the Creative Commons Attribution 4.0 License.
the Creative Commons Attribution 4.0 License.
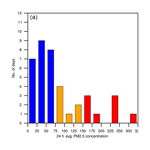
Effectiveness of short-term air quality emission controls: a high-resolution model study of Beijing during the Asia-Pacific Economic Cooperation (APEC) summit period
Tabish Umar Ansari
Jie Li
Ting Yang
Weiqi Xu
Zifa Wang
We explore the impacts of short-term emission controls on haze events in Beijing in October–November 2014 using high-resolution Weather Research and Forecasting model with Chemistry (WRF-Chem) simulations. The model reproduces surface temperature and relative humidity profiles over the period well and captures the observed variations in key atmospheric pollutants. We highlight the sensitivity of simulated pollutant levels to meteorological variables and model resolution and in particular to treatment of turbulent mixing in the planetary boundary layer. We note that simulating particle composition in the region remains a challenge, and we overpredict NH4 and NO3 at the expense of SO4. We find that the emission controls implemented for the Asia-Pacific Economic Cooperation (APEC) summit period made a relatively small contribution to improved air quality (20 %–26 %), highlighting the important role played by favourable meteorological conditions over this period. We demonstrate that the same controls applied under less favourable meteorological conditions would have been insufficient in reducing pollutant levels to meet the required standards. Continued application of these controls over the 6-week period considered would only have reduced the number of haze days when daily mean fine particulate matter exceeds 75 µg m−3 from 15 to 13 d (days). Our study highlights the limitations of current emission controls and the need for more stringent measures over a wider region during meteorologically stagnant weather.
- Article
(7278 KB) - Full-text XML
-
Supplement
(746 KB) - BibTeX
- EndNote
Air pollution poses serious health risks to urban residents and is one of the most important environmental problems facing cities around the world (Liang et al., 2017). Fine particulate matter with a diameter less than 2.5 µm (PM2.5) is a major air pollutant that often exceeds safe limits during haze episodes, which are a common occurrence in many developing megacities over the past decade. It has been estimated that outdoor air pollution, mostly by PM2.5, leads to 3.3 million premature deaths per year worldwide, predominantly in Asia (Lelieveld et al., 2015). PM2.5 also reduces visibility and has important impacts on regional climate (Westervelt et al., 2016). Beijing is the capital and political and cultural centre of China and is among the most polluted cities in the country (Batterman et al., 2016). The population of Beijing municipality increased from 14.2 million in 2002 to 21.2 million in 2013 (Ma et al., 2014), and this has been accompanied by an increase in anthropogenic emissions across the region. High PM2.5 concentrations are frequently reported in city clusters in the Beijing–Tianjin–Hebei, Yangtze River Delta, and Pearl River Delta regions in China. Haze episodes are particularly common during winter months and have attracted substantial scientific attention (Gao et al., 2017). Independent observational (J. Gao et al., 2016; Zhong et al., 2018; Shang et al., 2018; W. Chen et al., 2015, Sun et al., 2016a), modelling (Matsui et al., 2009; Kajino et al., 2017; M. Gao et al., 2015; D. Chen et al., 2016), and long-term data analysis studies (Z. Chen et al., 2016; T. Liu et al., 2017; H. Chen et al., 2015; Yan et al., 2018) have investigated the sources, evolution, and fate of PM2.5 in Beijing, but many uncertainties remain, and improved understanding is required in order to inform sound, evidence-based emission control policies. Strict short-term emission controls have been applied effectively to improve air quality in Beijing during the Beijing Olympics in 2008 (Gao et al., 2011; Yang et al., 2011) and more recently for major events such as the Asia-Pacific Economic Cooperation (APEC) summit in November 2014 (K. Li et al., 2017; Y. Wang et al., 2016) and the China Victory Day Parade in 2015 (Liang et al., 2017; Liu et al., 2016; Zhao et al., 2017). Real-world emission controls provide an ideal opportunity for testing current scientific understanding of the sources and processing of air pollution as represented in models in a robust way. With improved confidence in model performance over a focus region, we can explore the impact of alternative control options to aid formulation of more effective policies for emission reduction.
A number of previous studies investigated the effect of emission controls during the APEC period in November 2014 using surface observations (Sun et al., 2016b; Xu et al., 2015; Y. Wang et al., 2016; K. Li et al., 2017; Zhou et al., 2017) and atmospheric chemical transport models (Zhang et al., 2016; Guo et al., 2016; Wang et al., 2017; Gao et al., 2017) and found that PM2.5 concentrations were much lower than during the preceding weeks. Many of these studies attributed this improved air quality largely to the emission controls that were applied without thoroughly evaluating the role of meteorological variations. Comparison with observations in preceding weeks or over similar time periods in earlier years does not adequately account for the role of meteorology in governing haze episodes. Model studies with and without emission controls are insufficient to evaluate the contribution of meteorological processes if they focus on the control period alone without evaluating the model performance outside the control period. Gao et al. (2017) found that the emission controls reduced PM2.5 levels by about 18 µg m−3 during APEC, with about half the reduction being due to emission controls in surrounding districts outside Beijing. However, the study involved coarse-resolution (27 km) model simulations, which may be insufficient to capture regional and city-level atmospheric events well and lacked component-level analysis of aerosols. Other studies have noted the role of meteorology during the period but have not quantified it, attributing the benefits mostly to emission controls.
In this study we investigate the effectiveness of short-term emission controls and how meteorological processes influence this, using the APEC period as an example. We use a nested version of the Weather Research and Forecasting model with Chemistry (WRF-Chem) over China, with a specific focus on the Beijing–Tianjin–Hebei region. WRF-Chem has been used successfully at coarser resolution in previous studies investigating haze formation over Beijing (Matsui et al., 2009; Tie et al., 2014; Zhang et al., 2015; D. Chen et al., 2016). We describe the model set-up, emissions, and observations in Sect. 2. In Sect. 3 we present a thorough meteorological and chemical evaluation of the model simulations against surface observations and tower measurements, including aerosol composition, and we assess the strengths and weaknesses of the model. We present sensitivity studies to key physical and chemical processes in Sect. 4. In Sect. 5 we investigate the impact of emission controls over the APEC period and compare these with the same controls over a period 2 weeks earlier to demonstrate the important role of meteorological conditions in governing their effectiveness.
We use the WRF-Chem model (Grell et al., 2005; Fast et al., 2006) version 3.7.1 to simulate the meteorology and air quality over northern China. Previous studies have shown that WRF-Chem is capable of reproducing air quality in China relatively well (M. Gao et al., 2015, 2016; Guo et al., 2016; D. Chen et al., 2016). We use the Carbon Bond Mechanism version Z (CBMZ) chemistry scheme coupled with the Model for Simulating Aerosol Interactions and Chemistry (MOSAIC) aerosol module (Zaveri et al., 2008). CBMZ explicitly treats 67 species with 164 gas-phase, heterogeneous, and aqueous reactions and provides a suitable compromise between chemical complexity and computational efficiency. MOSAIC uses a sectional approach with eight aerosol size bins and treats the key aerosol species, including sulfate, nitrate, chloride, ammonium, sodium, black carbon, primary organic mass, liquid water, and other inorganic mass. Secondary organic aerosol (SOA) formation is not included in the chemical mechanism used here. Current SOA schemes are poorly parameterized for Chinese conditions and significantly underpredict SOA (M. Gao et al., 2016; Y. Gao et al., 2015). SOA contributed only 17 %–23 % of total ground-level fine particulate matter in Beijing during the period investigated here, while secondary inorganic aerosols contributed up to 62 % by mass (Sun et al., 2016b). We consider the lack of SOA formation in the model in drawing our conclusions. Further details of the model configuration used in this study are given in Table 1.
We perform two-way coupled simulations with three nested domains that include China as the parent domain (D01) at 27 km horizontal resolution, northern China as a nest (D02) at 9 km resolution, and the North China Plain as an innermost nest (D03) at 3 km resolution, as shown in Fig. 1. The model is nudged to meteorological reanalysis data above the boundary layer every 6 h for winds, temperature, and moisture to permit direct comparison of the simulations with observed pollutant concentrations under comparable conditions.
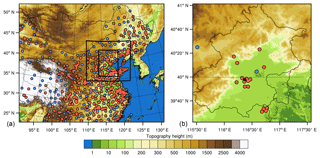
Figure 1Map of the model domain (a) showing nests over northern China and the North China Plain, and map of Beijing municipality (b) showing the location of IAP (black) and measurement stations for meteorology (blue) and air quality (red).
We use anthropogenic emissions from the Multi-resolution Emission Inventory for China (MEIC) for the year 2010 (M. Li et al., 2017). This provides emissions of major air pollutants including NOx, CO, non-methane volatile organic compounds (NMVOCs), SO2, NH3, PM2.5, PM10, black carbon (BC), and organic carbon (OC) from five major emission sectors that include residential, traffic, industry, power, and agricultural sources, and it has been used in a number of previous modelling studies (Li et al., 2015; M. Gao et al., 2015; Zhang et al., 2015; H. Chen et al., 2015; D. Chen et al., 2016). Emissions were provided at the native resolution of each domain, i.e. at 27, 9, and 3 km. We impose a vertical profile for these emissions over the lowest eight model levels to account for the effective source height distribution for each sector based on the distribution used for EMEP emissions (Bieser et al., 2011; Mailler et al., 2013) and impose a diurnal cycle for each sector. SO2 emissions over the Beijing–Tianjin–Hebei region were reduced by 50 % to account for strong emission reductions between 2010 and our focus year of 2014 (Zheng et al., 2018; Krotkov et al., 2016). We assume that 6 % by mass of SO2 is emitted as primary SO4 to account for the discrepancy between high observed concentrations of SO4 and low secondary production in the model (M. Gao et al., 2015; D. Chen et al., 2016; G. Li et al., 2017). Biogenic emissions are based on the Model of Emissions of Gases and Aerosols from Nature (MEGAN; Guenther et al., 2012). These are calculated online in the model based on canopy and emission factors and factors for leaf age, soil moisture, the leaf area index, light dependence, and temperature responses. Hourly fire emissions are included from the Fire Emissions INventory from NCAR (FINN; Wiedinmyer et al., 2011) to represent biomass burning, although this is not a major source in the region at this time of year.
To evaluate the model, meteorological observations were obtained from the National Climatic Data Center (NCDC) hourly integrated surface database (http://www.ncdc.noaa.gov/data-access/, last access: 3 July 2019) for all of China. These sites are shown in Fig. 1. We focus on 2 m temperature and relative humidity, 10 m wind speed, and direction for model evaluation. Vertical profiles of meteorological variables were obtained from the 325 m high observational tower located at the Institute of Atmospheric Physics (IAP), Chinese Academy of Sciences, Beijing ( N, E). This provides independent measurements of temperature, relative humidity, wind speed, and wind direction at 17 different height levels. Measurements of boundary layer mixing height were retrieved from aerosol lidar profiles at IAP (Yang et al., 2017), providing a valuable additional test of model meteorological processes. Hourly concentrations of NO2, CO, SO2, O3, PM2.5, and PM10 are available from the national monitoring network run by the China National Environmental Monitoring Center (CNEMC). In addition, detailed measurements of atmospheric pollutants and aerosol composition were made from IAP tower over the October–November 2014 period. These include measurements of NH4, NO3, SO4, and OC from an Aerodyne Aerosol Chemical Speciation Monitor (ACSM) instrument at 260 m altitude (Sun et al., 2016b) and from a high-resolution aerosol mass spectrometer (HR-AMS) instrument at the surface (Xu et al., 2015), and BC at the surface was measured with an aethalometer. The size-segregated samples collected at the two heights were analysed for water-soluble ions. Detailed procedures for the data analysis are described in Ng et al. (2011) and Sun et al. (2012).
To investigate the strengths and weaknesses of the model in representing air quality in China, the model was evaluated against meteorological and pollutant measurements across all three domains and at IAP tower site in Beijing.
3.1 Meteorology
We test the model performance using two sets of meteorological fields: final reanalysis data (FNL) from the National Centers for Environmental Prediction (NCEP) and ERA-Interim data from the European Centre for Medium-Range Weather Forecasts (ECMWF). Table 2 presents a comparison of the performance of the model against ground-based observations from the NCDC dataset for Beijing and the North China Plain. For a detailed evaluation over each model domain, please see Table S1 in the Supplement. The average 2 m temperature is reproduced well over the North China Plain but is overpredicted at the single Beijing site. This is located at the airport on the outskirts of the city and may not be representative of the wider region. The surface relative humidity is underpredicted for all domains with both sets of fields, although the biases are smaller and correlation is better with ECMWF data. The humidity is underpredicted by about 15 % at the Beijing site, and this may have implications for heterogeneous reactions and the hydroscopic growth of secondary aerosols. The 10 m wind speed is substantially underpredicted using both sets of fields, and this is most notable for the Beijing site. However, the correlation at this site is reasonably good, suggesting that the hourly variability in wind speeds is captured adequately. The 10 m wind direction and its variability are also reproduced relatively well. Based on these comparisons, and on subsequent comparison of pollutant concentrations, we find that the model performs marginally better using the ECMWF meteorological fields. With these fields the model captures the timing of pollution episodes better, leading to more realistic pollutant behaviour, and we have therefore chosen to use ECMWF fields for our model studies.
Table 2Comparison of observed and simulated meteorological variables using FNL and ECMWF fields.
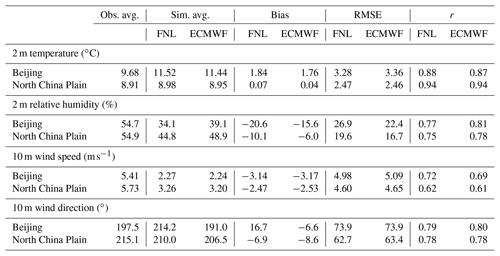
Hourly values are taken from 1 station in Beijing and 30 stations over the North China Plain from 12 October to 19 November 2014. Where observation data are missing, model values were removed to ensure that sampling was consistent.
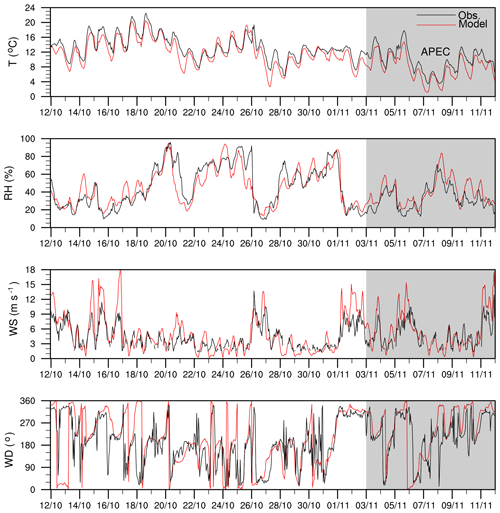
Figure 2Comparison of meteorological measurements at 190–310 m on IAP tower in Beijing with model simulations using ECMWF meteorological fields between 12 October and 12 November 2014. The period with emission controls is shaded.
Figure 2 presents an evaluation of meteorological variables with measurements from IAP tower. We evaluate the model against measurements at 190–310 m (model level 4) to minimize the effects of buildings surrounding the site, which are not adequately resolved in the model. The daily maxima and minima in temperature are reproduced reasonably well, with a small underestimation that averages less than 2 ∘C. The diurnal variations and averages for relative humidity, wind speed, and wind direction are also captured well. The mean bias in relative humidity is 0.9 %, and the large underprediction seen at the airport meteorological station is not evident here, suggesting that it may be a surface-level feature or reflect overestimation of temperature at that location. Over the height of the tower (five model levels; Fig. S2) the diurnal variation in humidity drops by more than a factor of 2, very similar to the reduction seen in the observations. The wind speed is slightly overestimated during windier periods, with a mean bias of 0.54 m s−1. This suggests that the underestimation of 10 m wind speeds at meteorological stations seen in Table 2 is a surface feature in the model and does not represent a systematic bias throughout the boundary layer. The synoptic patterns in all four variables are captured very well, highlighting the quality of the ECMWF meteorological data, and there is only one occasion, on 20–21 October, when substantial deviations in temperature and humidity are evident. There are some marked differences in meteorological conditions between the APEC period (3–12 November) and the period preceding it. These include a gradual temperature drop of 7 ∘C associated with the changing seasons, which is accompanied by a drop in relative humidity. There is an increase in the frequency of northwesterly flow with higher wind speeds, and this contrasts strongly with the lighter wind speeds and more frequent southerly flow in October. These changes are captured well by the model. A more detailed comparison of the meteorological conditions is given in Table S2 in the Supplement.
3.2 Air quality
We ran the model from 10 October to 19 November 2014 using ECMWF meteorology, and the first 2 d (days) were set aside as model spin-up. A comparison of hourly modelled pollutants for Beijing and the North China Plain against measurements from the CNEMC network is presented for October in Table 3, and the mean spatial distribution of PM2.5 during October is shown in Fig. 3. We do not include the November period here because emission controls were implemented across Beijing and surrounding provinces from the beginning of November. A more detailed comparison of concentrations on an hourly and daily basis over all model domains is given in Table S3 in the Supplement.
Table 3Comparison of hourly pollutant concentrations with network measurements over the period 12–31 October 2014.
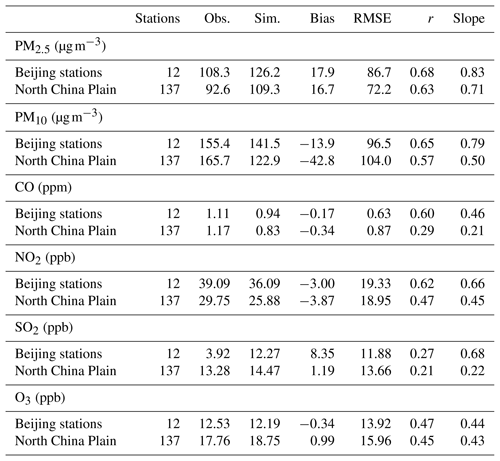
Where observation data are missing, model values were removed to ensure consistent sampling.
The model overpredicts average surface PM2.5 over the period by 5 %–18 % across the three model domains. The correlation for hourly PM2.5 improves with resolution, from r=0.47 for domain 1 to 0.63 for domain 3 and 0.68 for the 12 Beijing sites. The model underestimates PM10, although the biases are relatively small (<10 %) over Beijing. This may be attributed to the lack of mineral dust sources in the model, which play a relatively small role over Beijing at this time of year. CO is underestimated over much of China, suggesting that the emissions in the inventory are too low, but the biases are relatively small over Beijing and the variability is captured well. A similar effect is seen for NO2, which is underestimated by as much as 45 % over parts of China but by only 8 % over the Beijing sites. This may partly reflect better representation of the emission distribution for this shorter-lived pollutant on a finer grid. SO2 is underestimated by 13 % over most of China but is overestimated over Beijing by a factor of 3. The large overestimation for Beijing can be attributed to the recent rapid reduction in emissions in the region between 2010 and 2014 that are not represented in the 2010 inventory (Zheng et al., 2018). Ozone is reproduced well over Beijing but is overestimated over much of China; this may reflect the bias in NO2 concentrations and is likely to be influenced by the urban locations of most of the air quality stations.
For most pollutants, the correlation and slope improve substantially with resolution and are better on a daily mean basis than at hourly resolution. This suggests that the day-to-day variability driven largely by regional meteorological processes is captured better than the diurnal variations driven by chemistry and local boundary layer mixing, as expected. This is particularly noticeable for ozone, although concentrations of this pollutant remain low at this time of year. Daily mean concentrations are typically used for most metrics of pollutant impacts on human health, and the reasonable model performance for daily averaged data suggests that it is suitable for assessment of these policy-relevant metrics.
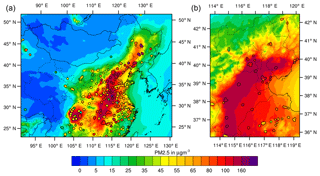
Figure 3Average spatial distribution of PM2.5 over the period 12–31 October 2014 for model domain 1 (China; a) and domain 3 (North China Plain; b) along with observations shown in circles.
The spatial distribution of mean PM2.5 concentrations over 12–31 October is shown in Fig. 3. The distribution is captured reasonably well by the model, with the western parts of China showing clean air (with concentrations less than 10 µg m−3), while the eastern, more populous parts of the country show average concentrations of 70–150 µg m−3. Key hotspots over the North China Plain, central China, and the Sichuan Basin are reproduced, and concentrations in coastal regions are notably lower, matching observations. The North China Plain is one of the most densely populated parts of the country, incorporating major cities such as Beijing, Tianjin, and Shijiazhuang, and frequently experiences heavy haze episodes with high levels of particulate matter (Wang et al., 2014; M. Gao et al., 2015). The highest concentrations of PM2.5 occur on the western side of the North China Plain, where they are trapped by southeasterly winds against the Taihang Mountains, and this is reproduced well by the model. There is a notable east–west gradient as concentrations drop off eastwards towards the coast. Over the mountains to the northwest of Beijing, concentrations are much lower, being typically less than 40 µg m−3.
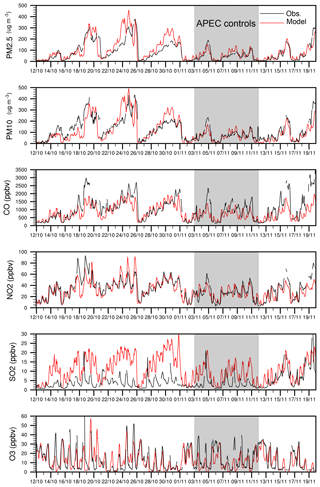
Figure 4Mean time series of surface pollutants over the 12 air quality stations in Beijing. Model values are with baseline emissions at all times, including during the APEC period (shaded).
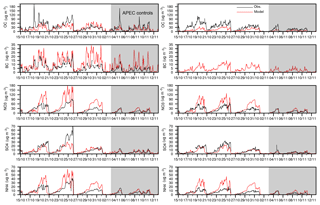
Figure 5Measured and simulated aerosol components at the surface (left) and 260 m (right) on IAP tower in Beijing. Model values are with baseline emissions at all times, including during the APEC period (shaded).
Figure 4 shows the time series of key gas-phase and particulate pollutants averaged over the 12 network sites in Beijing. The general synoptic and diurnal patterns of PM2.5, PM10, CO, NO2, and O3 are reproduced well by the model, including the magnitude of daily maxima and minima. SO2 is greatly overestimated in October, reflecting recent rapid emission reductions in Beijing (Zheng et al., 2018), and this is consistent with the findings of previous studies (D. Chen et al., 2016; M. Gao et al., 2015; Guo et al., 2016). However, we note that SO2 is reproduced much better from 15 November onwards following the start of the heating season, highlighting the continuing major importance of this source. The observations show that the region experiences clear synoptic patterns of pollutant build-up over 4–5 d followed by a sudden clean-out which is typically associated with frontal passage from the northwest (Guo et al., 2014). These synoptic patterns are seen more clearly for particulate matter than for gas-phase pollutants like NO2 and CO, which exhibit a stronger diurnal signal reflecting chemical and dynamical processes. With the exception of SO2, key pollutants and their variation over this period are reproduced well.
Comparison of aerosol composition with measurements at IAP over this period provides a more critical test of model performance (see Fig. 5). The model overestimates BC, NO3, and NH4 and underpredicts OC and SO4 during the three episodes in October. Overestimation of BC likely reflects the reduction in emissions between 2010 and 2014 but may also indicate insufficient removal in the model. The overprediction of NO3 and NH4 may be due to uncertainty in NO2 and NH3 emissions or to overestimated gas to particle conversion in the model. In particular, secondary production of NO3 and NH4 may be overestimated during stagnant conditions during pollution episodes but matches better in November, when conditions are less stagnant. The underestimation of SO4 occurs despite an overestimation of gas-phase SO2, highlighting insufficient formation of SO4 in the model. This may also contribute to the overestimation of NO3, as a decrease in SO4 frees up ammonia to react with nitric acid and transfers it into the aerosol phase (Seinfeld and Pandis, 2006). The underestimation of OC can be explained by the absence of secondary organic aerosol in our studies.
The model captures the vertical gradients of NO3 and SO4 well, with drops of 10 %–15 % and 30 % between the surface and 260 m, respectively, similar to the drops seen in the observations. However, the model shows a weaker vertical gradient than that observed (22 % vs. 33 % drop) for NH4, which can be attributed to higher secondary production of NH4 in the model. For OC, the model shows a stronger vertical gradient than that observed (53 % vs. 12 % drop), which reflects the lack of secondary production at elevated levels in the model.
While the baseline model simulation with ECMWF meteorological fields reproduces observed pollutant levels reasonably well, the comparisons have highlighted uncertainties associated with resolution, vertical mixing processes, and aerosol composition. We explore the sensitivity of our results to these factors here.
4.1 Model resolution
To investigate the benefits of high spatial resolution, we sample all three model domains at the 12 Beijing stations and compare the results with observations. To eliminate the influence of two-way nesting, where results from nested domains feed back to the parent domain, we perform an additional simulation at 27 km resolution over the parent domain only. Table 4 shows a comparison of modelled PM2.5 over Beijing for the different resolutions with measurements in October. In the nested simulation, PM2.5 is overestimated by 14 % for domain 1, 19 % for domain 2, and 16 % for domain 3 but is underestimated by 8 % for the domain 1 simulation without nesting. Although the mean biases do not improve with higher resolution, reflecting the two-way nesting, there is a substantial improvement in the correlation coefficient (0.59 to 0.68) and slope (0.55 to 0.83) for PM2.5 when nesting is used, and this occurs for other pollutants. too (see Table S4). For many variables the results sampled at 9 km resolution (D02) are slightly better than those sampled at 3 km resolution (D03). Results at 27 km resolution without nesting are substantially less good than those with two-way nesting, highlighting the important contribution of the coupling. We conclude that it is worth performing simulations at higher horizontal resolution, as it gives a better representation of urban pollution levels.
4.2 Boundary layer mixing
Representing turbulent mixing processes in the boundary layer well is critical for simulating surface air quality. The nighttime boundary layer under stable meteorological conditions is particularly difficult to model, and we find that the mixing height is often severely underpredicted (as low as 20 m on some occasions), causing pollutant concentrations to reach unrealistically high levels. Nudging meteorological fields to ECMWF reanalysis data reduces this bias but does not remove it. After testing a number of different boundary layer algorithms, we selected the Yonsei University (YSU) scheme (Hong et al., 2006), as it provides the best overall match to lidar-derived observations of boundary layer height. However, stable conditions remain a challenge for this scheme, and we therefore explore the sensitivity of simulated surface concentrations to boundary layer mixing under these conditions.
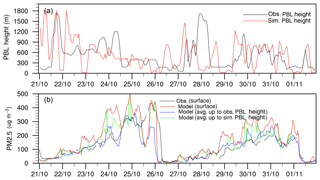
Figure 6Simulated and observed boundary layer mixing height in metres (a) and simulated and observed PM2.5 (in µg m−3) showing the effect of mixing up to the PBL height in the model (b) between 21 October and 1 November 2014.
Figure 6 shows the time series of simulated and observed planetary boundary layer (PBL) height. The observed PBL height was derived from lidar extinction profiles at IAP using the cubic root gradient method of Yang et al. (2017). The simulated PBL height was diagnosed using the maximum decrease in the modelled PM2.5 profile to ensure a consistent definition. We compare the observed PBL height with the simulated height at IAP and use PM2.5 measurements from the surface pollutant station at Aotizhongxin, the closest station to IAP site (within 2 km), to assess the effect on PM2.5 concentrations. The PBL height shows highly variable behaviour over the day and from day to day. While the average model PBL height (514 m) is similar to the average observed height (509 m) over the haze episodes shown, the nighttime PBL height is severely underpredicted on a number of occasions. Assuming that the PBL height reflects the efficiency of mixing in the boundary layer, we expect the model to overpredict surface pollutant concentrations under these stable nighttime conditions, and this is seen in the time series of PM2.5 in Fig. 6. To account for misrepresentation of local boundary layer mixing, we post-process the model results by vertically averaging PM2.5 up to the simulated mixing height to eliminate the effect of underestimated mixing and up to the observed mixing height to provide a direct comparison against PM2.5 observations. Averaging up to the observed PBL height gives a substantial improvement in PM2.5 levels compared to observations, particularly for the episodes of 21–25 October and 27 October–1 November, when the model underestimates the PBL height. The simulated mean surface PM2.5 concentration during the period is reduced from 169 to 118 µg m−3 (the observed mean is 129 µg m−3); the root-mean-square error (RMSE) is reduced from 94 to 65 µg m−3; and the biases in NO3, NH4, and BC are significantly reduced. For a more detailed analysis of component-level sensitivity to boundary layer mixing, see Fig. S1 and Table S5 in the Supplement.
These results highlight the importance of representing PBL mixing well for accurate reproduction of surface pollutant levels. We note a steady decline in PBL height over the pollution episode during 21–25 October, and PM2.5 shows a consistent build-up over this period. This provides observational evidence for the radiative feedback between aerosol concentrations and mixing height, and this appears to be captured relatively well by the model, as shown in previous studies (Y. Gao et al., 2015). Further improvement in simulation of surface pollutant concentrations requires additional research on representation of PBL mixing processes in urban environments. Profiles of aerosol and meteorological variables from high-resolution lidar measurements provide an important aid to such investigations.
4.3 Regional NH3 emissions
The aerosol components NO3 and NH4 are overestimated in these simulations, as shown in Fig. 5. These components are governed by secondary production from their gaseous precursors, NO2 and NH3. Since the concentration of NO2 is close to that observed, we perform a short sensitivity study over the pollution episode from 21–25 October with NH3 emissions over the North China Plain reduced by 50 % to explore the response of NO3 and NH4 to ammonia emissions in the model. We find that the reduction in NH3 emissions reduces NH4 and NO3 concentrations substantially and brings them closer to observations (see Table 5 and Fig. S3). This is likely because NH3 is the limiting reactant in the formation of NH4NO3 that directly controls the concentration of both NH4 and NO3 aerosols in the North China Plain (M. Gao et al., 2016; D. Chen et al., 2016). However, reduction in SO4 concentrations is small (1 µg m−3) because SO4 formation is only indirectly associated with NH3 availability (Tsimpidi et al., 2007). Total PM2.5 concentrations are reduced by approximately 26 %, bringing them closer to observed concentrations. Ammonia emissions were reported to be 1574 kt yr−1 over the Beijing–Tianjin–Hebei region in 2010 (Zhou et al., 2015), while those in the MEIC emission inventory used here are only 540 kt yr−1. Given that our NH3 emissions are already low compared with other studies (Kang et al., 2016), we do not reduce them further in this study. However, we have demonstrated that PM2.5 concentrations during this period are highly sensitive to NH3 emissions, consistent with the findings of other studies (Zhang et al., 2016), and highlight this issue for further investigation.
The Asia-Pacific Economic Cooperation (APEC) summit was held from 10–12 November 2014 in Beijing and was the focus of short-term emission controls to ensure good air quality over the period. Emission controls were applied in Beijing and surrounding regions, including Tianjin; the provinces of Hebei, Shanxi, and Shandong; and the Inner Mongolia autonomous region. More than 460 businesses with high emissions in Beijing were required to limit or stop their production during 3–12 November 2014 (Tang et al., 2015; H. Wang et al., 2016; Guo et al., 2016). The number of private vehicles in operation over this period was reduced by about 50 % through odd–even license-plate restrictions. Furthermore, 9300 enterprises were suspended, 3900 enterprises were ordered to limit production, and more than 40 000 construction sites were shut down across the northern China region (Y. Wang et al., 2016; Tang et al., 2015). The start-up of municipal winter heating systems was delayed until 15 November, after the summit. Previous studies report that implementation of these emission controls resulted in significant impacts on regional pollutant transport and local pollutant contributions (Meng et al., 2014; Sun et al., 2016b; Gao et al., 2017).
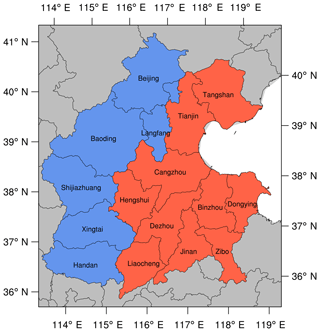
Figure 7Map of districts where major emission controls were implemented during the APEC period. During phase 1 emissions were focussed on Beijing and western Hebei (blue), and in phase 2 additional controls were applied over other parts of the North China Plain (red).
Table 6Emission controls during APEC period.
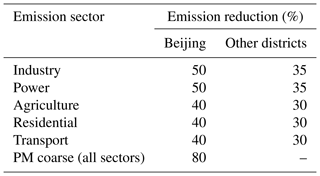
APEC1: Beijing, Langfang, Baoding, Shijiazhuang, Xingtai, and Handan. APEC2: APEC1 plus Tangshan, Tianjin, Cangzhou, Hengshui, Dezhou, Binzhou, Dongying, Zibo, Jinan, and Liaocheng.
Previous model studies of the APEC period have adopted different estimates of the emission reductions imposed (Guo et al., 2016; Gao et al., 2017; Wen et al., 2016; H. Liu et al., 2017; Wang et al., 2017). The most detailed study of emission reductions considered application of controls in two distinct phases (Wen et al., 2016), and we have chosen to implement these controls in our study, as the emission reductions applied are consistent with observation-based assessments of regional emission controls (K. Li et al., 2017). During the initial phase (APEC1; 3–5 November), emission controls were implemented in Beijing and the western side of the North China Plain. In a subsequent phase (APEC2; 6–12 November), controls were applied over a wider region including eastern Hebei and parts of Shandong. We represent these controls in the model over the districts shown in Fig. 7, following K. Li et al. (2017), and neglect smaller changes in emissions in other districts and more distant provinces. Controls were applied across different activity sectors, following Wen et al. (2016) and (K. Li et al., 2017; see Table 6).
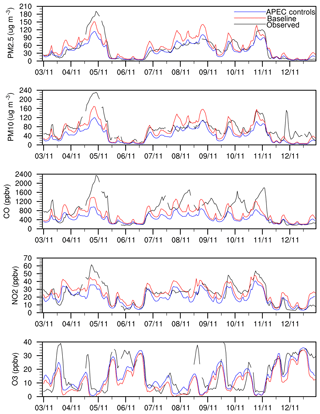
Figure 8Time series of surface pollutants averaged over the 12 measurement stations in Beijing during the APEC period.
Figure 8 shows the effect of these controls on key pollutants over the period 3–12 November. There is a minor pollution episode over 4–5 November, and the model underestimates PM2.5 levels over this period, even without emission controls. This may partly reflect an underestimation of OC, as the simulation of secondary inorganic aerosol for these 2 d is good (see Fig. 5). PM2.5 levels are very well matched in the period 6–9 November leading up to the summit, when emission controls are applied. PM10 levels are underestimated in the simulations, but this is influenced by what may be a minor dust episode on 11–12 November, when coarse particles were high but PM2.5 remained very low. Overall, the controls had a notable effect, reducing concentrations by 20 %–30 % for all pollutants except O3, which showed a small increase as expected with reduced levels of NO. Over the critical 10–12 November meeting period, PM2.5, PM10, CO, and NO2 were reduced by 21 %, 26 %, 22 %, and 22 %, respectively (see Table 7). The reduction in PM2.5 is very similar to the 22 % reduction found in previous studies (Gao et al., 2017). However, the absolute improvement in air quality over the meeting period was small, averaging less than 10 µg m−3 for PM2.5 and reflecting the relatively clean conditions over the period. Average PM2.5 in the baseline simulation was 39 µg m−3, close to the observed 36 µg m−3. Under these conditions the key air quality standard, a 24 h averaged PM2.5 of 75 µg m−3, corresponding to a Chinese Air Quality Index (AQI) value of 100, would have been met in the model simulation even without the controls.
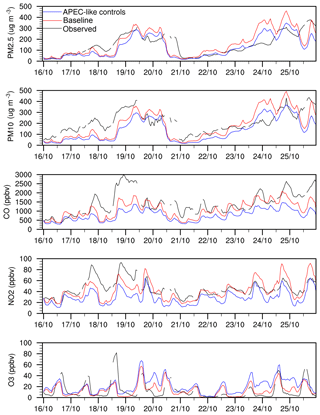
Figure 9Time series of surface pollutants averaged over the 12 measurement stations in Beijing during 16–25 October 2014.
To explore the importance of meteorological conditions in contributing to favourable air quality during the APEC period, we apply the same magnitude, location, and duration of emission controls to the major pollution episode at the end of October. Figure 9 shows the effect of these controls on key pollutants over 16–25 October. The controls reduced pollutant concentrations by a larger amount than during the APEC period, but the relative improvements of 23 %–38 % were very similar. The absolute pollutant concentrations were much higher than in November. This can be attributed to lower wind speeds and to winds from the south and east bringing air from across the North China Plain, in contrast to the APEC period, which experienced higher wind speeds and more frequent air from the clean northwest sector (see Fig. 2). The 3 d baseline average concentrations over 23–25 October for PM2.5, PM10, CO, and NO2 were 279 µg m−3, 310 µg m−3, 1.48 ppm, and 53 ppb, respectively, substantially exceeding air quality standards. The difference in baseline PM2.5 concentrations between the October and November periods without emission controls, 279 vs. 39 µg m−3, highlights the dominant role played by meteorology in bringing clean air during APEC. The emission controls have a much larger absolute effect during the October episode than in the APEC period, with reductions in PM2.5 of 65 µg m−3 for 23–25 October, bringing average PM2.5 levels down to 214 µg m−3. However, this is insufficient in meeting the standard needed for clean air of 75 µg m−3. This indicates that the same emission control policies applied would have failed to produce the desired results if the meeting had been held at the end of October.
Table 8 presents the effect on aerosol components and gas-phase pollutants at IAP tower. During the emission controls in both the polluted October and cleaner November periods, primary components were reduced by 31 %–34 %, while secondary components were reduced by only 3 %–17 %. This suggests that pollution episodes dominated by primary aerosols may be more easily controlled and have serious implications for winter haze episodes over the North China Plain because much of the increase in aerosol loading is contributed by regional secondary aerosols (see Sun et al., 2016b). The percentage reduction in SO4 (14 %–17 %) may be overestimated, as some fraction of SO4 mass for which chemical formation pathway remains unknown is treated as primary aerosol in the model. Similarly, the percentage reduction in OC may be overestimated because all OC is primary in the model.
To investigate the feasibility of meeting air quality standards during pollution episodes such as those on 21–25 October, we ran the model with all anthropogenic emissions removed over the North China Plain from 16–25 October. The 3 d average concentrations over 23–25 October showed substantial reductions: 83 % for PM2.5, 82 % for PM10, 79 % for CO, 99 % for NO2, and 88 % for SO2. Average PM2.5 concentrations were reduced from 279 to 48 µg m−3, demonstrating that air quality standards can be met on highly polluted days, at least in theory, under the most stringent emission controls. From this simulation, and accounting for nonlinearity in secondary aerosol formation, we estimate that a 92 % emission reduction over the 10 d period would have been needed to keep the average concentrations for 23–25 October below 75 µg m−3. Even accounting for model overestimation of average PM2.5 during this period, driven principally by the positive bias on 24 October, we find that an 85 % emission reduction would be required, which is substantially more than what is feasible realistically. It is clear from this analysis that emission controls would need to be applied over a much wider area over neighbouring provinces if the air quality standards in Beijing are to be met.
Finally, we analyse the full simulation period (12 October–19 November) to investigate how many days would meet the “blue-sky” criteria of 24 h average PM2.5 concentrations less than 75 µg m−3 with and without APEC-like controls. We conducted another simulation with APEC 2 controls implemented over the full period and found a reduction in daily average PM2.5 of 26 %±6 % and a reduction of 23 %±4 % for haze days with the daily mean . Since primary and secondary aerosol components can respond differently to emission controls, we use component-level fractional reductions from the model and apply them to the observed component concentrations to find the reduction in total PM. This is approximately 22 % for both October and November periods based on our APEC control runs, suggesting that this scaling is appropriate and robust for uncertainties in model aerosol composition. To generate an emission control scenario over the full period, we reduce daily mean observed PM2.5 concentrations by 22 % for all days except 3–12 November, when controls were actually in place. For the 3–12 November period, we apply an increase of 16 %–33 % based on the APEC controls run to represent conditions with no controls. With these scenarios we find that 15 of the 39 d considered failed to meet the blue-sky criteria of daily average PM2.5 concentrations less than 75 µg m−3 without controls, and this fell to 13 d when the controls were implemented, a modest decrease of 2 d (see Fig. 10). However, if we choose a higher threshold of 150 µg m−3 (AQI of 200), the emission controls appear to be more effective, reducing the number of exceedances from 8 to 5 d, and with a threshold of 200 µg m−3 (AQI of 250), the number of exceedances falls from 4 to 1 d.
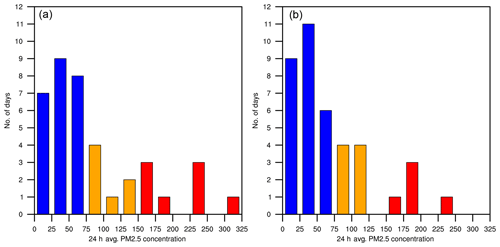
Figure 10Frequency distribution of daily average PM2.5 over 12 October–19 November 2014, showing the number of days meeting thresholds of 75 µg m−3 (blue) and 150 µg m−3 (blue plus orange) without (a) and with (b) emission controls.
To organize a 3 d meeting such as APEC successfully, all 3 d must individually meet the chosen air quality criteria. We find that without emission controls, only 9 out of 37 possible 3 d time slots in our simulation period meet the criteria, including only 3 out of the 8 available during the APEC period of 3–12 November. Under the emission controls, the meeting could have been organized in 14 out of the 37 slots, including all 8 during early November. This suggests that the emission controls were only sufficient to provide an additional five time slots to hold a 3 d event meeting the criteria. Interestingly, these all occur during the APEC period, highlighting that while favourable weather conditions were vital for meeting the air quality criteria, the emission controls provided critical support in achieving the 75 µg m−3 threshold needed to realize blue-sky conditions. Specifically, in the absence of emission controls the first day of the APEC meeting (10 November) would have exceeded the air quality standards. In this respect, it is reasonable to claim that the APEC emission controls were a success. However, it is clear that favourable meteorology was essential in making it possible for the emission controls to produce the marginal improvements needed to meet the air quality standards.
It should be noted that 23 out of the 37 possible 3 d time periods (more than 60 %) would not have met the standards even under the emission controls applied. It is therefore clear that much more stringent controls are needed in future to counter the effect of unfavourable meteorological conditions. While greater reductions in the magnitude of emissions are required, it is important that these are applied over a much larger area, including in the neighbouring provinces that surround the North China Plain.
Table 7Influence of emission controls averaged over Beijing air quality stations in October and November.
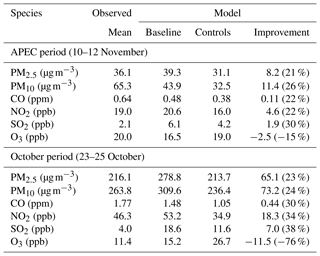
We have demonstrated that using a high-resolution nested air quality model we can reproduce the observed hourly variation of major pollutants in Beijing during October–November 2014 reasonably well. We capture the synoptic drivers of air quality well, including the build-up of pollutants during pollution episodes and the subsequent cleaning effect of winds from the northwest. The concentrations of PM2.5, the dominant pollutant in this season, are reproduced well, and we show that where the model is biased high, typically during nighttime, underlying weaknesses in the treatment of turbulent mixing in the planetary boundary layer are often responsible. We show that use of two-way nesting to high resolution brings a substantial benefit in reproducing observed pollutant concentrations, even when comparing at the coarsest resolution used. Thorough evaluation against aerosol composition measurements over the period highlights some weaknesses in representation of key aerosol components, particularly the balance between SO4, NO3, and NH3, which requires more detailed analysis.
We show that short-term emission controls played a valuable role in improving air quality over the APEC period but that their overall contribution was relatively small, with average reductions of 20 %–26 % for key pollutants. Without the controls, average PM2.5 levels are likely to have exceeded the national standard of 75 µg m−3 on 10 November, the first day of the APEC meeting, but the effects were largely incremental, highlighting the important role played by favourable meteorology during the period. If the APEC meeting had been held at a different time, particularly at the end of October, air quality standards would not have been achieved with the emission controls applied. We find that the relative effect of the controls during the pollution episodes of late October is very similar to that during the clean APEC period, averaging 23 % for PM2.5. Much greater emission reductions of at least 85 % would have been needed over the North China Plain region to bring pollutant levels down to meet air quality standards. It is clear that under the stable meteorological conditions present during these pollution episodes, much more stringent emission controls are needed than those that were applied and that these need to be implemented over a much wider region of northern China. Our study demonstrates the value of short-term emission controls but highlights that long-term, sustained emission reductions on a regional scale are required to bring blue skies to Beijing.
The WRF-Chem code is available from http://www2.mmm.ucar.edu/wrf/users/download/ (Skamarock et al., 2008). The name list for the model and surface pollutant distributions generated in this study are available from the Lancaster University data archive at https://doi.org/10.17635/lancaster/researchdata/300 (Ansari, 2019).
The supplement related to this article is available online at: https://doi.org/10.5194/acp-19-8651-2019-supplement.
TUA, OW, and ZW designed this study, and TUA performed the model simulations and analysis. JL provided emission data and expertise on the model set-up; TY provided the lidar data and guidance on deriving PBL height; and YS, WX, and ZW provided measurement data from IAP tower. TUA and OW prepared the paper, with input from all co-authors.
The authors declare that they have no conflict of interest.
Oliver Wild thanks the UK Natural Environment Research Council for support under grants NE/N006925/1 and NE/N006976/1. Yele Sun thanks the National Natural Science Foundation of China (grant no. 91744207). Jie Li thanks the National Natural Science Foundation of China (grant nos. 41571130034 and 91744203). Ting Yang thanks the Strategic Priority Research Program of the Chinese Academy of Sciences (grant no. XDA19040203). Tabish Umar Ansari thanks Adam Philips, Mary Haley, Dennis Shea, Rashed Mahmood, Rick Brownrigg, and Karin Meier-Fleischer of the “ncl-talk” online forum for providing assistance on data visualization. Tabish Umar Ansari thanks the Lancaster Environment Centre for providing funding to carry out his research.
This research has been supported by the Natural Environment Research Council (grant nos. NE/N006925/1 and NE/N006976/1), the National Natural Science Foundation of China (grant nos. 91744207, 41571130034 and 91744203), and the Strategic Priority Research Program of the Chinese Academy of Sciences (grant no. XDA19040203).
This paper was edited by Andreas Hofzumahaus and reviewed by two anonymous referees.
Ansari, T. U.: Surface meteorological fields and key pollutant concentrations, https://doi.org/10.17635/lancaster/researchdata/300, 2019. a
Batterman, S., Xu, L., Chen, F., Chen, F., and Zhong, X.: Characteristics of PM2.5 concentrations across Beijing during 2013–2015, Atmos. Environ., 145, 104–114, https://doi.org/10.1016/j.atmosenv.2016.08.060, 2016. a
Bieser, J., Aulinger, A., Matthias, V., Quante, M., and Denier Van Der Gon, H. A. C.: Vertical emission profiles for Europe based on plume rise calculations, Environ. Pollut., 159, 2935–2946, https://doi.org/10.1016/j.envpol.2011.04.030, 2011. a
Chen, D., Liu, Z., Fast, J., and Ban, J.: Simulations of sulfate–nitrate–ammonium (SNA) aerosols during the extreme haze events over northern China in October 2014, Atmos. Chem. Phys., 16, 10707–10724, https://doi.org/10.5194/acp-16-10707-2016, 2016. a, b, c, d, e, f, g
Chen, F. and Dudhia, J.: Coupling an Advanced Land Surface–Hydrology Model with the Penn State–NCAR MM5 Modeling System. Part II: Preliminary Model Validation, Mon. Weather Rev., 129, 587–604, https://doi.org/10.1175/1520-0493(2001)129<0587:CAALSH>2.0.CO;2, 2001. a
Chen, H., Li, J., Ge, B., Yang, W., Wang, Z., Huang, S., Wang, Y., Yan, P., Li, J., and Zhu, L.: Modeling study of source contributions and emergency control effects during a severe haze episode over the Beijing-Tianjin-Hebei area, Science China Chemistry, 58, 1403–1415, https://doi.org/10.1007/s11426-015-5458-y, 2015. a, b
Chen, W., Tang, H., and Zhao, H.: Diurnal, weekly and monthly spatial variations of air pollutants and air quality of Beijing, Atmospheric Environment, 119, 21–34, https://doi.org/10.1016/j.atmosenv.2015.08.040, 2015. a
Chen, Z., Xu, B., Cai, J., and Gao, B.: Understanding temporal patterns and characteristics of air quality in Beijing: A local and regional perspective, Atmos. Environ., 127, 303–315, https://doi.org/10.1016/j.atmosenv.2015.12.011, 2016. a
Clough, S. A., Shephard, M. W., Mlawer, E. J., Delamere, J. S., Iacono, M. J., Cady-Pereira, K., Boukabara, S., and Brown, P. D.: Atmospheric radiative transfer modeling: A summary of the AER codes, J. Quant. Spectrosc. Ra., 91, 233–244, https://doi.org/10.1016/j.jqsrt.2004.05.058, 2005. a
Fast, J. D., Gustafson, W. I., Easter, R. C., Zaveri, R. A., Barnard, J. C., Chapman, E. G., Grell, G. A., and Peckham, S. E.: Evolution of ozone, particulates, and aerosol direct radiative forcing in the vicinity of Houston using a fully coupled meteorology-chemistry-aerosol model, J. Geophys. Res.-Atmos., 111, 1–29, https://doi.org/10.1029/2005JD006721, 2006. a
Gao, J., Peng, X., Chen, G., Xu, J., Shi, G.-L., Zhang, Y.-C., and Feng, Y.-C.: Insights into the chemical characterization and sources of PM2.5 in Beijing at a 1-h time resolution, Sci. Total Environ., 542, 162–171, https://doi.org/10.1016/j.scitotenv.2015.10.082, 2016. a
Gao, M., Carmichael, G. R., Wang, Y., Saide, P. E., Yu, M., Xin, J., Liu, Z., and Wang, Z.: Modeling study of the 2010 regional haze event in the North China Plain, Atmos. Chem. Phys., 16, 1673–1691, https://doi.org/10.5194/acp-16-1673-2016, 2016. a, b, c, d, e, f
Gao, M., Carmichael, G. R., Saide, P. E., Lu, Z., Yu, M., Streets, D. G., and Wang, Z.: Response of winter fine particulate matter concentrations to emission and meteorology changes in North China, Atmos. Chem. Phys., 16, 11837–11851, https://doi.org/10.5194/acp-16-11837-2016, 2016. a, b, c
Gao, M., Liu, Z., Wang, Y., Lu, X., Ji, D., Wang, L., Li, M., Wang, Z., Zhang, Q., and Carmichael, G. R.: Distinguishing the roles of meteorology, emission control measures, regional transport, and co-benefits of reduced aerosol feedbacks in “APEC Blue”, Atmos. Environ., 167, 476–486, https://doi.org/10.1016/j.atmosenv.2017.08.054, 2017. a, b, c, d, e, f
Gao, Y., Liu, X., Zhao, C., and Zhang, M.: Emission controls versus meteorological conditions in determining aerosol concentrations in Beijing during the 2008 Olympic Games, Atmos. Chem. Phys., 11, 12437–12451, https://doi.org/10.5194/acp-11-12437-2011, 2011. a
Gao, Y., Zhang, M., Liu, Z., Wang, L., Wang, P., Xia, X., Tao, M., and Zhu, L.: Modeling the feedback between aerosol and meteorological variables in the atmospheric boundary layer during a severe fog–haze event over the North China Plain, Atmos. Chem. Phys., 15, 4279–4295, https://doi.org/10.5194/acp-15-4279-2015, 2015. a, b
Grell, G. A., Peckham, S. E., Schmitz, R., McKeen, S. A., Frost, G., Skamarock, W. C., and Eder, B.: Fully coupled “online” chemistry within the WRF model, Atmos. Environ., 39, 6957–6975, https://doi.org/10.1016/j.atmosenv.2005.04.027, 2005. a
Guenther, A. B., Jiang, X., Heald, C. L., Sakulyanontvittaya, T., Duhl, T., Emmons, L. K., and Wang, X.: The Model of Emissions of Gases and Aerosols from Nature version 2.1 (MEGAN2.1): an extended and updated framework for modeling biogenic emissions, Geosci. Model Dev., 5, 1471–1492, https://doi.org/10.5194/gmd-5-1471-2012, 2012. a, b
Guo, J., He, J., Liu, H., Miao, Y., Liu, H., and Zhai, P.: Impact of various emission control schemes on air quality using WRF-Chem during APEC China 2014, Atmos. Environ., 140, 311–319, https://doi.org/10.1016/j.atmosenv.2016.05.046, 2016. a, b, c, d, e
Guo, S., Hu, M., Zamora, M. L., Peng, J., Shang, D., Zheng, J., Du, Z., Wu, Z., Shao, M., Zeng, L., Molina, M. J., and Zhang, R.: Elucidating severe urban haze formation in China, P. Natl. Acad. Sci. USA, 111, 17373–17378, https://doi.org/10.1073/pnas.1419604111, 2014. a
Hong, S.-Y., Noh, Y., and Dudhia, J.: A New Vertical Diffusion Package with an Explicit Treatment of Entrainment Processes, Mon. Weather Rev., 134, 2318–2341, https://doi.org/10.1175/MWR3199.1, 2006. a, b
Kajino, M., Ueda, H., Han, Z., Kudo, R., and Inomata, Y.: Synergy between air pollution and urban meteorological changes through aerosol-radiation-diffusion feedback – A case study of Beijing in January, Atmos. Environ., 171, 98–110, https://doi.org/10.1016/j.atmosenv.2017.10.018, 2017. a
Kang, Y., Liu, M., Song, Y., Huang, X., Yao, H., Cai, X., Zhang, H., Kang, L., Liu, X., Yan, X., He, H., Zhang, Q., Shao, M., and Zhu, T.: High-resolution ammonia emissions inventories in China from 1980 to 2012, Atmos. Chem. Phys., 16, 2043–2058, https://doi.org/10.5194/acp-16-2043-2016, 2016. a
Krotkov, N. A., McLinden, C. A., Li, C., Lamsal, L. N., Celarier, E. A., Marchenko, S. V., Swartz, W. H., Bucsela, E. J., Joiner, J., Duncan, B. N., Boersma, K. F., Veefkind, J. P., Levelt, P. F., Fioletov, V. E., Dickerson, R. R., He, H., Lu, Z., and Streets, D. G.: Aura OMI observations of regional SO2 and NO2 pollution changes from 2005 to 2015, Atmos. Chem. Phys., 16, 4605–4629, https://doi.org/10.5194/acp-16-4605-2016, 2016. a
Lelieveld, J., Evans, J. S., Fnais, M., Giannadaki, D., and Pozzer, A.: The contribution of outdoor air pollution sources to premature mortality on a global scale, Nature, 525, 367–71, https://doi.org/10.1038/nature15371, 2015. a
Li, G., Bei, N., Cao, J., Huang, R., Wu, J., Feng, T., Wang, Y., Liu, S., Zhang, Q., Tie, X., and Molina, L. T.: A possible pathway for rapid growth of sulfate during haze days in China, Atmos. Chem. Phys., 17, 3301–3316, https://doi.org/10.5194/acp-17-3301-2017, 2017. a
Li, K., Li, J., Wang, W., Tong, S., Liggio, J., and Ge, M.: Evaluating the effectiveness of joint emission control policies on the reduction of ambient VOCs: Implications from observation during the 2014 APEC summit in suburban Beijing, Atmos. Environ., 164, 117–127, https://doi.org/10.1016/j.atmosenv.2017.05.050, 2017. a, b, c, d, e
Li, M., Zhang, Q., Kurokawa, J.-I., Woo, J.-H., He, K., Lu, Z., Ohara, T., Song, Y., Streets, D. G., Carmichael, G. R., Cheng, Y., Hong, C., Huo, H., Jiang, X., Kang, S., Liu, F., Su, H., and Zheng, B.: MIX: a mosaic Asian anthropogenic emission inventory under the international collaboration framework of the MICS-Asia and HTAP, Atmos. Chem. Phys., 17, 935–963, https://doi.org/10.5194/acp-17-935-2017, 2017. a
Li, X., Zhang, Q., Zhang, Y., Zheng, B., Wang, K., Chen, Y., Wallington, T. J., Han, W., Shen, W., Zhang, X., and He, K.: Source contributions of urban PM2.5 in the Beijing–Tianjin–Hebei region: Changes between 2006 and 2013 and relative impacts of emissions and meteorology, Atmos. Environ., 123, 229–239, https://doi.org/10.1016/j.atmosenv.2015.10.048, 2015. a
Liang, P., Zhu, T., Fang, Y., Li, Y., Han, Y., Wu, Y., Hu, M., and Wang, J.: The role of meteorological conditions and pollution control strategies in reducing air pollution in Beijing during APEC 2014 and Victory Parade 2015, Atmos. Chem. Phys., 17, 13921–13940, https://doi.org/10.5194/acp-17-13921-2017, 2017. a, b
Lin, Y.-L., Farley, R. D., and Orville, H. D.: Bulk Parameterization of the Snow Field in a Cloud Model, J. Clim. Appl. Meteorol., 22, 1065–1092, https://doi.org/10.1175/1520-0450(1983)022<1065:BPOTSF>2.0.CO;2, 1983. a
Liu, H., Liu, C., Xie, Z., Li, Y., Huang, X., and Wang, S.: OPEN A paradox for air pollution controlling in China revealed by “APEC Blue” and “Parade Blue”, Nature Publishing Group, 1–13, https://doi.org/10.1038/srep34408, 2016. a
Liu, H., He, J., Guo, J., Miao, Y., Yin, J., Wang, Y., Xu, H., Liu, H., Yan, Y., Li, Y., and Zhai, P.: The blue skies in Beijing during APEC 2014 : A quantitative assessment of emission control efficiency and meteorological influence, Atmos. Environ., 167, 235–244, https://doi.org/10.1016/j.atmosenv.2017.08.032, 2017. a
Liu, T., Gong, S., He, J., Yu, M., Wang, Q., Li, H., Liu, W., Zhang, J., Li, L., Wang, X., Li, S., Lu, Y., Du, H., Wang, Y., Zhou, C., Liu, H., and Zhao, Q.: Attributions of meteorological and emission factors to the 2015 winter severe haze pollution episodes in China's Jing-Jin-Ji area, Atmos. Chem. Phys., 17, 2971–2980, https://doi.org/10.5194/acp-17-2971-2017, 2017. a
Ma, J., Zhang, W., Xu, Y., Xie, H., Xu, X., Li, Q., Gao, J., Zheng, J., Xian, Z., Li, X., and Sheng, L. (Eds.): China Statistical Yearbook, China Statistics Publishing House, September Edn., 2014. a
Mailler, S., Khvorostyanov, D., and Menut, L.: Impact of the vertical emission profiles on background gas-phase pollution simulated from the EMEP emissions over Europe, Atmos. Chem. Phys., 13, 5987–5998, https://doi.org/10.5194/acp-13-5987-2013, 2013. a
Matsui, H., Koike, M., Kondo, Y., Takegawa, N., Kita, K., Miyazaki, Y., Hu, M., Chang, S. Y., Blake, D. R., Fast, J. D., Zaveri, R. A., Streets, D. G., Zhang, Q., and Zhu, T.: Spatial and temporal variations of aerosols around Beijing in summer 2006: Model evaluation and source apportionment, J. Geophys. Res. Atmos., 114, 1–22, https://doi.org/10.1029/2008JD010906, 2009. a, b
Meng, R., Zhao, F. R., Sun, K., Zhang, R., Huang, C., Yang, J., Müller, R., and Thenkabail, P. S.: Analysis of the 2014 “APEC Blue” in Beijing Using More than One Decade of Satellite Observations: Lessons Learned from Radical Emission Control Measures, Remote Sensing, 7, 15224–15243, https://doi.org/10.3390/rs71115224, 2014. a
Mlawer, E. J., Taubman, S. J., Brown, P. D., Iacono, M. J., and Clough, S. A.: Radiative transfer for inhomogeneous atmospheres: RRTM, a validated correlated-k model for the longwave, J. Geophys. Res., 102, 16663, https://doi.org/10.1029/97JD00237, 1997. a
Monin, A. and Obukhov, A.: Basic laws of turbulent mixing in the surface layer of the atmosphere, Contrib. Geophys. Inst. Acad. Sci. USSR, 24, 163–187, 1954. a
Ng, N. L., Herndon, S. C., Trimborn, A., Canagaratna, M. R., Croteau, P. L., Onasch, T. B., Sueper, D., Worsnop, D. R., Zhang, Q., Sun, Y. L., and Jayne, J. T.: An Aerosol Chemical Speciation Monitor (ACSM) for Routine Monitoring of the Composition and Mass Concentrations of Ambient Aerosol, Aerosol Sci. Technol., 45, 780–794, https://doi.org/10.1080/02786826.2011.560211, 2011. a
Seinfeld, J. and Pandis, S.: Atmospheric Chemistry and Physics: From Air Pollution to Climate Change, Wiley, 2006. a
Shang, X., Zhang, K., Meng, F., Wang, S., Lee, M., Suh, I., Kim, D., Jeon, K., Park, H., Wang, X., and Zhao, Y.: Characteristics and source apportionment of fine haze aerosol in Beijing during the winter of 2013, Atmos. Chem. Phys., 18, 2573–2584, https://doi.org/10.5194/acp-18-2573-2018, 2018. a
Skamarock, W. C., Klemp, J. B., Dudhia, J., Gill, D. O., Barker, D. M., Duda, M. G., Huang, X.-Y., Wang, W., and Powers, J. G.: A Description of the Advanced Research WRF Version 3. NCAR Tech. Note NCAR/TN-475+STR, 113 pp. https://doi.org/10.5065/D68S4MVH, 2008. a
Sun, Y., Wang, Z., Dong, H., Yang, T., Li, J., Pan, X., Chen, P., and Jayne, J. T.: Characterization of summer organic and inorganic aerosols in Beijing, China with an Aerosol Chemical Speciation Monitor, Atmos. Environ., 51, 250–259, https://doi.org/10.1016/J.ATMOSENV.2012.01.013, 2012. a
Sun, Y., Chen, C., Zhang, Y., Xu, W., Zhou, L., Cheng, X., Zheng, H., Ji, D., Li, J., Tang, X., Fu, P., and Wang, Z.: Rapid formation and evolution of an extreme haze episode in Northern China during winter 2015, Sci. Rep., 6, 27151, https://doi.org/10.1038/srep27151, 2016a. a
Sun, Y., Wang, Z., Wild, O., Xu, W., Chen, C., Fu, P., Du, W., Zhou, L., Zhang, Q., Han, T., Wang, Q., Pan, X., Zheng, H., Li, J., Guo, X., Liu, J., and Worsnop, D. R.: “APEC Blue”: Secondary Aerosol Reductions from Emission Controls in Beijing, Sci. Rep., 6, 20668, https://doi.org/10.1038/srep20668, 2016b. a, b, c, d, e
Tang, G., Zhu, X., Hu, B., Xin, J., Wang, L., Münkel, C., Mao, G., and Wang, Y.: Impact of emission controls on air quality in Beijing during APEC 2014: lidar ceilometer observations, Atmos. Chem. Phys., 15, 12667–12680, https://doi.org/10.5194/acp-15-12667-2015, 2015. a, b
Tie, X., Zhang, Q., He, H., Cao, J., Han, S., Gao, Y., Li, X., and Jia, X. C.: A budget analysis of the formation of haze in Beijing, Atmos. Environ., 100, 25–36, https://doi.org/10.1016/j.atmosenv.2014.10.038, 2014. a
Tsimpidi, A. P., Karydis, V. A., and Pandis, S. N.: Response of Inorganic Fine Particulate Matter to Emission Changes of Sulfur Dioxide and Ammonia: The Eastern United States as a Case Study, J. Air Waste Manage., 57, 1489–1498, https://doi.org/10.3155/1047-3289.57.12.1489, 2007. a
Wang, G., Cheng, S., Wei, W., Yang, X., Wang, X., Jia, J., Lang, J., and Lv, Z.: Characteristics and emission-reduction measures evaluation of PM2.5 during the two major eventsAPEC and Parade, Sci. Total Environ.t, 595, 81–92, https://doi.org/10.1016/j.scitotenv.2017.03.231, 2017. a, b
Wang, H., Zhao, L., Xie, Y., and Hu, Q.: “APEC blue” – The effects and implications of joint pollution prevention and control program, Sci. Total Environ., 553, 429–438, https://doi.org/10.1016/j.scitotenv.2016.02.122, 2016a. a
Wang, L. T., Wei, Z., Yang, J., Zhang, Y., Zhang, F. F., Su, J., Meng, C. C., and Zhang, Q.: The 2013 severe haze over southern Hebei, China: model evaluation, source apportionment, and policy implications, Atmos. Chem. Phys., 14, 3151–3173, https://doi.org/10.5194/acp-14-3151-2014, 2014. a
Wang, Y., Zhang, Y., Jay, J., Foy, B. D., Guo, B., and Zhang, Y.: Relative impact of emissions controls and meteorology on air pollution mitigation associated with the Asia-Pacific Economic Cooperation (APEC) conference in Beijing , China, Sci. Total Environ., 571, 1467–1476, https://doi.org/10.1016/j.scitotenv.2016.06.215, 2016b. a, b, c
Wen, W., Cheng, S., Chen, X., Wang, G., and Li, S.: Impact of emission control on PM2.5 and the chemical composition change in Beijing-Tianjin-Hebei during the APEC summit 2014, Environ. Sci. Pollut. Res., 23, 4509–4521, https://doi.org/10.1007/s11356-015-5379-5, 2016. a, b, c
Westervelt, D., Horowitz, L., Naik, V., Tai, A., Fiore, A., and Mauzerall, D.: Quantifying PM2.5-meteorology sensitivities in a global climate model, Atmos. Environ., 142, 43–56, https://doi.org/10.1016/J.ATMOSENV.2016.07.040, 2016. a
Wiedinmyer, C., Akagi, S. K., Yokelson, R. J., Emmons, L. K., Al-Saadi, J. A., Orlando, J. J., and Soja, A. J.: The Fire INventory from NCAR (FINN): a high resolution global model to estimate the emissions from open burning, Geosci. Model Dev., 4, 625–641, https://doi.org/10.5194/gmd-4-625-2011, 2011. a
Wild, O., Zhu, X., and Prather, M. J.: Fast-J: Accurate simulation of in- and below-cloud photolysis in tropospheric chemical models, J. Atmos. Chem., 37, 245–282, https://doi.org/10.1023/A:1006415919030, 2000. a
Xu, W. Q., Sun, Y. L., Chen, C., Du, W., Han, T. T., Wang, Q. Q., Fu, P. Q., Wang, Z. F., Zhao, X. J., Zhou, L. B., Ji, D. S., Wang, P. C., and Worsnop, D. R.: Aerosol composition, oxidation properties, and sources in Beijing: results from the 2014 Asia-Pacific Economic Cooperation summit study, Atmos. Chem. Phys., 15, 13681–13698, https://doi.org/10.5194/acp-15-13681-2015, 2015. a, b
Yan, D., Lei, Y., Shi, Y., Zhu, Q., Li, L., and Zhang, Z.: Evolution of the spatiotemporal pattern of PM2.5 concentrations in China – A case study from the Beijing-Tianjin-Hebei region, Atmos. Environ., 183, 225–233, https://doi.org/10.1016/j.atmosenv.2018.03.041, 2018. a
Yang, L., Wu, Y., Davis, J. M., and Hao, J.: Estimating the effects of meteorology on PM2.5 reduction during the 2008 Summer Olympic Games in Beijing, China, Front. Environ. Sci. Eng., 5, 331–341, https://doi.org/10.1007/s11783-011-0307-5, 2011. a
Yang, T., Wang, Z., Zhang, W., Gbaguidi, A., Sugimoto, N., Wang, X., Matsui, I., and Sun, Y.: Technical note: Boundary layer height determination from lidar for improving air pollution episode modeling: development of new algorithm and evaluation, Atmos. Chem. Phys., 17, 6215–6225, https://doi.org/10.5194/acp-17-6215-2017, 2017. a, b
Zaveri, R. A. and Peters, L. K.: A new lumped structure photochemical mechanism for large-scale applications, J. Geophys. Res., 104, 30387, https://doi.org/10.1029/1999JD900876, 1999. a
Zaveri, R. A., Easter, R. C., Fast, J. D., and Peters, L. K.: Model for Simulating Aerosol Interactions and Chemistry (MOSAIC), J. Geophys. Res., 113, D13204, https://doi.org/10.1029/2007JD008782, 2008. a, b
Zhang, L., Wang, T., Lv, M., and Zhang, Q.: On the severe haze in Beijing during January 2013: Unraveling the effects of meteorological anomalies with WRF-Chem, Atmos. Environ., 104, 11–21, https://doi.org/10.1016/j.atmosenv.2015.01.001, 2015. a, b
Zhang, L., Shao, J., Lu, X., Zhao, Y., Hu, Y., Henze, D. K., Liao, H., Gong, S., and Zhang, Q.: Sources and Processes Affecting Fine Particulate Matter Pollution over North China: An Adjoint Analysis of the Beijing APEC Period, Environ. Sci. Techol., 50, 8731–8740, https://doi.org/10.1021/acs.est.6b03010, 2016. a, b
Zhao, J., Du, W., Zhang, Y., Wang, Q., Chen, C., Xu, W., Han, T., Wang, Y., Fu, P., Wang, Z., Li, Z., and Sun, Y.: Insights into aerosol chemistry during the 2015 China Victory Day parade: results from simultaneous measurements at ground level and 260 m in Beijing, Atmos. Chem. Phys., 17, 3215–3232, https://doi.org/10.5194/acp-17-3215-2017, 2017. a
Zheng, B., Tong, D., Li, M., Liu, F., Hong, C., Geng, G., Li, H., Li, X., Peng, L., Qi, J., Yan, L., Zhang, Y., Zhao, H., Zheng, Y., He, K., and Zhang, Q.: Trends in China's anthropogenic emissions since 2010 as the consequence of clean air actions, Atmos. Chem. Phys., 18, 14095–14111, https://doi.org/10.5194/acp-18-14095-2018, 2018. a, b, c
Zhong, J., Zhang, X., Dong, Y., Wang, Y., Liu, C., Wang, J., Zhang, Y., and Che, H.: Feedback effects of boundary-layer meteorological factors on cumulative explosive growth of PM2.5 during winter heavy pollution episodes in Beijing from 2013 to 2016, Atmos. Chem. Phys., 18, 247–258, https://doi.org/10.5194/acp-18-247-2018, 2018. a
Zhou, Y., Cheng, S., Lang, J., Chen, D., Zhao, B., Liu, C., Xu, R., and Li, T.: A comprehensive ammonia emission inventory with high-resolution and its evaluation in the Beijing-Tianjin-Hebei (BTH) region, China, Atmos. Environ., 106, 305–317, https://doi.org/10.1016/j.atmosenv.2015.01.069, 2015. a
Zhou, Y., Wang, Q., Huang, R., Liu, S., Tie, X., Su, X., Niu, X., Zhao, Z., Ni, H., Wang, M., Zhang, Y., and Cao, J.: Optical Properties of Aerosols and Implications for Radiative Effects in Beijing During the Asia-Pacific Economic Cooperation Summit 2014, J. Geophys. Res.-Atmos., 122, 10119–10132, https://doi.org/10.1002/2017JD026997, 2017. a