2017; Pierce, 2017). While the formation of these molecular clusters appears to take place almost everywhere and at all times in the atmosphere (Kerminen et al., 2018), the formation of thermodynamically stable aerosol particles with diameters (Dp) of 1.5 nm requires favorable conditions in terms of temperature, availability of condensable vapors, and the background of pre-existing bigger particles that compete for condensing vapors, and so this formation may not occur in every atmospheric environment (Kulmala et al., 2014). Most of these newly formed particles are lost by coagulation with larger particles, and they do not contribute to particle number (Westervelt et al., 2014). A subset of the nucleated particles grows by condensation to become larger particles with reduced Brownian motion and hence lower coagulational loss rates (e.g., Pierce and Adams, 2007). Particles with Dp≳50 nm can serve as cloud condensation nuclei (CCN) at supersaturations found in typical marine cumulus and stratocumulus clouds (Quinn et al., 2008), increasing droplet number concentrations and cloud albedo and thus indirectly affecting the Earth's radiative budget (Twomey, 1974; IPCC, 2013).
The tropical upper troposphere (UT) is known to be a major source region of new particles (e.g., Clarke, 1993; Brock et al., 1995; Clarke and Kapustin, 2002; Weigel et al., 2011; Williamson et al., 2019). This strong aerosol production is believed to be linked with frequent deep convection in this region. The mechanism proposed by Clarke (1992) involves the formation of new particles in the UT from convectively lifted and cloud-processed boundary layer air. At the conditions of cold temperatures, high photolytic fluxes, and low concentrations of pre-existing aerosol particles found in the outflow of deep convection at altitudes >8 km, aerosol precursor gases that may survive convective transport and scavenging can oxidize and nucleate new particles which then grow to CCN sizes as they descend in the gradually subsiding air that compensates for the upward convection. Raes (1995) used a box model to determine that observed concentrations of CCN in the remote marine boundary layer (MBL), as well as their temporal stability, could not be explained without a source of particles being entrained from the free troposphere (FT). Clarke et al. (2006) estimated that entrainment from the FT provides 35 %–80 % of the CCN flux into the MBL over latitudes between 40∘ S and 40∘ N with the rest coming from sea salt aerosol. More recently, Quinn et al. (2017) found that at ∼ 0.5 % supersaturation, the accumulation mode aerosol, composed primarily of sulfate compounds rather than sea-spray particles, provides ∼ 70 % of the CCN population throughout the MBL of the tropics and midlatitudes, and they suggested that these particles originate from the FT.
Despite the climatic importance of new particle formation (NPF) in the tropical UT, the chemical mechanisms are poorly understood (e.g., English et al., 2011). This lack of understanding is driven by the fundamental complexity and variability of the atmosphere, the range of potential chemical species and mechanisms that could lead to NPF and subsequent growth of the newly formed particles to CCN, and the difficulty in obtaining observations of processes occurring in remote areas, at high altitudes, and over timescales ranging from minutes (NPF) to weeks (condensational growth during gradual descent). Together, these issues have made it difficult to validate NPF schemes used in global models and have hindered our ability to reduce uncertainty in aerosol–cloud–radiation interactions.
Williamson et al. (2019) showed that three of four global models examined in their study underestimated the magnitude of NPF in the tropical Pacific UT, and all failed to accurately simulate the abundance of CCN-sized particles in the lower troposphere of the same region (the fourth model significantly overestimated aerosol loadings throughout the troposphere). None of these models used a NPF scheme involving organics, and the three models may lack sufficient precursor vapors for growth, in addition to other deficiencies. Previous model studies (e.g., Kazil et al., 2010; Yu et al., 2010; Zhang et al., 2010; Zhu et al., 2019) show that the choice of NPF mechanism can drive substantial changes in the predicted abundance and spatial distribution of particles. While Westervelt et al. (2014) suggested that the global-mean boundary layer CCN are not very sensitive to the number of particles formed in the UT due to the dampening effects of coagulation (i.e., more nucleation leads to faster coagulational losses), different choices of NPF mechanisms in models might alter the spatial and temporal pattern of NPF and thus affect the spatial distribution and magnitude of CCN abundance. It is clear that accurate simulation of NPF and growth processes is essential to adequately represent particle size distributions and their spatial distribution in global models and improve predictions of aerosol–cloud–radiation effects (Hodshire et al., 2018; Williamson et al., 2019).
Field measurements have shown that sulfuric acid is a key component in atmospheric NPF in the continental boundary layer (e.g., Weber et al., 1997; Riipinen et al., 2007; Sihto et al., 2006). Several nucleation schemes involving sulfuric acid have been used in global models as a consequence. These include the activation nucleation scheme that depends on sulfuric acid only (Kulmala et al., 2006); binary schemes that involve sulfuric acid and water to form new particles (e.g., Vehkamäki et al., 2002); or ternary schemes in which sulfuric acid, water, and ammonia condense to form new particles (e.g., Napari et al., 2002). The activation nucleation scheme, however, is an empirical formulation tuned to midlatitude continental boundary layer observations, so it is appropriate to use only there. Binary NPF has been suggested to be favored in the remote tropical UT due to cold temperatures, high relative humidity (RH), and the availability of supersaturated sulfuric acid (Clarke, 1992; Brock et al., 1995; Clarke and Kapustin, 2002). Ion-assisted nucleation of sulfuric acid and water clusters has been identified as a potential pathway for binary NPF (Kirkby et al., 2011; Lovejoy et al., 2004; Kazil and Lovejoy, 2007; Raes et al., 1997; Yu 2010). Ions stabilize the molecular clusters so that nucleation can occur at warmer temperatures and lower nucleating-vapor concentrations (Yu, 2010).
Recent observations of the composition of molecular clusters present during NPF have highlighted the role that organics may play (Kulmala et al., 2013; Smith et al., 2004). Murphy et al. (2006) and Froyd et al. (2009) found that larger particles (Dp>0.15 µm) in the UT contained significant organic matter that was likely secondary, which suggests that condensable gas-phase organic compounds are present in the UT. Andreae et al. (2018) postulated that biogenic volatile organic compounds carried from the boundary layer to the UT by deep convection and oxidized to form condensable species over the Amazon are responsible for NPF observed in this continental UT region. Weigel et al. (2011) also suggested that organics might contribute to NPF events observed in the UT. Other nucleation processes combining sulfuric acid with ammonia (Kürten et al., 2016; Merikanto et al., 2007), amines (Almeida et al., 2013), diamines (Jen et al., 2016), or organics (Kulmala et al., 2006; Metzger et al., 2010; Riccobono et al., 2014) or processes with organics alone (Kirkby et al., 2016; Bianchi et al., 2016) have been proposed to explain some field and laboratory observations of NPF, primarily at warmer temperatures and continental locations. In a modeling study, Zhu et al. (2019) found that pure organic nucleation from biogenic volatile organic compounds could be an important source of particles, especially in the UT of modern-day pristine, continental environments and during the preindustrial period.
Because there have been no in situ observations of the composition of molecular clusters and nanoparticles found in convective outflow in the UT, it is difficult to ascertain which of these varied mechanisms, if any, contribute to NPF in the remote FT. In this study, we use unique observations obtained during the Atmospheric Tomography (ATom), a multi-year airborne program to measure gas and aerosol properties of the remote troposphere over both the Atlantic and Pacific oceans across four seasons. Recently formed particles observed in the tropical UT were linked to recent outflow from deep convection. We use box models constrained by trajectory calculations to evaluate how well different NPF formation mechanisms can simulate the observed particle size distributions. We perform extensive model sensitivity studies to determine which nucleation mechanisms and initial precursor mixing ratios allow for the model to match observed size distributions.
To establish a link between convection and NPF and to explore the processes that govern NPF and initial growth in the tropical and subtropical free troposphere over the Pacific Ocean, we couple measured size distributions between 2.6 nm and 4.8 µm in diameter from the four ATom missions with calculated air mass backward trajectories and two box models. The backward trajectories identify air masses potentially influenced by recent convection. We compare our simulations with in situ ATom observations of aerosol size distributions. We examine which nucleation schemes best explain the observations and evaluate whether observed sulfur precursors (SO2 and dimethyl sulfide (DMS)) can explain the NPF and the particle's initial growth. In one model, we simulate particle formation by neutral and charged binary and ternary schemes, as well as a neutral organics scheme, and we also add organics for initial growth of the particles in all schemes. In an additional model, we form particles using both neutral and charged binary schemes.
2.1 The Atmospheric Tomography mission
The NASA Atmospheric Tomography (ATom) mission was an airborne global survey that used the NASA DC-8 research aircraft to map for the first time the composition of the remote atmosphere over both the Pacific and Atlantic basins (∼ 82∘ N to ∼ 86∘ S; Fig. 1) in nearly continuous ascents and descents between ∼ 0.18 and 13 km in all four seasons (July–August 2016, January–February 2017, September–October 2017, and April–May 2018). The primary objectives of the mission were to examine the composition of the remote atmosphere to improve understanding of photochemical mechanisms for reactive and long-lived gas-phase species and to identify the abundance, distribution, and sources of aerosol particles in the remote marine troposphere (Wofsy et al., 2018).
2.2 Measurements
A suite of fast time response (1 Hz) particle counters and optical particle size spectrometers were used to measure dry size distributions between 2.6 nm and 4.8 µm in diameter (Brock et al., 2019). Two nucleation-mode aerosol size spectrometers (NMASSs; Williamson et al., 2018), each consisting of five continuous-flow condensation particle counters (CPCs) with different fixed cut-off diameters (i.e., diameters at which each CPC detects 50 % of the incoming particles) between 3.2 and 59 nm, measured particle number concentration. Two optical aerosol counters, an ultra-high-resolution sensitivity aerosol spectrometer (UHSAS; Kupc et al., 2018), and a laser aerosol spectrometer (LAS; Froyd et al., 2019) measured particle size distributions from 0.06 < Dp < 1 µm and 0.12 < Dp < 4.8 µm, respectively. All concentrations reported here are corrected to standard temperature and pressure (STP), 1013 hPa and 273.15 K. The NOAA Particle Analysis by Laser Mass Spectrometry (PALMS) instrument measured the composition of individual aerosol particles (Froyd et al., 2009, 2019). For this study, the PALMS size range is restricted to 0.125–1.5 µm. Due to inlet sampling artifacts (Weber et al., 1998; Murphy et al., 2006), cloudy periods were removed from the analysis. Clouds were detected using a second-generation cloud, aerosol, and precipitation spectrometer (CAPS) mounted under the wing, which also measured coarse aerosols >0.5 µm (Dollner et al., 2021) at ambient conditions.
Temperature, pressure, and wind speed with high time resolution (1 Hz) were measured with accuracies of ±0.3 K, ±0.3 hPa, and ±1.0 m s−1, respectively (Scott et al., 1990). Highly sensitive sulfur dioxide (SO2) measurements were made during ATom 4 using laser-induced fluorescence (Rollins et al., 2016; Rollins et al., 2017) with a precision (1σ) of 1–2 pptv at 10 s and an overall uncertainty of ± (9 % + 2 pptv). Laser-induced fluorescence was used to measure OH and HO2 simultaneously (Faloona et al., 2004; Brune et al., 2020) with an accuracy of ±35 %. Measurements of carbon monoxide (CO) were made using a Picarro G2401m (Chen et al., 2013) with a precision (1σ) of 2–3 ppb at 10 s and an average uncertainty of 4 ppb. All data used in this analysis can be found in Kupc et al. (2021).
2.3 Air mass backward trajectories and convective influence
To identify air in the UT influenced by recent deep convection, we calculated 10 d air mass backward trajectories using the Bowman trajectory model (Bowman, 1993) driven with meteorological fields (3 hourly, 0.25∘ horizontal resolution) from the National Centers for Environmental Prediction (NCEP, 2015) Global Forecast System (GFS). Trajectories were also run with MERRA2 and ERA5 reanalysis meteorology and the results were similar. Meteorological products from NCEP interpolated to the aircraft flight track agreed best with quantities measured on the aircraft during ATom, so all analyses were done using the trajectories based on the NCEP data.
A cluster of 245 trajectories was initialized within a grid (0.3∘ × 0.3∘ × 20 hPa; Fig. S1 in the Supplement) centered around the DC-8 flight track location every minute of flight (Fig. 1). The backward trajectory time step was 3 h, based on the reanalysis data, while a time step of 15 min interpolated from the 3 h reanalysis data was used for box model simulations. Uncertainty in the backward trajectory locations is represented by the 3-D spread in the trajectory cluster. The vertical uncertainty is estimated as the standard deviation in pressure (hPa) of the trajectory cluster at each time. The horizontal uncertainty is estimated using a probability grid based on the trajectory cluster in longitude and latitude at each time (Fig. S1), where probability grid refers to the number of trajectories at each time that are within each latitude–longitude grid box (2∘ × 2∘). For instance, if 24 of the trajectories are within a certain grid box at a certain time, then the probability for that grid box is ∼ 10 % (24/245). The probability that air sampled by the aircraft was influenced by deep convection was calculated based on coincidences of the backward trajectory cluster with satellite-derived cloud locations and characteristics such as cloud top and base pressure levels (NASA Langley Cloud and Radiation Research, 2018). To isolate deep convection, only clouds with vertical extent >5 km were considered in the convective influence (CI) calculation. The CI probability is the fraction of the trajectories in each cluster that intersected a convective system within a distance tolerance of 0.15∘ (∼ 10–15 km) and for which the RH with respect to liquid water (RHw) of the trajectory was >50 %. If the CI probability determined in this manner was >95 %, we assume that the aircraft was sampling air strongly influenced by deep convection.
2.4 Description of models
We use two independent aerosol nucleation and growth box models to test if different nucleation schemes are consistent with observations, following the trajectories from convective outflow to the location of the aircraft. These two models are conceptually similar but differ in size resolution and their support for different nucleation mechanisms. Our primary model, the TwO-Moment Aerosol Sectional (TOMAS; Adams and Seinfeld, 2002; Pierce and Adams, 2009; Pierce et al., 2011) includes both neutral and charged mechanisms. The neutral mechanisms include sulfuric acid and water (binary scheme; Vehkamäki et al., 2002); sulfuric acid, water, and ammonia (ternary scheme; Napari et al., 2002); and sulfuric acid with organics (organics scheme; Riccobono et al., 2014; Yu et al., 2017). The charged mechanism is as in Dunne et al. (2016), which quantifies NPF in terms of sulfuric acid, ammonia, and ion concentration (also including neutral pathways). In addition to testing the role of organics in nucleation and growth, we also test the influence of organics on aerosol initial growth when added as a condensing species following the nucleation of particles formed by each of the nucleation schemes in TOMAS, as described in Sect. “The TOMAS box model”.
We also use the Model of Aerosols and Ions in the Atmosphere (MAIA; Lovejoy et al., 2004; Kazil and Lovejoy, 2007) to test ion-assisted nucleation of sulfuric acid and water. Since ion-assisted nucleation simulations using MAIA did not explain the observed size distributions in our work, we focus on the TOMAS model description and results, and the details on the MAIA model are in the Supplement (Sect. S1). Some features common to both models are described below.
The MAIA and TOMAS box models are constrained to follow the meteorological conditions along the trajectories. They are initialized at the point where the trajectories intersect deep convection and proceed forward in time until reaching the aircraft sampling location and time. The temperature, pressure, and RHw vary based on the trajectory. We vary the initial SO2, NH3, and organic aerosol precursors in TOMAS and SO2 in MAIA (Tables 1 and S1 in the Supplement) to see which initial values of these species allow for the best matches to the observed size distribution. We note that neither model explicitly simulates DMS, which is likely to be an important aerosol precursor through its oxidation to form SO2 and subsequently H2SO4, as well as through its oxidation to methanesulfonic acid (MSA; Hodshire et al., 2019), which is a condensing species that may also be able to participate in NPF (Bork et al., 2014; Chen et al., 2015; Chen and Finlayson-Pitts, 2017). Previous analyses have shown that most of the observed reactive gas-phase sulfur above the boundary layer is in the form of SO2 (Veres et al., 2020). In this work, both models are initialized with a measurement-based pre-existing background aerosol population that acts as a sink for condensable vapors and small particles (see Sect. 2.5). Nucleation-mode particles are initialized at zero concentration. We calculate the OH diurnal cycle using a prescribed peak noontime value based on observations of OH on the DC-8 aircraft (Sect. 2.5 and Fig. S3). The OH concentration along the trajectory and the resulting production rate of H2SO4 from oxidation of SO2 are then calculated. We ignore possible enhanced OH due to cloud reflectivity in the vicinity of convective outflow and reduced OH from shading by higher clouds.
The TOMAS box model
The TOMAS model simulates particle nucleation, condensation, and coagulation in 43 logarithmically spaced particle size bins, which represent dry diameters from 0.7 nm–10 µm. TOMAS tracks the total aerosol number and mass of each species for each size bin. The simulated aerosol species are sulfate, ammonia, a representative oxidation product of biogenic organics, and water. In these simulations, neutral sulfuric acid–water nucleation is based on Vehkamäki et al. (2002), neutral sulfuric acid–water–ammonia nucleation is from Napari et al. (2002), ion-induced and neutral sulfuric acid–water and sulfuric acid–water–ammonia nucleation is from Dunne et al. (2016), and neutral sulfuric acid–organic nucleation is from Riccobono et al. (2014).
Vehkamäki et al. (2002), referred to here as VEHK, describe a parametrization for neutral sulfuric acid–water particle formation based on a classical nucleation model. They use a model for the hydrate formation relying on ab initio calculations of small sulfuric acid clusters and on experimental data for vapor pressures and equilibrium constants for hydrate formation. The parameterized formulas are valid at temperatures between 230.15 and 305.15 K, RHw from 0.01 %–100 %, and sulfuric acid concentrations from 104–1011 cm−3. Temperatures along the trajectories ranged between 218 and 252 K and thus were below the applicable temperatures of this nucleation scheme in 18 out of 32 simulated cases. In these low-temperature cases, we assume the temperature to be 230.15 K (i.e., we do not extrapolate beyond the bounds of the parameterization). When sulfuric acid concentration was < 104 molec. cm−3, the model assumes a nucleation rate of zero, and it limits the maximum concentration of gas-phase sulfuric acid to 1011 molec. cm−3.
In the Napari et al. (2002) scheme, referred to here as NAPA, the nucleation rate is parameterized using four variables: temperature, RHw, H2SO4 concentration, and NH3 mixing ratio. The parameterization is valid for temperatures from 240–300 K, RHw from 5 %–95%, sulfuric acid concentrations from 104–109 molec. cm−3, ammonia mixing ratios from 0.1–100 ppt, and nucleation rates from 10−5–106 cm−3 s−1. When temperature is < 240 K or >300 K (25 out of 32 simulated cases) and/or RHw is outside of the limits stated above, the model assumes the temperature to be 240 or 300 K and RHw to be 5 % or 95 %, respectively. When the sulfuric acid concentration is < 104 molec. cm−3, the model assumes a nucleation rate of zero, and it limits the maximum concentration of gas-phase sulfuric acid to 109 molec. cm−3.
This parametrization accounts only for hydrate formation and neglects the formation of ammonium bisulfate and its effect on nucleation rate (Zhang et al., 2010). It overpredicts the effect of ammonia on nucleation when compared with laboratory measurements (Zhang et al., 2010). Merikanto et al. (2007) showed that nucleation rates based on the NAPA scheme were biased high, and Lucas and Akimoto (2006) indicated that this scheme in a global model predicted unrealistically high nucleation rates throughout the troposphere. To address these issues, Westervelt et al. (2013) and Jung et al. (2010) used a nucleation rate tuning factor of in the boundary layer and found that the model produced a reasonable agreement with observations. In this study we performed simulations both with this tuning factor (referred to as NAPAt) and without it (NAPA).
In Dunne et al. (2016), referred to here as DUN, the inorganic nucleation rates determined experimentally in the CLOUD (Cosmics Leaving Outdoor Droplets) chamber are parameterized in four dimensions: sulfuric acid, ammonia, temperature (208–292 K), and ion formation rates (0–75 cm−3 s−1). Humidity is not included in this parametrization. The overall nucleation rate is given by the sum of the individual processes:
where Jb,n is the binary neutral rate, Jb,i is the binary ion-induced rate, Jt,n is the ternary neutral rate, Jt,i is the ternary ion-induced rate, n− is the steady state concentration of small negative ions, and [H2SO4] and [NH3] are gas concentrations (cm−3). In this paper, we investigated separately ion-induced binary (DUN with NH3 set to 0) scheme as well as the overall nucleation scheme (DUN) given by the sum of the above.
Sulfuric acid–organic nucleation was simulated using the scheme described in Riccobono et al. (2014), referred to here as RIC. While this scheme was developed to represent terrestrial organic species, we use it here as a surrogate for marine organic compounds, because there are no specific mechanisms that have been developed for remote marine-sourced precursors.
The model includes a secondary organics aerosol precursor (SOAP; MW = 200 g mol−1) variable, which can oxidize to form a condensable aerosol species. This species can both participate in nucleation in the RIC scheme and condense onto particles in all schemes studied here. We assume a reaction rate constant for the oxidation of biogenic organic species against OH is ∼ cm3 s−1 molec.−1, which is roughly an average reaction rate of non-methane alkanes according to Table 1 of Atkinson and Arey (2003). This rate constant gives a SOAP lifetime of ∼ 2 d for a typical diurnally averaged UT OH concentration of 2×106 cm−3. The yield of SOAP to secondary organic aerosol (SOA) is set to 1, which allows us to use SOAP as a simple, tunable variable to determine how much SOA may be necessary to match observed aerosol formation and growth. We use the SOAP oxidation product (i.e., condensable organic) in the RIC scheme, but also use it to explore the effects of organics on new particle growth for each of the nucleation schemes (Riipinen et al., 2011).
Table 1Ranges of parameters used for sensitivity studies in the TOMAS box model. Values varied to match the observed size distribution are in italic.
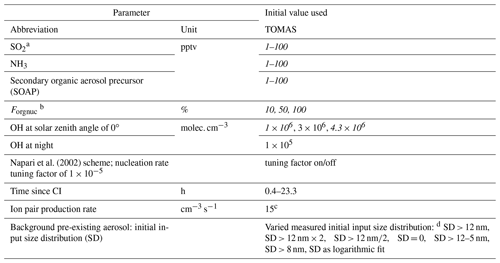
a SO2 measured on ATom 4 only. b Fraction of SOAP participating in nucleation when using Riccobono et al. (2014) in TOMAS. c Value typical for the tropical upper troposphere (Dunne et al., 2016). d Initial background aerosol size distribution was varied: SD > 12 nm means background SD as described in the text was used to initiate the model; SD > 12 nm × 2 means background SD multiplied by 2; SD > 12 nm∕2 means SD divided by 2; SD = 0 means no background aerosol; SD > 12–5 nm means SD was shifted by 5 nm to smaller diameters; SD > 8 nm means measured background SD > 8 nm was used as initial SD, where SD refers to the number size distribution dN∕dlog 10Dp.
In the RIC mechanism, nucleation occurs when only a fraction of the oxidation products of biogenic organic compounds (BioOxOrg in the terminology of the RIC mechanism), formed from SOAP oxidation, are able to form stabilized clusters. The formation rate dependence on sulfuric acid and BioOxOrg concentration is given by a fit to experimental data in the form
where JORG is the formation rate (cm−3 s−1) of stable particles with diameters ∼ 1.7 nm, kNUC is the nucleation rate constant with a value of cm6 s−1 at 278 K and RHw at 39 %, BioOxOrg represents concentration of later-generation oxidation products of biogenic monoterpenes (cm−3), and the exponents p=2 and q=1 represent the power law dependence of JORG upon the concentrations of sulfuric acid and BioOxOrg.
Using the RIC scheme, we test the effect of different fractions of condensable organic formed from SOAP oxidation. This fraction Forgnuc represents the fraction of the condensable BioOxOrg that may participate in nucleation by stabilizing the cluster. The value of Forgnuc does not affect the condensation of organics onto already-nucleated or pre-existing particles. Using Forgnuc allows us to decouple the possible role of organics in nucleation vs. their role in subsequent condensational growth.
Since the RIC scheme does not consider the possible effect of temperature on the nucleation rate, we modify the nucleation rates predicted in Eq. (5) using the temperature dependence (270–310 K) for this nucleation rate from Yu et al. (2017)
where fT is the nucleation rate scale factor accounting for the temperature dependence, and ΔH is the change in enthalpy of −38.3 kcal mol−1 associated with the critical cluster formation. We assume that ΔH is constant throughout our full temperature range.
One of the limitations of our box modeling effort is that the temperatures along the trajectories ranged between 218 and 252 K, often below the applicable temperatures of the three nucleation schemes: VEHK, NAPA, and RIC (Table S2). We would expect faster nucleation rates at the lower trajectory temperatures than are simulated by these schemes (e.g., Yue and Hamill, 1979). Using VEHK and NAPA schemes below their lower temperature limit means forcing them to their lowest rated temperature 230.15 and 240 K, respectively. This in turn may result in underestimating particle concentration and size. This bias for cold cases means that VEHK and NAPA schemes may predict SO2 and organic precursors that would be anomalously high. In the RIC scheme the temperature dependence of Yu et al. (2017) is not experimentally verified down to the tropical UT temperatures. Thus, we tested the impact of changing the ΔH by ±3 kcal mol−1 (Fig. S2). We also have not explored the organic-only nucleation scheme by Kirkby et al. (2016).
2.5 TOMAS input data
Measured and estimated inputs needed to initialize the TOMAS box model (Adams and Seinfeld, 2002; Pierce and Adams, 2009; Pierce et al., 2011) are given in Table 1. TOMAS was configured to use measured size distributions (>12 nm) in discrete bins. Each input in Table 1 represents the initial conditions present at the start of the simulation (t0). Hence, condensing vapor in the gas phase can contribute both to the formation and growth of new particles and growth of the pre-existing background aerosol.
We expect the output of the TOMAS model to be sensitive to the temperature dependence of nucleation rates; the type and number of organic compounds; SO2, OH, and NH3 mixing ratios; and the pre-existing background aerosol into which the convective outflow is mixed. The variability of the simulated aerosol size distribution to various initial conditions was examined by conducting sensitivity simulations (Table 1) on SO2, NH3, OH, background aerosol size distribution, organics added for initial growth (e.g., SOAP), and on the RIC scheme scale factor Forgnuc for organics involved in cluster formation.
The pre-existing aerosol is estimated based on the 1 min averaged size distributions for Dp>12 nm as observed at the aircraft location. The concentration of particles with Dp < 12 nm is set to zero under the assumption that these particles were produced by the new particle formation being modeled and were not present in the background air at the point of mixing with the air detrained from convection. The box model simulations do not explicitly account for the mixing of highly scavenged air detraining from convective outflow with surrounding UT air containing more aged aerosol (e.g., Weigel et al., 2011). We have undertaken sensitivity studies that vary the pre-existing background aerosol used as initial input parameter (Table 1).
The box model simulations were run forward in time from the moment the parcel exited the convection (t0) to the point of measurement by the aircraft (tfin), with temperature, pressure, and RHw varying as a function of time as determined from the backward trajectory. The change in RHw along the trajectory between the trajectory location at the cloud edge (t0) and the point of the aircraft location (tfin) for 32 simulated cases is presented in Fig. S67. The concentration of OH at solar zenith angle of 0∘ in the simulations was set to 3×106 molec. cm−3; however, we also tested OH concentrations of 1×106 and 4.3×106 molec. cm−3. These estimates agree well with aircraft-measured concentrations (Fig. S3) and with values given in Seinfeld and Pandis (2006). In TOMAS, OH is parameterized as a function of the cosine of the solar zenith angle, where the nighttime OH is 1×105 molec. cm−3. The solar zenith angle is calculated for the time, altitude, latitude, and longitude of the backward trajectories.
The SO2 and NH3 mixing ratios were varied between 1 and 100 pptv to explore a large range of plausible conditions. The evaluated SO2 range exceeds that measured on ATom 4 (Figs. S4 and S5) and covers the < 30 pptv mixing ratios previously reported in the UT over the central and western tropical Pacific (Thornton et al., 1997; Rollins et al., 2017, 2018). Organic aerosol precursors are unknown in the UT and were not directly measured; thus we explored a range of probable mixing ratios between 1 and 100 pptv.
2.6 Evaluating simulated size distributions
To determine which sets of parameters allow the models to reproduce the observed size distributions best, we evaluate every simulation against observations using the normalized mean error (NME) statistic of the first four moments (zeroth through third) of the size distribution for each model simulation as
where Si and Oi are ith moments of the simulated and observed size distributions, respectively (Hodshire et al., 2018).
The ith moment is defined as
where nN is the number of particles in size interval dDp and Dp is the diameter. Equation (9) is integrated over the diameter range from 2.6–20 nm, and Mi represents either Si or Oi. The zeroth moment (i=0) corresponds to the number of particles, the first moment (i=1) to the total diameter of particles (i.e., total aerosol length), the second moment (i=2) is proportional to the total surface area of particles, and the third moment (i=3) is proportional to the total volume of particles. A NME of 0 is a perfect fit between the simulation and observations; a NME of 1.0 indicates that the average bias of the zeroth through third moments between the simulation and observations is 100 %. As the NME is given as an absolute value, we do not discriminate between cases in which the model is underpredicting or overpredicting the moments on average. Since these moments are equally weighted, a low value of NME can be achieved only if the modeled size distribution accurately simulates both the shape and magnitude of the observed size distribution over the full range of sizes evaluated.
3.1 Observations
Our data show seasonally persistent high nanoparticle concentrations over the remote tropical UT (Fig. 2; Williamson et al., 2019). In this region, the highest concentrations of particles were in the nucleation mode (3–12 nm), which have a short lifetime and are the products of recent NPF. This tropical UT feature was observed in all ATom deployments over all four seasons, over both the Pacific and Atlantic basins. The concentrations of particles observed in the UT over the tropical Atlantic were lower than observed over the Pacific (Fig. S6). In this study, we focus on observations over the remote Pacific, which is a region less perturbed by continental emissions than the Atlantic (Figs. 3, S7 and S8).
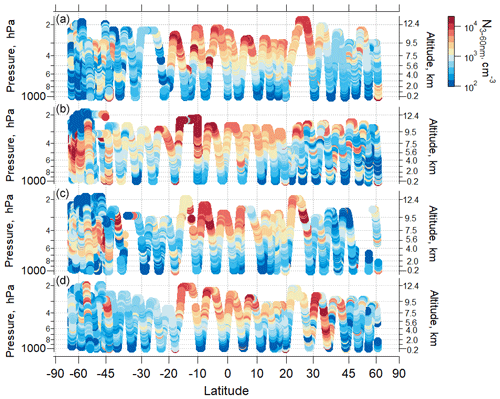
Figure 2Ambient pressure as a function of latitude colored by the measured number concentration of particles with Dp from 3–60 nm over the Pacific Ocean for (a) ATom 1, July–August 2016; (b) ATom 2, January–February 2017; (c) ATom 3, September–October 2017; and (d) ATom 4, April–May 2018). Periods of flight in clouds, over continents, and near airports have been removed.
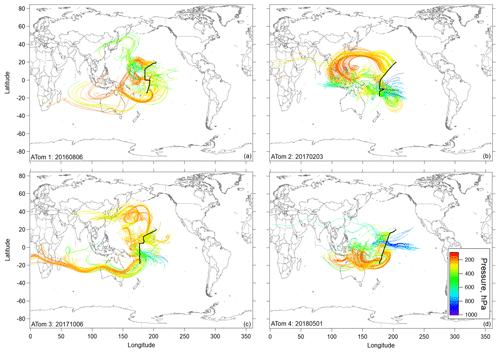
Figure 3Flight track and selected 10 d backward trajectories initiated for times in flight at pressures < 400 hPa (< ∼ 260 K) sampled along the DC-8 flight track during ATom missions 1, 2 (a, b), 3, and 4 (c, d) during the most tropical flight in each deployment (Hawaii–Samoa on ATom 1 and Hawaii–Fiji on ATom missions 2–4). Trajectories are colored according to the pressure along their pathway.
Previous studies (e.g., Clarke, 1992, 1993; Clarke et al., 1998, 2006; Brock et al., 1995; Weber et al., 1995; Raes et al., 1997; Thornton et al., 1997; Weber et al., 1998; Clarke and Kapustin, 2002; Twohy et al., 2002; Froyd et al., 2009; Borrmann et al., 2010; Weigel et al., 2011) have provided strong evidence of NPF in the tropical UT and its link to convective activity. However, these earlier studies did not provide such extensive, representative, and global-scale coverage of the remote marine troposphere over a wide range of altitudes and latitudes (Williamson et al., 2019). The ATom observations also provide accurate, sensitive state-of-the-art measurements of the chemical composition of the bulk aerosol and the abundance of hundreds of gas-phase species in all four seasons, making these observations the most comprehensive to date. However, no measurements were made during ATom of NH3, the highly oxygenated organic molecules that are likely aerosol precursors, or molecular cluster composition, and measurements of SO2 took place only during the fourth ATom deployment.
Ten-day backward trajectories in the region of NPF in the central Pacific showed transport primarily over the Pacific, with some possible terrestrial influence from the western Pacific region (Fig. 3). However, trajectories coming from the western Pacific generally stayed at high altitudes and did not show recent convective uplift from regions influenced by terrestrial sources. Further, CO and other continental tracers were at background levels over the Pacific, confirming little continental influence in the sampled air masses (Fig. S7), as opposed to the Atlantic (Figs. S6 and S7). Thus, the precursors of the recently formed particles are likely mostly marine in origin. The latter is also supported by the measurement of particle-phase methanesulfonic acid (MSA) that can be considered a tracer for maritime influence on the tropical UT (Fig. S9).
The observations and trajectory modeling show that newly formed particles were often associated with deep convection. Similar trajectory analyses, in terms of examining the history of the sampled air masses for interactions with deep convection, have been undertaken by Froyd et al. (2009) and Andreae et al. (2018). Here, using the CI probability criterion of 95 % to identify when the aircraft was sampling air recently influenced by convection (Sect. 2.3), as well as considering the latitude range 30∘ S–30∘ N and ambient temperatures < 260 K for ATom 1 and ATom 2, the shorter the time since convection, the higher the number of small particles (Fig. 4a–d). Such strong trends were not evident for ATom 3 and ATom 4, indicating that factors other than time since CI affect nucleation-mode concentrations. The more recent the convection, the smaller the diameter of the nucleation mode (Fig. 4e–h). These relationships are again strongest for ATom 1 and ATom 2 as well as ATom 4. Our hypothesis for these relationships is that with increasing time since CI, particles with diameters < 12 nm grew by condensation and coagulation and decreased in concentration by coagulation, leading to the decrease in nucleation-mode concentration and increase in diameter. A similar trend was observed over the Atlantic (Fig. S10 in the Supplement). The highest concentrations of nucleation-mode particles occurred during ATom 2 and were associated with the shortest times since CI.
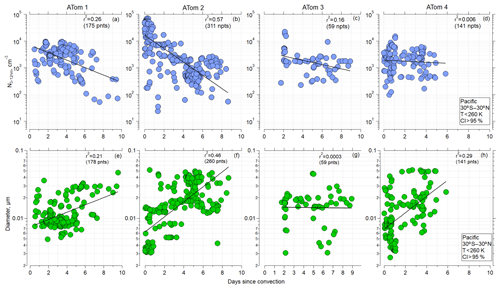
Figure 4(a–d) Concentration of nucleation-mode particles as a function of time since convective influence for ATom missions 1–4, over Pacific (30∘ S–30∘ N), T<260 K, and probability of convective influence >95 %, respectively. (e–h) Modal diameter of particles with Dp<0.06 µm as a function of time since convective influence (30∘ S–30∘ N) for ATom missions 1–4, respectively. Black line, used to guide the eye, represents the linear regression fitted to log-y values. A corresponding Pearson correlation coefficient r2 is indicated.
Air detraining from deep convection is likely depleted in pre-existing particles due to in-cloud removal, leading to a reduced condensation sink (CS) that enhances the likelihood of NPF (e.g., Clarke, 1992; Williamson et al., 2019). Figure 5 shows the concentration of measured nucleation-mode particles as a function of altitude for the Pacific basin over four ATom missions. The median concentration of nucleation-mode particles averaged from 30∘ S to 30∘ N is highest at altitudes >10 km, reaching ∼ 40 000 cm−3 (Fig. 5a), coinciding with the lowest values of CS>12 nm, which competes with NPF for condensing vapors. The CS term is calculated for particle diameters >12 nm and for the diameter range between 3 and 12 nm following Williamson et al. (2019). CS>12 nm serves as an estimate of the condensation sink prior to nucleation starting, and it is negatively correlated with the number of nucleation-mode particles (Fig. 5b), while the nucleation mode is positively correlated with CS3–12 nm (Fig. 5c). Over the Atlantic, the maximum concentration of nucleation-mode particles >8 km in altitude averaged from 30∘ S to 30∘ N, ∼ 3000 cm−3, is considerably smaller than over the Pacific, but the shape of the profile is similar (Fig. S11).
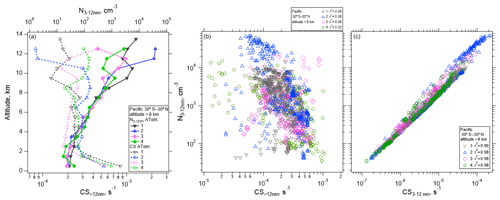
Figure 5(a) Vertical profile of the median number concentration of nucleation-mode particles (3–12 nm) and condensation sink (CS) averaged between 30∘ S–30∘ N as a function of altitude for the four ATom deployments. (b–c) One-minute average nucleation-mode particle concentrations at > 8 km in altitude as a function of CS>12 nm (b) and CS3–12 nm (c), between 30∘ S–30∘ N over the Pacific Ocean. Pearson correlation coefficient values (r2) are indicated in the legend.
Some variability in the strength of NPF and its dependence on CS>12 nm can be observed. In general, CS>12 nm is weakly negatively correlated (r2 between 0.08 and 0.36 depending on the ATom mission) with the concentration of nucleation-mode particles (Fig. 5b), as would be expected if NPF were competing with CS>12 nm for condensing vapors. When CS is dominated by small particles (CS3–12 nm), the correlation is strongly positive (r2 between 0.97–1, Fig. 5c). Factors other than CS are also important in controlling the concentrations of newly formed particles. These factors may include temperature and RHw, actinic flux, and OH that drive photochemical reactions that oxidize precursor species; the abundance of those precursor species in the air lifted by convection and in the background air; and the time since the air parcel exited a convective cloud (Figs. S12–S13).
3.2 Box model simulations
Case studies were selected for box model simulations based on specific criteria such as temperature and CI probability. We restrict the analysis to data taken nominally in the tropics and subtropics, between 30∘ S–30∘ N latitude. We consider the case for analysis by box modeling if the CI probability is >95 %, temperature at the point of measurement is < 260 K, and an aerosol number mode with a modal peak diameter < 12 nm is present (Table 2). We performed simulations for 32 cases randomly selected from the ATom 2 (20 out of 47 identified cases) and ATom 4 (12 out of 60 identified cases) datasets over the remote tropical Pacific from the total number of 109 cases with time since convection < 1 d (Table 2). Data from ATom 2 and ATom 4 were selected for simulations because these deployments had the most identified cases with time since CI < 1 d. During ATom 2, we observed the highest numbers of nucleation-mode particles, lowest condensation sink, and shortest time since convection (Fig. 5) among all missions. Measurements of SO2 mixing ratio were made only during the ATom 4 deployment, providing an important constraint for the box model simulations. We did not perform simulations on ATom 1 and ATom 3 data as there were only two and zero identified cases with time since CI < 1 d, respectively (Table 2).
Table 2Number of identified cases of recent NPF associated with CI for the Pacific (Atlantic in Table S4) between 30∘ N and 30∘ S latitude that meet the following criteria: T<260 K, CI > 95 %, and modal peak diameter < 12 nm.
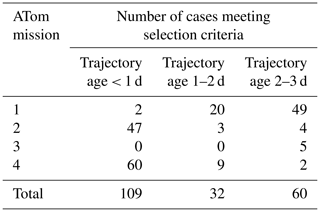
The correlation between nucleation-mode particles and time since CI was strongest in ATom 2 (Fig. 4), while CO levels, a proxy for continental influence, were the lowest for trajectory times < 1 d (Fig. S7). Although SO2 was not measured during ATom 2, we expect SO2 in this region in the UT to be < 30 pptv based on SO2 levels measured during ATom 4 and other missions in the Pacific (Figs. S4, S5).
The size distributions simulated by TOMAS were smoothed to avoid the artificial distortion of the distribution caused through size-bin emptying (Hodshire et al., 2019; Stevens et al., 1996). The latter and the smoothing technique are described in Sect. S2.
We performed box model simulations on the 32 selected cases using the range of values listed in Table 1. The success of each model simulation was evaluated using the NME described by Eq. (8). As an example using a single case, Fig. 6a shows the observed and simulated aerosol size distribution with the best NME obtained for each of the various nucleation schemes tested, along with the corresponding mixing ratios of SO2, NH3, or organics. Organics here refer to the SOAP oxidation product (i.e., condensable organic) that participates in nucleation in the RIC scheme (as BioOxOrg) and in the particle condensational growth in all schemes. The values of NME as a function of the mixing ratios of SO2, NH3, and organics for each nucleation scheme are also shown (Fig. 6b–j). The summary of each of the 32 simulated cases is presented in Table S4 and Figs. S15–S45. The simulations in Fig. 6 used the default OH scheme with a maximum concentration of 3×106 cm−3 at a solar zenith angle of 0∘ (Fig. S3). Sensitivity studies for maximum OH values of 1×106, 3×106, and 4.3×106 cm−3 are presented in Figs. S46–S50.
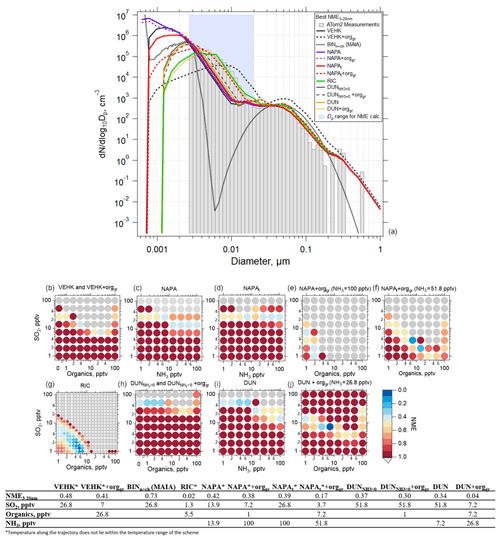
Figure 6Results of simulations using the TOMAS box model for an example case (ATom 2, 4 February 2017, 03:05:31–03:06:30 UTC; case identifier: sd486) where measurements were made 7.3 h following convective influence, and temperature along the trajectory varied between 218 and 226 K. (a) Observed (shaded bars) and simulated (lines) aerosol size distributions with best normalized mean error (NME) calculated for Dp between 2.6 and 20 nm (blue shading) for each of the NPF and growth schemes investigated. Best results from the MAIA box model ion-assisted + neutral binary nucleation scheme shown as a dotted black line. (b) NME between the modeled and measured size distribution for the VEHK scheme with varying organics mixing ratios for condensational growth. The color of the circle indicates the value of NME corresponding to a particular initial mixing ratio of SO2, NH3, or organics that varied between 0 and 100 pptv. Blue represents the best agreement, red poorer agreement, and grey the worst (NME > 1). There were 64 sensitivity tests. (c) As in (b) but for the NAPA scheme. (d) As in (c) but for the NAPAt scheme. (e, f) As in (c) and (d), respectively, but with NH3 fixed and varying organics for condensation growth. (g) As in (b) but for the RIC scheme, which provides the lowest NME. There were 400 sensitivity tests for this scheme. (h) As in (b) but for the DUN scheme with NH3 set to 0 (DUN). (i) As in (c) but for the DUN scheme. (j) As in (i) but with varying organics for condensation growth. The table presents the NME results for the corresponding size distributions in (a) and associated initial mixing ratios of gas-phase precursors.
TOMAS simulations using VEHK scheme substantially underpredict the observed tropical nucleation-mode number concentration, with resulting poor values of NME (Fig. 6). Sensitivity tests that vary the pre-existing initial (background) aerosol or completely remove background particles do not change the results significantly (Figs. S51–S53). Further, we find that changing initial input parameters such as SO2 and OH as indicated in Table 1 do not improve the NME for VEHK scheme (Figs. 6b and S46). Adding organics to grow particles nucleated by the VEHK scheme, while reducing NME slightly, does not provide adequate agreement with the observations. Varying the pre-existing initial aerosol or completely removing background particles in VEHK scheme with added organics may improve the fits for certain initial conditions (Fig. S52), making it plausible for better NME values if CS>12 nm was s−1 and SO2 and organics were 13.9 and 7.2 pptv, respectively, for this particular case. Out of 32 cases studied here, there is no case with NME < 0.2 for VEHK scheme. Similarly, the ion-assisted binary nucleation scheme of the MAIA box model does not provide good matches with observations (Table S4).
The NAPA scheme, both with (NAPAt) and without (NAPA) the tuning factor, did not significantly reduce the NME values from the VEHK results for this case (Fig. 6c). However, when organics were added to condense on the particles nucleated by this mechanism, the NME improves, resulting in six cases with NME < 0.2 (Table S4). The RIC nucleation scheme, updated by the temperature dependence of Yu et al. (2017), provides the best NME (NME = 0.02) for all the schemes investigated (Fig. 6f) for the case shown in Fig. 6. We explored this mechanism with six more sensitivity simulations, including various combinations of initial SO2 and organic mixing ratios, to see how sensitive NME is to small changes of initial precursor vapor mixing ratios. For the example case presented in Fig. 6a, organic mixing ratios < 10 pptv and SO2 mixing ratios < 5 pptv were sufficient to produce size distributions that matched the observations with good fidelity (NME = 0.02). Varying the scale factor of organics taking part in nucleation (Forgnuc) did not change the results significantly (Fig. S48). Nucleation can have strong self-regulating mechanisms, and these are likely contributing here. Increasing Forgnuc increases the nucleation rate initially in the high-Forgnuc simulations, which increases the condensation and coagulation sinks, which decreases the vapor concentrations (and hence nucleation and growth rates), and increases the coagulational loss rates. Each of these feedbacks lead to a buffering of changes to the nucleation mode. Westervelt et al. (2014) describes these buffering mechanisms in detail. Changing Forgnuc does not change the amount of organic material and sulfuric acid that condenses though, so ultimately the size distributions are relatively insensitive to changing Forgnuc because the buffering mechanism and total condensation remain approximately constant. The most recently developed NPF mechanism, the ion-induced sulfuric acid–water, referred here as DUN with NH3 set to 0, and the sulfuric acid–water–ammonia (DUN) nucleation scheme from Dunne et al. (2016), did not provide the lowest NME values among the schemes tested, although adding organics for initial growth of the nucleated particles improved the fits (NME = 0.04) (Fig. 6i, Table S4). The addition of organics resulted in best NME values for DUN in 5 out of the 32 cases simulated.
Overall, a reduction in NME when organics are added for initial particle growth was also observed for other schemes (Table S4). Out of 32 case studies, we found 6 cases when the NAPAt with organics for growth and the tuning factor applied gave lower NME values than all other schemes. However, four out of these six cases require SO2 or NH3 mixing ratios >50 pptv that exceed ATom 4 SO2 observations and literature values in the tropical UT for SO2 of < 30 pptv (Fig. S4; Rollins et al., 2017, 2018; Thornton et al., 1997; and for NH3 of <10 pptv; Höpfner et al., 2016; Feng and Penner, 2007; Adams et al., 1999).
Growth rates calculated based on the diameter of the leading edge and threshold value of cm−3 in any of the size bins below 12 nm were mostly between 0.1 and 3 nm h−1 for the RIC scheme (Fig. S71). The growth rates for all cases investigated here using RIC and other schemes are presented in Fig. S72.
Figure 7 shows the time evolution for particle number concentration, surface area, and volume for the nucleation, Aitken, and accumulation modes using the Riccobono et al. (2014) scheme for the same case as shown in Fig. 6 for the simulation with the lowest NME in Fig. 6g (SO2 = 1.3 pptv, organics = 5.5 pptv). There is rapid evolution of the nucleation mode and slower changes of the larger modes, and the model effectively matches the number, surface, and volume of the measured nucleation mode. In the case presented in Fig. 7b, the surface area measured on ATom is dominated by the nucleation-mode particles; however, although frequent, this is not a typical pattern among all other cases studied here. In general, in the cases with the highest surface area, values are split between nucleation, Aitken, and accumulation modes almost equally (Table S5, Figs. S68–70).
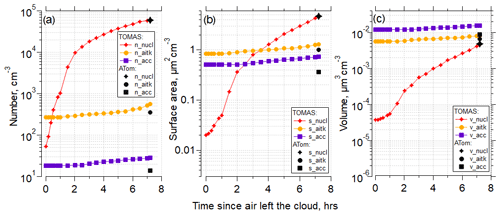
Figure 7(a) TOMAS box model simulation of the case (case identifier: sd486) shown in Fig. 6 for the lowest (best) NME for the RIC scheme, showing number concentration of the nucleation (3–12 nm), Aitken (12–60 nm) and accumulation (60–500 nm) modes as a function of time since the air parcel exited the cloud to the time of measurement by the aircraft. Black symbols indicate values at the point of measurement. The measured Aitken and accumulation mode values from the observations were used as approximate initial conditions for the model simulation and are shown at time t=fin. (b) As in (a) but for surface area concentrations. (c) As in (a) but for volume concentrations. Conditions for the simulations were diurnally varying OH concentrations with solar zenith angle. Initial SO2=1.3 pptv, and initial organics = 5.5 pptv.
In 21 out of the 32 cases for which multiple box model simulations were run, the sulfuric acid–organic nucleation scheme of Riccobono et al. (2014) produced lower (better) values of NME than the other parameterizations tested (Table S4). Two of those 21 best NME cases for RIC were tied with NAPA and NAPAt, both with organics added for initial particle growth. The remaining 12 best NMEs came from two different ternary nucleation schemes with added organics for growth of particles. These schemes were the NAPA or NAPAt (or the DUN with both charged and neutral channels). The majority of these ternary cases, however, required initial conditions of NH3 of 52 pptv or more, much greater than the mixing ratios expected at these locations in the UT (Höpfner et al., 2016). While we are limited by the lack of direct observations of NH3, amines, and condensable organic species, it is plausible that there are enough of these compounds – a few to tens of pptv – to participate in ternary nucleation and subsequent growth to be consistent with the ATom measurements. Further, regardless of the available SO2, the results strongly suggest that binary sulfuric acid–water nucleation, whether ion-assisted or neutral (and whether coupled with organic growth or not), generally cannot explain the ATom observations.
The findings for the case of organic-mediated NPF are summarized in Fig. 8, where we show the SO2 and organic precursor mixing ratios for all sensitivity simulations using the RIC scheme, highlighting the assumptions that yielded the lowest NME for each case. The results show that for all of the cases where sulfuric acid–organic nucleation most successfully simulated the observations (21 of 32 cases), initial SO2 mixing ratios < 30 pptv, and organic precursors < 100 pptv (with an assumed yield of 1) were needed. These SO2 mixing ratios are consistent with observations during ATom 4 (Figs. S4, S5, Table S6) and earlier results (Rollins et al., 2017, 2018). Lacking measurements of condensable organic species, we can only speculate that a few to tens of pptv are reasonable for the marine tropical UT. Williamson et al. (2019; Extended Data Fig. 7) suggested that organics dominate the composition of smaller particles at pressure < 400 hPa. We note that we performed no simulations with mixing ratios of SO2 or organics above 100 pptv. While we cannot exclude that for some cases the mixing ratios of these precursors at levels above 100 pptv could improve fits, these levels are outside of prior observations so were not considered in this study.
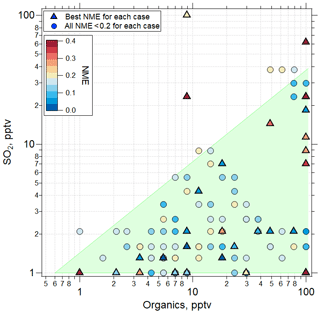
Figure 8Values of NME (colored symbols) for best fits of the sensitivity studies. TOMAS model simulations were made using the RIC sulfuric acid–organic scheme. Among the sensitivity tests using this scheme, the one with the lowest NME case is shown with a triangle located at the initial conditions of SO2 and organics for that case, while the next best NME case (provided NME < 0.2) is shown as a circle. The shaded region represents the approximate parameter space in which the best agreement between model and measurement is found for all the convective influence cases studied. Note the different NME color scale range (0–0.4) than the one presented in Fig. 6 (0–1).
In the case shown in Fig. 6, mixing ratios of SO2 and organics of ∼ 1.3 and 5.5 pptv, respectively, were sufficient to nucleate particles and produce a size distribution that matched the observations with an NME of 0.02 using the RIC scheme. In a majority of the cases, the RIC scheme predicted SO2 < 5 pptv, which is lower than typical UT SO2 concentrations, suggesting that our temperature extrapolation may overpredict nucleation rates at the typical SO2 mixing ratios of ∼ 30 pptv in the UT. Overall, the lowest NME values were obtained when initial SO2 values were low (< 30 pptv), while organics varied over a range of mixing ratios as shown by triangles in Fig. 8. This suggests that organic matter will often contribute significantly to the composition of the nucleated and growing particles on a mole basis and even more so on a mass basis, because the assumed molecular weight of organic precursors and products is 200 g mol−1 compared to 96 g mol−1 for SO4.
3.3 Discussion
Comparing aerosol size distribution measurements with box model simulations shows that none of the binary neutral or ion-assisted NPF schemes are consistent with observations, regardless of precursor concentrations and the presence or absence of condensing organics for further growth. These schemes predict significant nucleation but do not make enough particles in the 5–20 nm size range (Fig. 6) to match observations. Adding organics for initial growth of particles shifts the size distribution to larger sizes but only slightly improves the model-to-measurement fits (Table S4).
However, schemes that incorporate organic compounds or NH3 to nucleate particles, plus condensing organics as growth agents, can plausibly replicate the observed size distributions. These results suggest that organic precursor species are likely important in NPF and initial growth in the tropical upper troposphere, even above marine regions remote from continental sources. In general, the RIC scheme provided best model-to-measurement fits; however, the improvement in the fit values for the DUN scheme when organics are added for initial growth of particles suggests that organics may be more important for growth than for nucleation (Table S4).
We find that to best reproduce both nucleation and growth rates by the RIC scheme, the mixing ratios of gas-phase organic precursors generally needs to be at least twice that of SO2 (Fig. 8). While an example in Fig. 6 shows that the source of condensable organics may be even ∼ 5 times the SO2 mixing ratio in the remote tropical UT (Fig. S66), we do not know whether or not there may be that much more organic precursor available in this region. Although, regions where the oceanic source of SOA may be higher than the DMS source have been reported previously (e.g., Croft et al., 2019).
Unfortunately, we have no information on the nature and mixing ratios of oxidized organic species that participated in NPF and initial growth in this environment. The mixing ratios used in this study do not seem out of the range of possibility. Potential precursors to these condensing species, such as isoprene or monoterpenes (e.g., α- or β-pinene), were found to be below the limit of instrument detection (2 pptv for isoprene, 0.1 pptv for α-pinene, and 0.2 pptv for β-pinene) in the tropical UT during the ATom deployments. The exact identification of these condensing organic species would require instrumentation such as an atmospheric-pressure-interface time-of-flight (API-TOF) mass spectrometer to measure the composition of molecular clusters, which was not a part of the suite of instrumentation during the ATom mission. Other studies also suggest that NPF and growth involving organic species may be common in the remote troposphere. Willis et al. (2016) showed that marine organics contribute to the growth of newly formed particles in the summertime Arctic at low altitude; however, it was unclear if marine organics were involved in nucleation. Burkart et al. (2017) found that particle growth in the remote Arctic was largely due to condensation of unidentified organic compounds, possibly of marine origin, associated with oxidation or photochemistry of the sea-surface micro-layer (Abbatt et al., 2019). Andreae et al. (2018) proposed that oxidized biogenic volatile organic compounds (VOCs) were the source of recently formed particles found in the outflows and anvils of convective storms over Amazonia.
Chemistry–climate models rarely include organic-driven nucleation pathways in the UT where globally significant NPF takes place. This may result in poor estimates of NPF and CCN abundance and contribute to uncertainties in aerosol–cloud–radiation effects. Williamson et al. (2019) showed that the production of newly formed particles and their growth to cloud-active sizes during descent towards the surface is not adequately captured in the global chemical transport models, which tend to underestimate the magnitude of tropical UT NPF and subsequent growth. This underestimate might be related to missing organic precursors, missing chemical mechanisms, or structural errors associated with convective parameterizations. According to Williamson et al. (2019), the combined direct and indirect radiative effect of NPF in the tropical UT is ∼ 0.1 W m−2 globally.
The assumptions in our box model simulations point to the need for further observational and modeling studies. For example, we do not directly simulate in TOMAS the oxidation of DMS to SO2 and MSA. However, the SO2 mixing ratios estimated in this study may serve as a proxy for DMS in the modeling in our study, although the timescale for forming H2SO4 from SO2 will be incorrect. We had measurements of SO2 only during the fourth ATom deployment, and no measurements of NH3 or highly oxygenated organic molecules that are likely aerosol precursors. Instead, we have constrained the box model simulations using reasonable lower and upper limits of their mixing ratios based on literature data and, in the case of SO2, ATom 4 data. One of the limitations of our box modeling effort is that the temperatures along the trajectories were often below the lower range limit of three (out of four) nucleation schemes evaluated (Table S6). In these cases (marked with a “∗” in Figs. S15–S45 and Table S6) the best-fit SO2 and organic concentrations are expected to be biased high. Although experimentally unverified, we incorporated temperature dependence into the Riccobono et al. (2014) scheme after Yu et al. (2017). We would expect faster nucleation rates at the lower trajectory temperatures than are simulated by these schemes (e.g., Yue and Hamill, 1979). Further, we have tested the Napari et al. (2002) scheme both with and without a tuning factor of 10−5 that was developed for continental regions (Jung et al., 2010; Westervelt et al., 2013), an obvious source of uncertainty when simulating NPF in the UT over the oceans. These are schemes that many models use and they do not appear to (often) work for this region, possibly due to their limited range of operating temperatures. We also have not explored the organic-only nucleation scheme described by Kirbky et al. (2016), an updated version of the Vehkamaki et al. (2002) scheme covering a wider range of temperatures and relative humidities by Määttänen et al. (2018) that has been validated against CLOUD measurements, or the recently published ternary nucleation look-up tables for model implementation (Yu et al., 2020), and these schemes are worth investigating in future studies.
Further, we did not account for mixing with surrounding air on the path between the cloud outflow and the point of measurement when running simulations. The box model used here simulates NPF in the outflow region of deep convective clouds. Although, active NPF was identified in the vicinity of clouds and in the cloud outflow region in many studies (such as Perry and Hobbs 1994; Clarke et al., 1998, 1999; Ström et al., 1999; Clement et al., 2002; Twohy et al., 2002; Weigelt et al., 2009; Waddicor et al., 2012; de Reus et al., 2001; Clarke and Kapustin 2002; or Andreae et al., 2018), the exact location of NPF with respect to cloud remains uncertain (Kulmala et al., 2006; Waddicor et al., 2012). We assume that nucleation does not occur within the cloud and that the outflow does not immediately mix with the surrounding air in the highly stratified upper troposphere. If NPF were to occur in a cloud or in a zone of the turbulent mixing at the cloud edge, as suggested by some studies (Ström et al., 1999; Lee et al., 2004; Weigel, 2011; Kazil et al., 2007; Kulmala et al., 2006), our results would be biased.
The limitations described above are important and point out the need to undertake further in situ measurements and modeling studies to confirm the suspected role of organics in UT NPF and subsequent growth in the remote troposphere. Better understanding of NPF in the remote UT, and the growth of these particles to cloud-active sizes, could substantially improve model simulations of the preindustrial atmosphere, would allow for better evaluations of the effect of current anthropogenic perturbations, and could allow more confident predictions of the evolution of the climate and its response to future emission scenarios. Modeling efforts should focus on developing new nucleation mechanisms based on chamber studies conducted at temperatures more representative of the UT. Further airborne research should focus on measuring the composition of molecular clusters, sulfuric acid, organics, and NH3 over the oceans and tropical continental areas. The planned Chemistry of the Atmosphere: Field Experiment in Brazil (CAFE-Brazil) study is the first expected to combine airborne measurements of nucleation-mode particle size distributions with API-TOF mass spectrometer measurements of the composition of nucleating clusters.
Airborne observations during the ATom mission show a globally significant source region of newly formed particles in the tropical and subtropical UT that persists over both the Atlantic and Pacific ocean basins over all seasons. These particles are often associated with the outflow from deep convection. Averaged across the tropics and subtropics over the Pacific, the particle number concentrations were a maximum (reaching as high as ∼ 40 000 cm−3) at altitudes above 10 km where the condensation sink from pre-existing aerosol particles was a minimum. Using backward trajectories to identify convectively influenced air parcels, the highest concentrations of recently formed particles were generally found where the CI was most recent, particularly during the first and second ATom deployments. The number concentration of nucleation-mode particles decreased with time since CI due to the effects of coagulation and condensational growth. During ATom 1 and ATom 2, particle size increased with time since CI, showing clear evidence for this growth.
We simulated particle nucleation and growth using two box models constrained to follow the calculated trajectories from the point of convective detrainment to the point of measurement by the aircraft, and we performed sensitivity tests varying the nucleation mechanisms and initial conditions such as precursor (SO2, NH3, organics), OH, and pre-existing particle concentrations.
These simulations indicate that nucleation schemes commonly used in global models, such as binary homogeneous H2SO4 (both neutral by Vehkamäki et al., 2002) or ternary H2SO4+ NH3 (neutral with and without a tuning factor by Napari et al., 2002, and Jung et al., 2010), as well as the recently developed neutral and ion-induced binary and ternary nucleation scheme by Dunne et al. (2016), were all inconsistent with observed size distributions in all simulated cases when no organics were included for growth. This result also held for the binary nucleation mechanisms even when organics were added as a condensing (but not nucleating) species. Adding organics for initial growth of particles formed by either of the tested ternary schemes (Napari et al., 2002, or Dunne et al., 2016) provided the best fits in 12 out of 32 simulated cases (Table S4). However, the majority of these ternary inorganic simulations required initial conditions of NH3 > 50 pptv, which is substantially greater than expected at these locations in the UT (Höpfner et al., 2016).
In contrast, a scheme involving oxidation products of biogenic organics and H2SO4 (Riccobono et al., 2014) gave results that were most consistent among the various models with observations in 21 out of 32 cases, while in two cases it was tied for the lowest NME with other schemes. These results strongly suggest that organics are involved in NPF and subsequent initial growth in the remote tropical UT. This supports the finding by Simon et al. (2020) that organics, despite their lower oxidation level and yield at low temperatures, may be important for nucleation and growth in the UT. However, the predicted SO2 concentrations were often anomalously low (<5 pptv), suggesting that our temperature extrapolation may overestimate the nucleation rates. While the Riccobono scheme was most consistent, the analysis suggests that multiple nucleation mechanisms may be plausible across the 32 cases.
We have assumed that the Riccobono et al. (2014) scheme, which was developed from laboratory measurements of nucleation from the oxidation products of terrestrial biogenic VOCs, represents processes in the remote marine UT of the tropical Pacific. In fact, there is virtually no information on the nature of oxidized organic species (or ammonia and amines) that may participate in NPF in this environment. Also, the Riccobono scheme, one of the least constrained nucleation mechanisms, required a large extrapolation in temperature to simulate UT conditions. Given that NPF in the tropical UT is a major source of CCN over a large portion of the globe (Williamson et al., 2019), we recommend that future work investigate the species contributing to NPF and growth explicitly, including direct measurements when possible. Additionally, we recommend studies that focus on potential tropical marine sources of aerosol precursor gases, the efficiency of their transport to the UT, the products of their oxidation, and the mechanisms of NPF at temperatures < 230 K.
The full ATom dataset is available as given in Wofsy et al. (2018, https://doi.org/10.3334/ORNLDAAC/1581) and may also be accessed at https://espoarchive.nasa.gov/archive/browse/atom (last access: April 2019). Data presented in this analysis are available at the Oak Ridge National Laboratory (ORNL) Distributed Active Archive Center (DAAC) (https://doi.org/10.3334/ORNLDAAC/1811; Kupc et al., 2021).
The supplement related to this article is available online at: https://doi.org/10.5194/acp-20-15037-2020-supplement.
AK, CJW, CAB, KDF, MD, BBW, TPB, KM, GPS, AT, and AR collected data. AK, JRP, CAB, and ALH conceived and designed the study. AK performed the analysis and wrote the article with help from CAB and JRP, with contributions from all co-authors. JRP, ALH, and JK provided TOMAS and MAIA box models and helped with model upgrades. AK performed all model simulations. MD and BBW analyzed cloud properties. ER calculated the air parcel backward trajectories.
The authors declare that they have no conflict of interest.
The contents do not necessarily represent the official views of the University of Colorado, the University of Vienna, NOAA or of the respective granting agencies. The use or mention of commercial products or services does not represent an endorsement by the authors or by any agency.
We thank Ken Aikin for contributions to this analysis, the ATom science team, and the NASA DC-8 flight crew for their contributions to the ATom data. We are grateful for the hard work of the ATom leadership and logistics teams. We thank the Whole Air Sampler (WAS; UCI) and the Trace Organic Gas Analyzer (TOGA; NCAR) teams for access to their data.
This work was supported by the National Aeronautics and Space Administration's Earth Venture program through awards NNX15AJ23G and NNH15AB12I and by NOAA's Health of the Atmosphere and Atmospheric Chemistry, Carbon Cycle, and Climate programs. Agnieszka Kupc was supported by the Austrian Science Fund FWF's Erwin Schrodinger Fellowship J-3613. Bernadett Weinzierl and Maximilian Dollner were supported by European Research Council (ERC) under the European Union's Horizon 2020 research and innovation framework program under grant 640458 (A-LIFE) and by the University of Vienna. Jeffrey R. Pierce and Anna L. Hodshire were supported by the US Department of Energy's Atmospheric System Research, an Office of Science, Office of Biological and Environmental Research program, under grant DE-SC0019000; and the NOAA, Office of Science, Office of Atmospheric Chemistry, Carbon Cycle, and Climate Program, under cooperative agreement award NA17OAR430001.
This paper was edited by Joachim Curtius and reviewed by three anonymous referees.
Abbatt, J. P. D., Leaitch, W. R., Aliabadi, A. A., Bertram, A. K., Blanchet, J.-P., Boivin-Rioux, A., Bozem, H., Burkart, J., Chang, R. Y. W., Charette, J., Chaubey, J. P., Christensen, R. J., Cirisan, A., Collins, D. B., Croft, B., Dionne, J., Evans, G. J., Fletcher, C. G., Galí, M., Ghahremaninezhad, R., Girard, E., Gong, W., Gosselin, M., Gourdal, M., Hanna, S. J., Hayashida, H., Herber, A. B., Hesaraki, S., Hoor, P., Huang, L., Hussherr, R., Irish, V. E., Keita, S. A., Kodros, J. K., Köllner, F., Kolonjari, F., Kunkel, D., Ladino, L. A., Law, K., Levasseur, M., Libois, Q., Liggio, J., Lizotte, M., Macdonald, K. M., Mahmood, R., Martin, R. V., Mason, R. H., Miller, L. A., Moravek, A., Mortenson, E., Mungall, E. L., Murphy, J. G., Namazi, M., Norman, A.-L., O'Neill, N. T., Pierce, J. R., Russell, L. M., Schneider, J., Schulz, H., Sharma, S., Si, M., Staebler, R. M., Steiner, N. S., Thomas, J. L., von Salzen, K., Wentzell, J. J. B., Willis, M. D., Wentworth, G. R., Xu, J.-W., and Yakobi-Hancock, J. D.: Overview paper: New insights into aerosol and climate in the Arctic, Atmos. Chem. Phys., 19, 2527–2560, https://doi.org/10.5194/acp-19-2527-2019, 2019.
Adams, P. J. and Seinfeld, J. H.: Predicting global aerosol size distributions in general circulation models, J. Geophys. Res., 107, AAC 4-1–AAC 4-23, https://doi.org/10.1029/2001jd001010, 2002.
Adams, P. J., Seinfeld, J. H., and Koch, D. M.: Global concentrations of tropospheric sulfate, nitrate, and ammonium aerosol simulated in a general circulation model, J. Geophys. Res., 104, 13791–13823, https://doi.org/10.1029/1999jd900083, 1999.
Almeida, J., Schobesberger, S., Kürten, A., Ortega, I. K., Kupiainen-Määttä, O., Praplan, A. P., Adamov, A., Amorim, A., Bianchi, F., Breitenlechner, M., David, A., Dommen, J., Donahue, N. M., Downard, A., Dunne, E., Duplissy, J., Ehrhart, S., Flagan, R. C., Franchin, A., Guida, R., Hakala, J., Hansel, A., Heinritzi, M., Henschel, H., Jokinen, T., Junninen, H., Kajos, M., Kangasluoma, J., Keskinen, H., Kupc, A., Kurtén, T., Kvashin, A. N., Laaksonen, A., Lehtipalo, K., Leiminger, M., Leppä, J., Loukonen, V., Makhmutov, V., Mathot, S., McGrath, M. J., Nieminen, T., Olenius, T., Onnela, A., Petäjä, T., Riccobono, F., Riipinen, I., Rissanen, M., Rondo, L., Ruuskanen, T., Santos, F. D., Sarnela, N., Schallhart, S., Schnitzhofer, R., Seinfeld, J. H., Simon, M., Sipilä, M., Stozhkov, Y., Stratmann, F., Tomé, A., Tröstl, J., Tsagkogeorgas, G., Vaattovaara, P., Viisanen, Y., Virtanen, A., Vrtala, A., Wagner, P. E., Weingartner, E., Wex, H., Williamson, C., Wimmer, D., Ye, P., Yli-Juuti, T., Carslaw, K. S., Kulmala, M., Curtius, J., Baltensperger, U., Worsnop, D. R., Vehkamäki, H., and Kirkby, J.: Molecular understanding of sulphuric acid–amine particle nucleation in the atmosphere, Nature, 502, 359–363, https://doi.org/10.1038/nature12663, 2013.
Andreae, M. O., Afchine, A., Albrecht, R., Holanda, B. A., Artaxo, P., Barbosa, H. M. J., Borrmann, S., Cecchini, M. A., Costa, A., Dollner, M., Fütterer, D., Järvinen, E., Jurkat, T., Klimach, T., Konemann, T., Knote, C., Krämer, M., Krisna, T., Machado, L. A. T., Mertes, S., Minikin, A., Pöhlker, C., Pöhlker, M. L., Pöschl, U., Rosenfeld, D., Sauer, D., Schlager, H., Schnaiter, M., Schneider, J., Schulz, C., Spanu, A., Sperling, V. B., Voigt, C., Walser, A., Wang, J., Weinzierl, B., Wendisch, M., and Ziereis, H.: Aerosol characteristics and particle production in the upper troposphere over the Amazon Basin, Atmos. Chem. Phys., 18, 921–961, https://doi.org/10.5194/acp-18-921-2018, 2018.
Atkinson, R. and Arey, J.: Atmospheric degradation of volatile organic compounds, Chem. Rev., 103, 4605–4638, https://doi.org/10.1021/cr0206420, 2003.
Bianchi, F., Tröstl, J., Junninen, H., Frege, C., Henne, S., Hoyle, C. R., Molteni, U., Herrmann, E., Adamov, A., Bukowiecki, N., Chen, X., Duplissy, J., Gysel, M., Hutterli, M., Kangasluoma, J., Kontkanen, J., Kürten, A., Manninen, H. E., Münch, S., Peräkylä, O., Petäjä, T., Rondo, L., Williamson, C., Weingartner, E., Curtius, J., Worsnop, D. R., Kulmala, M., Dommen, J., and Baltensperger, U.: New particle formation in the free troposphere: A question of chemistry and timing, Science, 352, 1109–1112, https://doi.org/10.1126/science.aad5456, 2016.
Bork, N., Elm, J., Olenius, T., and Vehkamäki, H.: Methane sulfonic acid-enhanced formation of molecular clusters of sulfuric acid and dimethyl amine, Atmos. Chem. Phys., 14, 12023–12030, https://doi.org/10.5194/acp-14-12023-2014, 2014.
Borrmann, S., Kunkel, D., Weigel, R., Minikin, A., Deshler, T., Wilson, J. C., Curtius, J., Volk, C. M., Homan, C. D., Ulanovsky, A., Ravegnani, F., Viciani, S., Shur, G. N., Belyaev, G. V., Law, K. S., and Cairo, F.: Aerosols in the tropical and subtropical UT/LS: in-situ measurements of submicron particle abundance and volatility, Atmos. Chem. Phys., 10, 5573–5592, https://doi.org/10.5194/acp-10-5573-2010, 2010.
Bowman, K. P.: Large-scale isentropic mixing properties of the Antarctic polar vortex from analyzed winds, J. Geophys. Res., 98, 23013–23027, https://doi.org/10.1029/93jd02599, 1993.
Brock, C. A., Hamill, P., Wilson, J. C., Jonsson, H. H., and Chan, K. R.: Particle formation in the upper tropical troposphere: A source of nuclei for the stratospheric aerosol, Science, 270, 1650–1653, https://doi.org/10.1126/science.270.5242.1650, 1995.
Brock, C. A., Williamson, C., Kupc, A., Froyd, K. D., Erdesz, F., Wagner, N., Richardson, M., Schwarz, J. P., Gao, R.-S., Katich, J. M., Campuzano-Jost, P., Nault, B. A., Schroder, J. C., Jimenez, J. L., Weinzierl, B., Dollner, M., Bui, T., and Murphy, D. M.: Aerosol size distributions during the Atmospheric Tomography Mission (ATom): methods, uncertainties, and data products, Atmos. Meas. Tech., 12, 3081–3099, https://doi.org/10.5194/amt-12-3081-2019, 2019.
Brune, W. H., Miller, D. O., Thames, A. B., Allen, H. M., Apel, E. C., Blake, D. R., Bui, T. P., Commane, R., Crounse, J. D., Daube, B. C., Diskin, G. S., DiGangi, J. P., Elkins, J. W., Hall, S. R., Hanisco, T. F., Hannun, R. A., Hintsa, E. J., Hornbrook, R. S., Kim, M. J., McKain, K., Moore, F. L., Neuman, J. A., Nicely, J. M., Peischl, J., Ryerson, T. B., St. Clair, J. M., Sweeney, C., Teng, A. P., Thompson, C., Ullmann, K., Veres, P. R., Wennberg, P. O., and Wolfe, G. M.: Exploring oxidation in the remote free troposphere: Insights from Atmospheric Tomography (ATom), J. Geophys. Res.-Atmos., 125, e2019JD031685, https://doi.org/10.1029/2019JD031685, 2020.
Burkart, J., Hodshire, A. L., Mungall, E. L., Pierce, J. R., Collins, D. B., Ladino, L. A., Lee, A. K. Y., Irish, V., Wentzell, J. J. B., Liggio, J., Papakyriakou, T., Murphy, J., and Abbatt, J.: Organic condensation and particle growth to CCN sizes in the summertime marine Arctic is driven by materials more semivolatile than at continental sites, Geophys. Res. Lett., 44, 10725–10734, https://doi.org/10.1002/2017gl075671, 2017.
Chen, H. and Finlayson-Pitts, B. J.: New particle formation from methanesulfonic acid and amines/ammonia as a function of temperature, Environ. Sci. Technol., 51, 243–252, https://doi.org/10.1021/acs.est.6b04173, 2017.
Chen, H., Karion, A., Rella, C. W., Winderlich, J., Gerbig, C., Filges, A., Newberger, T., Sweeney, C., and Tans, P. P.: Accurate measurements of carbon monoxide in humid air using the cavity ring-down spectroscopy (CRDS) technique, Atmos. Meas. Tech., 6, 1031–1040, https://doi.org/10.5194/amt-6-1031-2013, 2013.
Chen, H., Ezell, M. J., Arquero, K. D., Varner, M. E., Dawson, M. L., Gerber, R. B., and Finlayson-Pitts, B. J.: New particle formation and growth from methanesulfonic acid, trimethylamine and water, Phys. Chem. Chem. Phys., 17, 13699–13709, https://doi.org/10.1039/c5cp00838g, 2015.
Clarke, A. D.: Atmospheric nuclei in the remote free-troposphere, J. Atmos. Chem., 14, 479–488, https://doi.org/10.1007/BF00115252, 1992.
Clarke, A. D.: Atmospheric nuclei in the Pacific midtroposphere: Their nature, concentration, and evolution, J. Geophys. Res., 98, 20633–20647, https://doi.org/10.1029/93jd00797, 1993.
Clarke, A. D., Davis, D., Kapustin, V. N., Eisele, F., Chen, G., Paluch, I., Lenschow, D., Bandy, A. R., Thornton, D., Moore, K., Mauldin, L., Tanner, D., Litchy, M., Carroll, M. A., Collins, J., and Albercook, G.: Particle Nucleation in the Tropical Boundary Layer and Its Coupling to Marine Sulfur Sources, Science, 282, 89–92, https://doi.org/10.1126/science.282.5386.89, 1998.
Clarke, A. D. and Kapustin, V. N.: A Pacific Aerosol Survey. Part I: A Decade of Data on Particle Production, Transport, Evolution, and Mixing in the Troposphere, Journal of J. Atmos. Sci., 59, 363–382, https://doi.org/10.1175/1520-0469(2002)059<0363:Apaspi>2.0.Co;2, 2002.
Clarke, A. D., Eisele, F., Kapustin, V. N., Moore, K., Tanner, D., Mauldin, L., Litchy, M., Lienert, B., Carroll, M. A., and Albercook, G.: Nucleation in the equatorial free troposphere: Favorable environments during PEM-Tropics, J. Geophys. Res., 104, 5735–5744, 1999.
Clarke, A. D., Owens, S. R., and Zhou, J.: An ultrafine sea-salt flux from breaking waves: Implications for cloud condensation nuclei in the remote marine atmosphere, J. Geophys. Res., 111, D06202, https://doi.org/10.1029/2005JD006565, 2006.
Clement, C. F., Ford, I. J., Twohy, C. H., Weinheimer, A. J., and Campos, T.: Particle production in the outflow of a mid-latitude storm, J. Geophys. Res., 107, AAC 5-1–AAC 5-9, https://doi.org/10.1029/2001JD001352, 2002.
Croft, B., Martin, R. V., Leaitch, W. R., Burkart, J., Chang, R. Y.-W., Collins, D. B., Hayes, P. L., Hodshire, A. L., Huang, L., Kodros, J. K., Moravek, A., Mungall, E. L., Murphy, J. G., Sharma, S., Tremblay, S., Wentworth, G. R., Willis, M. D., Abbatt, J. P. D., and Pierce, J. R.: Arctic marine secondary organic aerosol contributes significantly to summertime particle size distributions in the Canadian Arctic Archipelago, Atmos. Chem. Phys., 19, 2787–2812, https://doi.org/10.5194/acp-19-2787-2019, 2019.
de Reus, M., Krejci, R., Scheele, R., Williams, J., Fischer, H., and Ström, J.: Vertical distributions of the aerosol number concentration and size distribution over the northern hemisphere Indian Ocean, J. Geophys. Res., 106, 28629–28642, 2001.
Dollner, M., et al.: Global in-situ observations of cloud phase during the airborne Atmospheric Tomography Mission, Atmos. Chem. Phys. Discuss., in preparation, 2021.
Dunne, E. M., Gordon, H., Kürten, A., Almeida, J., Duplissy, J., Williamson, C., Ortega, I. K., Pringle, K. J., Adamov, A., Baltensperger, U., Barmet, P., Benduhn, F., Bianchi, F., Breitenlechner, M., Clarke, A., Curtius, J., Dommen, J., Donahue, N. M., Ehrhart, S., Flagan, R. C., Franchin, A., Guida, R., Hakala, J., Hansel, A., Heinritzi, M., Jokinen, T., Kangasluoma, J., Kirkby, J., Kulmala, M., Kupc, A., Lawler, M. J., Lehtipalo, K., Makhmutov, V., Mann, G., Mathot, S., Merikanto, J., Miettinen, P., Nenes, A., Onnela, A., Rap, A., Reddington, C. L. S., Riccobono, F., Richards, N. A. D., Rissanen, M. P., Rondo, L., Sarnela, N., Schobesberger, S., Sengupta, K., Simon, M., Sipilä, M., Smith, J. N., Stozkhov, Y., Tomé, A., Tröstl, J., Wagner, P. E., Wimmer, D., Winkler, P. M., Worsnop, D. R., and Carslaw, K. S.: Global atmospheric particle formation from CERN CLOUD measurements, Science, 354, 1119–1124, https://doi.org/10.1126/science.aaf2649, 2016.
English, J. M., Toon, O. B., Mills, M. J., and Yu, F.: Microphysical simulations of new particle formation in the upper troposphere and lower stratosphere, Atmos. Chem. Phys., 11, 9303–9322, https://doi.org/10.5194/acp-11-9303-2011, 2011.
Faloona, I. C., Tan, D., Lesher, R. L., Hazen, N. L., Frame, C. L., Simpas, J. B., Harder, H., Martinez, M., Di Carlo, P., Ren, X., and Brune, W. H.: A laser-induced fluorescence instrument for detecting tropospheric OH and HO2: Characteristics and calibration, J. Atmos. Chem., 47, 139–167, https://doi.org/10.1023/B:JOCH.0000021036.53185.0e, 2004.
Feng, Y. and Penner, J. E.: Global modeling of nitrate and ammonium: Interaction of aerosols and tropospheric chemistry, J. Geophys. Res., 112, D01304, https://doi.org/10.1029/2005jd006404, 2007.
Froyd, K. D., Murphy, D. M., Sanford, T. J., Thomson, D. S., Wilson, J. C., Pfister, L., and Lait, L.: Aerosol composition of the tropical upper troposphere, Atmos. Chem. Phys., 9, 4363–4385, https://doi.org/10.5194/acp-9-4363-2009, 2009.
Froyd, K. D., Murphy, D. M., Brock, C. A., Campuzano-Jost, P., Dibb, J. E., Jimenez, J.-L., Kupc, A., Middlebrook, A. M., Schill, G. P., Thornhill, K. L., Williamson, C. J., Wilson, J. C., and Ziemba, L. D.: A new method to quantify mineral dust and other aerosol species from aircraft platforms using single-particle mass spectrometry, Atmos. Meas. Tech., 12, 6209–6239, https://doi.org/10.5194/amt-12-6209-2019, 2019.
Gordon, H., Kirkby, J., Baltensperger, U., Bianchi, F., Breitenlechner, M., Curtius, J., Dias, A., Dommen, J., Donahue, N. M., Dunne, E. M., Duplissy, J., Ehrhart, S., Flagan, R. C., Frege, C., Fuchs, C., Hansel, A., Hoyle, C. R., Kulmala, M., Kürten, A., Lehtipalo, K., Makhmutov, V., Molteni, U., Rissanen, M. P., Stozkhov, Y., Tröstl, J., Tsagkogeorgas, G., Wagner, R., Williamson, C., Wimmer, D., Winkler, P. M., Yan, C., and Carslaw, K. S.: Causes and importance of new particle formation in the present-day and preindustrial atmospheres, J. Geophys. Res., 122, 8739–8760, https://doi.org/10.1002/2017jd026844, 2017.
Hodshire, A. L., Palm, B. B., Alexander, M. L., Bian, Q., Campuzano-Jost, P., Cross, E. S., Day, D. A., de Sá, S. S., Guenther, A. B., Hansel, A., Hunter, J. F., Jud, W., Karl, T., Kim, S., Kroll, J. H., Park, J.-H., Peng, Z., Seco, R., Smith, J. N., Jimenez, J. L., and Pierce, J. R.: Constraining nucleation, condensation, and chemistry in oxidation flow reactors using size-distribution measurements and aerosol microphysical modeling, Atmos. Chem. Phys., 18, 12433–12460, https://doi.org/10.5194/acp-18-12433-2018, 2018.
Hodshire, A. L., Bian, Q., Ramnarine, E., Lonsdale, C. R., Alvarado, M. J., Kreidenweis, S. M., Jathar, S. H., and Pierce, J. R.: More Than Emissions and Chemistry: Fire Size, Dilution, and Background Aerosol Also Greatly Influence Near-Field Biomass Burning Aerosol Aging, J. Geophys. Res.-Atmos., 124, 5589–5611, https://doi.org/10.1029/2018JD029674, 2019.
Höpfner, M., Volkamer, R., Grabowski, U., Grutter, M., Orphal, J., Stiller, G., von Clarmann, T., and Wetzel, G.: First detection of ammonia (NH3) in the Asian summer monsoon upper troposphere, Atmos. Chem. Phys., 16, 14357–14369, https://doi.org/10.5194/acp-16-14357-2016, 2016.
IPCC: Climate Change 2013: The Physical Science Basis. Contribution of Working Group I to the Fifth Assessment Report of the Intergovernmental Panel on Climate Change, Cambridge University Press, Cambridge, United Kingdom and New York, NY, USA, 1535 pp., 2013.
Jen, C. N., Zhao, J., McMurry, P. H., and Hanson, D. R.: Chemical ionization of clusters formed from sulfuric acid and dimethylamine or diamines, Atmos. Chem. Phys., 16, 12513–12529, https://doi.org/10.5194/acp-16-12513-2016, 2016.
Jung, J., Fountoukis, C., Adams, P. J., and Pandis, S. N.: Simulation of in situ ultrafine particle formation in the eastern United States using PMCAMx-UF, J. Geophys. Res., 115, D03203, https://doi.org/10.1029/2009jd012313, 2010.
Kazil, J. and Lovejoy, E. R.: A semi-analytical method for calculating rates of new sulfate aerosol formation from the gas phase, Atmos. Chem. Phys., 7, 3447–3459, https://doi.org/10.5194/acp-7-3447-2007, 2007.
Kazil, J., Stier, P., Zhang, K., Quaas, J., Kinne, S., O'Donnell, D., Rast, S., Esch, M., Ferrachat, S., Lohmann, U., and Feichter, J.: Aerosol nucleation and its role for clouds and Earth's radiative forcing in the aerosol-climate model ECHAM5-HAM, Atmos. Chem. Phys., 10, 10733–10752, https://doi.org/10.5194/acp-10-10733-2010, 2010.
Kerminen, V.-M., Chen, X., Vakkari, V., Petäjä, T., Kulmala, M., and Bianchi, F.: Atmospheric new particle formation and growth: review of field observations, Environ. Res. Lett., 13, 103003, https://doi.org/10.1088/1748-9326/aadf3c, 2018.
Kirkby, J., Curtius, J., Almeida, J., Dunne, E., Duplissy, J., Ehrhart, S., Franchin, A., Gagné, S., Ickes, L., Kürten, A., Kupc, A., Metzger, A., Riccobono, F., Rondo, L., Schobesberger, S., Tsagkogeorgas, G., Wimmer, D., Amorim, A., Bianchi, F., Breitenlechner, M., David, A., Dommen, J., Downard, A., Ehn, M., Flagan, R. C., Haider, S., Hansel, A., Hauser, D., Jud, W., Junninen, H., Kreissl, F., Kvashin, A., Laaksonen, A., Lehtipalo, K., Lima, J., Lovejoy, E. R., Makhmutov, V., Mathot, S., Mikkilä, J., Minginette, P., Mogo, S., Nieminen, T., Onnela, A., Pereira, P., Petäjä, T., Schnitzhofer, R., Seinfeld, J. H., Sipilä, M., Stozhkov, Y., Stratmann, F., Tomé, A., Vanhanen, J., Viisanen, Y., Vrtala, A., Wagner, P. E., Walther, H., Weingartner, E., Wex, H., Winkler, P. M., Carslaw, K. S., Worsnop, D. R., Baltensperger, U., and Kulmala, M.: Role of sulphuric acid, ammonia and galactic cosmic rays in atmospheric aerosol nucleation, Nature, 476, 429–433, https://doi.org/10.1038/nature10343, 2011.
Kirkby, J., Duplissy, J., Sengupta, K., Frege, C., Gordon, H., Williamson, C., Heinritzi, M., Simon, M., Yan, C., Almeida, J., Tröstl, J., Nieminen, T., Ortega, I. K., Wagner, R., Adamov, A., Amorim, A., Bernhammer, A.-K., Bianchi, F., Breitenlechner, M., Brilke, S., Chen, X., Craven, J., Dias, A., Ehrhart, S., Flagan, R. C., Franchin, A., Fuchs, C., Guida, R., Hakala, J., Hoyle, C. R., Jokinen, T., Junninen, H., Kangasluoma, J., Kim, J., Krapf, M., Kürten, A., Laaksonen, A., Lehtipalo, K., Makhmutov, V., Mathot, S., Molteni, U., Onnela, A., Peräkylä, O., Piel, F., Petäjä, T., Praplan, A. P., Pringle, K., Rap, A., Richards, N. A. D., Riipinen, I., Rissanen, M. P., Rondo, L., Sarnela, N., Schobesberger, S., Scott, C. E., Seinfeld, J. H., Sipilä, M., Steiner, G., Stozhkov, Y., Stratmann, F., Tomé, A., Virtanen, A., Vogel, A. L., Wagner, A. C., Wagner, P. E., Weingartner, E., Wimmer, D., Winkler, P. M., Ye, P., Zhang, X., Hansel, A., Dommen, J., Donahue, N. M., Worsnop, D. R., Baltensperger, U., Kulmala, M., Carslaw, K. S., and Curtius, J.: Ion-induced nucleation of pure biogenic particles, Nature, 533, 521–526, https://doi.org/10.1038/nature17953, 2016.
Kulmala, M., Lehtinen, K. E. J., and Laaksonen, A.: Cluster activation theory as an explanation of the linear dependence between formation rate of 3 nm particles and sulphuric acid concentration, Atmos. Chem. Phys., 6, 787–793, https://doi.org/10.5194/acp-6-787-2006, 2006.
Kulmala, M., Kontkanen, J., Junninen, H., Lehtipalo, K., Manninen, H. E., Nieminen, T., Petäjä, T., Sipilä, M., Schobesberger, S., Rantala, P., Franchin, A., Jokinen, T., Järvinen, E., Äijälä, M., Kangasluoma, J., Hakala, J., Aalto, P. P., Paasonen, P., Mikkilä, J., Vanhanen, J., Aalto, J., Hakola, H., Makkonen, U., Ruuskanen, T., Mauldin, R. L., Duplissy, J., Vehkamäki, H., Bäck, J., Kortelainen, A., Riipinen, I., Kurtén, T., Johnston, M. V., Smith, J. N., Ehn, M., Mentel, T. F., Lehtinen, K. E. J., Laaksonen, A., Kerminen, V.-M., and Worsnop, D. R.: Direct observations of atmospheric aerosol nucleation, Science, 339, 943–946, https://doi.org/10.1126/science.1227385, 2013.
Kulmala, M., Petäjä, T., Ehn, M., Thornton, J., Sipilä, M., Worsnop, D. R., and Kerminen, V.-M.: Chemistry of Atmospheric Nucleation: On the recent advances on precursor characterization and atmospheric cluster composition in connection with atmospheric new particle formation, Annu. Rev. Phys. Chem., 65, 21–37, https://doi.org/10.1146/annurev-physchem-040412-110014, 2014.
Kupc, A., Williamson, C., Wagner, N. L., Richardson, M., and Brock, C. A.: Modification, calibration, and performance of the Ultra-High Sensitivity Aerosol Spectrometer for particle size distribution and volatility measurements during the Atmospheric Tomography Mission (ATom) airborne campaign, Atmos. Meas. Tech., 11, 369–383, https://doi.org/10.5194/amt-11-369-2018, 2018.
Kupc, A., Williamson, C. J., Hodshire, A. L., Kazil, J., Ray, E., Bui, T. P., Dollner, M., Froyd, K. D., McKain, K., Rollins, A., Schill, G. P., Thames, A., Weinzierl, B. B., Pierce, J. R., and Brock, C. A.: ATom: In Situ Tropical Aerosol Properties and Box Model Outputs, Oak Ridge, Tennessee, USA, ORNL DAAC, https://doi.org/10.3334/ORNLDAAC/1811, 2021.
Kürten, A., Bianchi, F., Almeida, J., Kupiainen-Määttä, O., Dunne, E. M., Duplissy, J., Williamson, C., Barmet, P., Breitenlechner, M., Dommen, J., Donahue, N. M., Flagan, R. C., Franchin, A., Gordon, H., Hakala, J., Hansel, A., Heinritzi, M., Ickes, L., Jokinen, T., Kangasluoma, J., Kim, J., Kirkby, J., Kupc, A., Lehtipalo, K., Leiminger, M., Makhmutov, V., Onnela, A., Ortega, I. K., Petäjä, T., Praplan, A. P., Riccobono, F., Rissanen, M. P., Rondo, L., Schnitzhofer, R., Schobesberger, S., Smith, J. N., Steiner, G., Stozhkov, Y., Tomé, A., Tröstl, J., Tsagkogeorgas, G., Wagner, P. E., Wimmer, D., Ye, P., Baltensperger, U., Carslaw, K., Kulmala, M., and Curtius, J.: Experimental particle formation rates spanning tropospheric sulfuric acid and ammonia abundances, ion production rates, and temperatures, J. Geophys. Res., 121, 12377–12400, https://doi.org/10.1002/2015jd023908, 2016.
Lee, S. H., Wilson, J. C., Baumgardner, D., Herman, R. L., Weinstock, E. M., LaFleur, B. G., Kok, G., Anderson, B., Lawson, P., Baker, B., Strawa, A., Pittman, J. V., Reeves, J. M., and Bui, T. P.: New particle formation observed in the tropical/subtropical cirrus clouds, J. Geophys. Res.-Atmos., 109, D20209, https://doi.org/10.1029/2004JD005033, 2004.
Lovejoy, E. R., Curtius, J., and Froyd, K. D.: Atmospheric ion-induced nucleation of sulfuric acid and water, J. Geophys. Res., 109, D08204, https://doi.org/10.1029/2003jd004460, 2004.
Lucas, D. D. and Akimoto, H.: Evaluating aerosol nucleation parameterizations in a global atmospheric model, Geophys. Res. Lett., 33, L10808, https://doi.org/10.1029/2006GL025672, 2006.
Määttänen, A., Merikanto, J., Henning H., Duplissy, J., Makkonen, R., Ortega, I. K., and and Vehkamäki, H.: New parameterizations for neutral and ion-induced sulfuric acid-water particle formation in nucleation and kinetic regimes, J. Geophys. Res.-Atmos., 123, 1269–1296, https://doi.org/10.1002/2017JD027429, 2018.
Merikanto, J., Napari, I., Vehkamäki, H., Anttila, T., and Kulmala, M.: New parameterization of sulfuric acid-ammonia-water ternary nucleation rates at tropospheric conditions, J. Geophys. Res., 112, D15207, https://doi.org/10.1029/2006jd007977, 2007.
Metzger, A., Verheggen, B., Dommen, J., Duplissy, J., Prevot, A. S. H., Weingartner, E., Riipinen, I., Kulmala, M., Spracklen, D. V., Carslaw, K. S., and Baltensperger, U.: Evidence for the role of organics in aerosol particle formation under atmospheric conditions, P. Natl. Acad. Sci. USA, 107, 6646–6651, https://doi.org/10.1073/pnas.0911330107, 2010.
Murphy, D. M., Cziczo, D. J., Froyd, K. D., Hudson, P. K., Matthew, B. M., Middlebrook, A. M., Peltier, R. E., Sullivan, A., Thomson, D. S., and Weber, R. J.: Single-particle mass spectrometry of tropospheric aerosol particles, J. Geophys. Res., 111, https://doi.org/10.1029/2006jd007340, 2006.
Napari, I., Noppel, M., Vehkamäki, H., and Kulmala, M.: Parametrization of ternary nucleation rates for H2SO4-NH3-H2O vapors, J. Geophys. Res., 107, AAC 6-1–AAC 6-6, https://doi.org/10.1029/2002jd002132, 2002.
NASA Langley Cloud and Radiation Research: Satellite imagery and cloud products, available at: https://www-pm.larc.nasa.gov/, last access: 1 June 2018.
National Centers for Environmental Prediction: Nation Weather Service, NOAA, U.S. Department of Commerce. NCEP GFS 0.25 degree global forecast grids historical archive. Research Data Archive at the National Center for Atmospheric Research, Computational and Information Systems Laboratory, 28 May 2018, https://doi.org/10.5065/D65D8PWK, 2015 (updated daily).
Perry, K. D. and Hobbs, P. V.: Further evidence for particle nucleation in clear air adjacent to marine cumulus clouds, J. Geophys. Res., 99, 22803–22818, https://doi.org/10.1029/94JD01926, 1994.
Pierce, J. R.: Cosmic rays, aerosols, clouds, and climate: Recent findings from the CLOUD experiment, J. Geophys. Res., 122, 8051–8055, https://doi.org/10.1002/2017jd027475, 2017.
Pierce, J. R. and Adams, P. J.: Efficiency of cloud condensation nuclei formation from ultrafine particles, Atmos. Chem. Phys., 7, 1367–1379, https://doi.org/10.5194/acp-7-1367-2007, 2007.
Pierce, J. R. and Adams, P. J.: Can cosmic rays affect cloud condensation nuclei by altering new particle formation rates?, Geophys. Res. Lett., 36, L09820, https://doi.org/10.1029/2009gl037946, 2009.
Pierce, J. R., Riipinen, I., Kulmala, M., Ehn, M., Petäjä, T., Junninen, H., Worsnop, D. R., and Donahue, N. M.: Quantification of the volatility of secondary organic compounds in ultrafine particles during nucleation events, Atmos. Chem. Phys., 11, 9019–9036, https://doi.org/10.5194/acp-11-9019-2011, 2011.
Quinn, P. K., Bates, T. S., Coffman, D. J., and Covert, D. S.: Influence of particle size and chemistry on the cloud nucleating properties of aerosols, Atmos. Chem. Phys., 8, 1029–1042, https://doi.org/10.5194/acp-8-1029-2008, 2008.
Quinn, P. K., Coffman, D. J., Johnson, J. E., Upchurch, L. M., and Bates, T. S.: Small fraction of marine cloud condensation nuclei made up of sea spray aerosol, Nat. Geosci., 10, 674, https://doi.org/10.1038/ngeo3003, 2017.
Raes, F.: Entrainment of free tropospheric aerosols as a regulating mechanism for cloud condensation nuclei in the remote marine boundary layer, J. Geophys. Res., 100, 2893–2903, https://doi.org/10.1029/94jd02832, 1995.
Raes, F., Van Dingenen, R., Cuevas, E., Van Velthoven, P. F. J., and Prospero, J. M.: Observations of aerosols in the free troposphere and marine boundary layer of the subtropical Northeast Atlantic: Discussion of processes determining their size distribution, J. Geophys. Res., 102, 21315–21328, https://doi.org/10.1029/97jd01122, 1997.
Riccobono, F., Schobesberger, S., Scott, C. E., Dommen, J., Ortega, I. K., Rondo, L., Almeida, J., Amorim, A., Bianchi, F., Breitenlechner, M., David, A., Downard, A., Dunne, E. M., Duplissy, J., Ehrhart, S., Flagan, R. C., Franchin, A., Hansel, A., Junninen, H., Kajos, M., Keskinen, H., Kupc, A., Kürten, A., Kvashin, A. N., Laaksonen, A., Lehtipalo, K., Makhmutov, V., Mathot, S., Nieminen, T., Onnela, A., Petäjä, T., Praplan, A. P., Santos, F. D., Schallhart, S., Seinfeld, J. H., Sipilä, M., Spracklen, D. V., Stozhkov, Y., Stratmann, F., Tomé, A., Tsagkogeorgas, G., Vaattovaara, P., Viisanen, Y., Vrtala, A., Wagner, P. E., Weingartner, E., Wex, H., Wimmer, D., Carslaw, K. S., Curtius, J., Donahue, N. M., Kirkby, J., Kulmala, M., Worsnop, D. R., and Baltensperger, U.: Oxidation products of biogenic emissions contribute to nucleation of atmospheric particles, Science, 344, 717–721, https://doi.org/10.1126/science.1243527, 2014.
Riipinen, I., Sihto, S.-L., Kulmala, M., Arnold, F., Dal Maso, M., Birmili, W., Saarnio, K., Teinilä, K., Kerminen, V.-M., Laaksonen, A., and Lehtinen, K. E. J.: Connections between atmospheric sulphuric acid and new particle formation during QUEST III–IV campaigns in Heidelberg and Hyytiälä, Atmos. Chem. Phys., 7, 1899–1914, https://doi.org/10.5194/acp-7-1899-2007, 2007.
Riipinen, I., Pierce, J. R., Yli-Juuti, T., Nieminen, T., Häkkinen, S., Ehn, M., Junninen, H., Lehtipalo, K., Petäjä, T., Slowik, J., Chang, R., Shantz, N. C., Abbatt, J., Leaitch, W. R., Kerminen, V.-M., Worsnop, D. R., Pandis, S. N., Donahue, N. M., and Kulmala, M.: Organic condensation: a vital link connecting aerosol formation to cloud condensation nuclei (CCN) concentrations, Atmos. Chem. Phys., 11, 3865–3878, https://doi.org/10.5194/acp-11-3865-2011, 2011.
Rollins, A. W., Thornberry, T. D., Ciciora, S. J., McLaughlin, R. J., Watts, L. A., Hanisco, T. F., Baumann, E., Giorgetta, F. R., Bui, T. V., Fahey, D. W., and Gao, R.-S.: A laser-induced fluorescence instrument for aircraft measurements of sulfur dioxide in the upper troposphere and lower stratosphere, Atmos. Meas. Tech., 9, 4601–4613, https://doi.org/10.5194/amt-9-4601-2016, 2016.
Rollins, A. W., Thornberry, T. D., Watts, L. A., Yu, P., Rosenlof, K. H., Mills, M., Baumann, E., Giorgetta, F. R., Bui, T. V., Höpfner, M., Walker, K. A., Boone, C., Bernath, P. F., Colarco, P. R., Newman, P. A., Fahey, D. W., and Gao, R. S.: The role of sulfur dioxide in stratospheric aerosol formation evaluated by using in situ measurements in the tropical lower stratosphere, Geophys. Res. Lett., 44, 4280–4286, https://doi.org/10.1002/2017gl072754, 2017.
Rollins, A. W., Thornberry, T. D., Atlas, E., Navarro, M., Schauffler, S., Moore, F., Elkins, J. W., Ray, E., Rosenlof, K., Aquila, V., and Gao, R.-S.: SO2 Observations and Sources in the Western Pacific Tropical Tropopause Region, J. Geophys. Res., 123, 13549–13559, https://doi.org/10.1029/2018jd029635, 2018.
Scott, S. G., Bui, T. P., Chan, K. R., and Bowen, S. W.: The meteorological measurement system on the NASA ER-2 aircraft, J. Atmos. Ocean. Tech., 7, 525–540, https://doi.org/10.1175/1520-0426(1990)007<0525:Tmmsot>2.0.Co;2, 1990.
Seinfeld, J. H. and Pandis, S. N.: Atmospheric Chemistry and Physics: From Air Pollution to Climate Change, Wiley, 2006.
Sihto, S.-L., Kulmala, M., Kerminen, V.-M., Dal Maso, M., Petäjä, T., Riipinen, I., Korhonen, H., Arnold, F., Janson, R., Boy, M., Laaksonen, A., and Lehtinen, K. E. J.: Atmospheric sulphuric acid and aerosol formation: implications from atmospheric measurements for nucleation and early growth mechanisms, Atmos. Chem. Phys., 6, 4079–4091, https://doi.org/10.5194/acp-6-4079-2006, 2006.
Simon, M., Dada, L., Heinritzi, M., Scholz, W., Stolzenburg, D., Fischer, L., Wagner, A. C., Kürten, A., Rörup, B., He, X.-C., Almeida, J., Baalbaki, R., Baccarini, A., Bauer, P. S., Beck, L., Bergen, A., Bianchi, F., Bräkling, S., Brilke, S., Caudillo, L., Chen, D., Chu, B., Dias, A., Draper, D. C., Duplissy, J., El-Haddad, I., Finkenzeller, H., Frege, C., Gonzalez-Carracedo, L., Gordon, H., Granzin, M., Hakala, J., Hofbauer, V., Hoyle, C. R., Kim, C., Kong, W., Lamkaddam, H., Lee, C. P., Lehtipalo, K., Leiminger, M., Mai, H., Manninen, H. E., Marie, G., Marten, R., Mentler, B., Molteni, U., Nichman, L., Nie, W., Ojdanic, A., Onnela, A., Partoll, E., Petäjä, T., Pfeifer, J., Philippov, M., Quéléver, L. L. J., Ranjithkumar, A., Rissanen, M. P., Schallhart, S., Schobesberger, S., Schuchmann, S., Shen, J., Sipilä, M., Steiner, G., Stozhkov, Y., Tauber, C., Tham, Y. J., Tomé, A. R., Vazquez-Pufleau, M., Vogel, A. L., Wagner, R., Wang, M., Wang, D. S., Wang, Y., Weber, S. K., Wu, Y., Xiao, M., Yan, C., Ye, P., Ye, Q., Zauner-Wieczorek, M., Zhou, X., Baltensperger, U., Dommen, J., Flagan, R. C., Hansel, A., Kulmala, M., Volkamer, R., Winkler, P. M., Worsnop, D. R., Donahue, N. M., Kirkby, J., and Curtius, J.: Molecular understanding of new-particle formation from α-pinene between −50 and +25 ∘C, Atmos. Chem. Phys., 20, 9183–9207, https://doi.org/10.5194/acp-20-9183-2020, 2020.
Smith, J. N., Moore, K. F., McMurry, P. H., and Eisele, F. L.: Atmospheric Measurements of Sub-20 nm Diameter Particle Chemical Composition by Thermal Desorption Chemical Ionization Mass Spectrometry, Aerosol Sci. Tech., 38, 100–110, 2004.
Stevens, B., Feingold, G., Cotton, W. R., and Walko, R. L.: Elements of the microphysical structure of numerically simulated nonprecipitating stratocumulus. J. Atmos. Sci., 53, 980–1006, https://doi.org/10.1175/1520-0469(1996)053<0980:EOTMSO>2.0.CO;2, 1996.
Ström, J., Fischer, H., Lelieveld, J., and Schröder, F.: In situ measurements of microphysical properties and trace gases in two cumulonimbus anvils over western Europe, J. Geophys. Res., 104, 12221–12226, 1999.
Thornton, D. C., Bandy, A. R., Blomquist, B. W., Talbot, R. W., and Dibb, J. E.: Transport of sulfur dioxide from the Asian Pacific Rim to the North Pacific troposphere, J. Geophys. Res., 102, 28489–28499, https://doi.org/10.1029/97jd01818, 1997.
Twohy, C. H., Clement, C. F., Gandrud, B. W., Weinheimer, A. J., Campos, T. L., Baumgardner, D., Brune, W. H., Faloona, I., Sachse, G. W., Vay, S. A., and Tan, D.: Deep convection as a source of new particles in the midlatitude upper troposphere, J. Geophys. Res., 107, AAC 6-1–AAC 6-10, https://doi.org/10.1029/2001jd000323, 2002.
Twomey, S.: Pollution and the planetary albedo, Atmos. Environ., 8, 1251–1256, https://doi.org/10.1016/0004-6981(74)90004-3, 1974.
Vehkamäki, H., Kulmala, M., Napari, I., Lehtinen, K. E. J., Timmreck, C., Noppel, M., and Laaksonen, A.: An improved parameterization for sulfuric acid–water nucleation rates for tropospheric and stratospheric conditions, J. Geophys. Res., 107, AAC 3-1–AAC 3-10, https://doi.org/10.1029/2002jd002184, 2002.
Veres, P. R., Neuman, J. A., Bertram, T. H., Assaf, E., Wolfe, G. M., Williamson, C. J., Weinzierl, B., Tilmes, S., Thompson, C. R., Thames, A. B., Schroder, J. C., Saiz-Lopez, A, Rollins, A. W., Roberts, J. M., Price, D., Peischl, J., Nault, B. A., Møller, K. H., Miller, D. O., Meinardi, S., Li, Q., Lamarque, J. F., Kupc, A., Kjaergaard, H. G., Kinnison, D., Jimenez, J. L., Jernigan, C. M, Hornbrook, R. S., Hills, A., Dollner, M., Day, D. A., Cuevas, C. A., Campuzano-Jost, P., Burkholder, J., Bui, T. P., Brune, W. H., Brown, S. S., Brock, C. A., Bourgeois, I., Blake, D. R., Apel, E. C., and Ryerson, T. B.: Global airborne sampling reveals a previously unobserved dimethyl sulfide oxidation mechanism in the marine atmosphere, P. Natl. Acad. Sci. USA, 117, 4505–4510, https://doi.org/10.1073/pnas.1919344117, 2020.
Waddicor, D. A., Vaughan, G., Choularton, T. W., Bower, K. N., Coe, H., Gallagher, M., Williams, P. I., Flynn, M., Volz-Thomas, A., Pätz, H.-W., Isaac, P., Hacker, J., Arnold, F., Schlager, H., and Whiteway, J. A.: Aerosol observations and growth rates downwind of the anvil of a deep tropical thunderstorm, Atmos. Chem. Phys., 12, 6157–6172, https://doi.org/10.5194/acp-12-6157-2012, 2012.
Weber, R., McMurry, P. H., Eisele, F., and Tanner, D.: Measurement of expected nucleation precursor species and 3–500-nm diameter particles at Mauna Loa observatory, Hawaii, J. Atmos. Sci., 2242–2257, 1995.
Weber, R. J., Marti, J. J., McMurry, P. H., Eisele, F. L., Tanner, D. J., and Jefferson, A.: Measurements of new particle formation and ultrafine particle growth rates at a clean continental site, J. Geophys. Res., 102, 4375–4385, https://doi.org/10.1029/96jd03656, 1997.
Weber, R. J., Clarke, A. D., Litchy, M., Li, J., Kok, G., Schillawski, R. D., and McMurry, P. H.: Spurious aerosol measurements when sampling from aircraft in the vicinity of clouds, J. Geophys. Res., 103, 28337–28346, https://doi.org/10.1029/98jd02086, 1998.
Weigel, R., Borrmann, S., Kazil, J., Minikin, A., Stohl, A., Wilson, J. C., Reeves, J. M., Kunkel, D., de Reus, M., Frey, W., Lovejoy, E. R., Volk, C. M., Viciani, S., D'Amato, F., Schiller, C., Peter, T., Schlager, H., Cairo, F., Law, K. S., Shur, G. N., Belyaev, G. V., and Curtius, J.: In situ observations of new particle formation in the tropical upper troposphere: the role of clouds and the nucleation mechanism, Atmos. Chem. Phys., 11, 9983–10010, https://doi.org/10.5194/acp-11-9983-2011, 2011.
Weigelt, A., Hermann, M., van Velthoven, P. F. J., Brenninkmeijer, C. A. M., Schlaf, G., Zahn, A., and Wiedensohler, A.: Influence of clouds on aerosol particle number concentrations in the upper troposphere, J. Geophys. Res., 114, D01204, https://doi.org/10.1029/2008JD009805, 2009.
Westervelt, D. M., Pierce, J. R., Riipinen, I., Trivitayanurak, W., Hamed, A., Kulmala, M., Laaksonen, A., Decesari, S., and Adams, P. J.: Formation and growth of nucleated particles into cloud condensation nuclei: model–measurement comparison, Atmos. Chem. Phys., 13, 7645–7663, https://doi.org/10.5194/acp-13-7645-2013, 2013.
Westervelt, D. M., Pierce, J. R., and Adams, P. J.: Analysis of feedbacks between nucleation rate, survival probability and cloud condensation nuclei formation, Atmos. Chem. Phys., 14, 5577–5597, https://doi.org/10.5194/acp-14-5577-2014, 2014.
Williamson, C., Kupc, A., Wilson, J., Gesler, D. W., Reeves, J. M., Erdesz, F., McLaughlin, R., and Brock, C. A.: Fast time response measurements of particle size distributions in the 3–60 nm size range with the nucleation mode aerosol size spectrometer, Atmos. Meas. Tech., 11, 3491–3509, https://doi.org/10.5194/amt-11-3491-2018, 2018.
Williamson, C. J., Kupc, A., Axisa, D., Bilsback, K. R., Bui, T., Campuzano-Jost, P., Dollner, M., Froyd, K. D., Hodshire, A. L., Jimenez, J. L., Kodros, J. K., Luo, G., Murphy, D. M., Nault, B. A., Ray, E. A., Weinzierl, B., Wilson, J. C., Yu, F., Yu, P., Pierce, J. R., and Brock, C. A.: A large source of cloud condensation nuclei from new particle formation in the tropics, Nature, 574, 399–403, https://doi.org/10.1038/s41586-019-1638-9, 2019.
Willis, M. D., Burkart, J., Thomas, J. L., Köllner, F., Schneider, J., Bozem, H., Hoor, P. M., Aliabadi, A. A., Schulz, H., Herber, A. B., Leaitch, W. R., and Abbatt, J. P. D.: Growth of nucleation mode particles in the summertime Arctic: a case study, Atmos. Chem. Phys., 16, 7663–7679, https://doi.org/10.5194/acp-16-7663-2016, 2016.
Wofsy, S. C., Afshar, S., Allen, H. M., Apel, E. C., Asher, E. C., Barletta, B., Bent, J., Bian, H., Biggs, B. C., Blake, D. R., Blake, N., Bourgeois, I., Brock, C. A., Brune, W. H., Budney, J. W., Bui, T. P., Butler, A., Campuzano-Jost, P., Chang, C. S., Chin, M., Commane, R., Correa, G., Crounse, J. D., Cullis, P. D., Daube, B. C., Day, D. A., Dean-Day, J. M., Dibb, J. E., DiGangi, J. P., Diskin, G. S., Dollner, M., Elkins, J. W., Erdesz, F., Fiore, A. M., Flynn, C. M., Froyd, K. D., Gesler, D. W., Hall, S. R., Hanisco, T. F., Hannun, R. A., Hills, A. J., Hintsa, E. J., Hoffman, A., Hornbrook, R. S., Huey, L. G., Hughes, S., Jimenez, J. L., Johnson, B. J., Katich, J. M., Keeling, R. F., Kim, M. J., Kupc, A., Lait, L. R., Lamarque, J.-F., Liu, J., McKain, K., Mclaughlin, R. J., Meinardi, S., Miller, D. O., Montzka, S. A., Moore, F. L., Morgan, E. J., Murphy, D. M., Murray, L. T., Nault, B. A., Neuman, J. A., Newman, P. A., Nicely, J. M., Pan, X., Paplawsky, W., Peischl, J., Prather, M. J., Price, D. J., Ray, E., Reeves, J. M., Richardson, M., Rollins, A. W., Rosenlof, K. H., Ryerson, T. B., Scheuer, E., Schill, G. P., Schroder, J. C., Schwarz, J. P., St.Clair, J. M., Steenrod, S. D., Stephens, B. B., Strode, S. A., Sweeney, C., Tanner, D., Teng, A. P., Thames, A. B., Thompson, C. R., Ullmann, K., Veres, P. R., Vieznor, N., Wagner, N. L., Watt, A., Weber, R., Weinzierl, B., Wennberg, P. O., Williamson, C. J., Wilson, J. C., Wolfe, G. M., Woods, C. T., and Zeng, L. H.: ATom: Merged atmospheric chemistry, trace gases, and aerosols, ORNL DAAC, Oak Ridge, Tennessee, USA, https://doi.org/10.3334/ORNLDAAC/1581, 2018.
Yu, F.: Ion-mediated nucleation in the atmosphere: Key controlling parameters, implications, and look-up table, J. Geophys. Res., 115, D03206, https://doi.org/10.1029/2009JD012630, 2010.
Yu, F., Luo, G., Bates, T. S., Anderson, B., Clarke, A., Kapustin, V., Yantosca, R. M., Wang, Y., and Wu, S.: Spatial distributions of particle number concentrations in the global troposphere: Simulations, observations, and implications for nucleation mechanisms, J. Geophys. Res., 115, D17205, https://doi.org/10.1029/2009jd013473, 2010.
Yu, F., Luo, G., Nadykto, A. B., and Herb, J.: Impact of temperature dependence on the possible contribution of organics to new particle formation in the atmosphere, Atmos. Chem. Phys., 17, 4997–5005, https://doi.org/10.5194/acp-17-4997-2017, 2017.
Yu, F., Nadykto, A. B., Luo, G., and Herb, J.: H2SO4–H2O binary and H2SO4–H2O–NH3 ternary homogeneous and ion-mediated nucleation: lookup tables version 1.0 for 3-D modeling application, Geosci. Model Dev., 13, 2663–2670, https://doi.org/10.5194/gmd-13-2663-2020, 2020.
Yue, G. K. and Hamill, P.: The homogeneous nucleation rates of H2SO4-H2O aerosol particles in air, J. Aerosol Sci., 10, 609–614, https://doi.org/10.1016/0021-8502(79)90023-5, 1979.
Zhang, Y., McMurry, P. H., Yu, F., and Jacobson, M. Z.: A comparative study of nucleation parameterizations: 1. Examination and evaluation of the formulations, J. Geophys. Res., 115, D20212, https://doi.org/10.1029/2010jd014150, 2010.
Zhu, J., Penner, J. E., Yu, F., Sillman, S., Andreae, M. O., and Coe, H.: Decrease in radiative forcing by organic aerosol nucleation, climate, and land use change, Nat. Commun., 10, 423, https://doi.org/10.1038/s41467-019-08407-7, 2019.