the Creative Commons Attribution 4.0 License.
the Creative Commons Attribution 4.0 License.
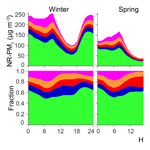
Submicron aerosol composition in the world's most polluted megacity: the Delhi Aerosol Supersite study
Shahzad Gani
Sahil Bhandari
Sarah Seraj
Dongyu S. Wang
Kanan Patel
Prashant Soni
Zainab Arub
Gazala Habib
Delhi, India, routinely experiences some of the world's highest urban particulate matter concentrations. We established the Delhi Aerosol Supersite study to provide long-term characterization of the ambient submicron aerosol composition in Delhi. Here we report on 1.25 years of highly time-resolved speciated submicron particulate matter (PM1) data, including black carbon (BC) and nonrefractory PM1 (NR-PM1), which we combine to develop a composition-based estimate of PM1 (“C-PM1” = BC + NR-PM1) concentrations.
We observed marked seasonal and diurnal variability in the concentration and composition of PM1 owing to the interactions of sources and atmospheric processes. Winter was the most polluted period of the year, with average C-PM1 mass concentrations of ∼210 µg m−3. The monsoon was hot and rainy, consequently making it the least polluted (C-PM1 ∼50 µg m−3) period. Organics constituted more than half of the C-PM1 for all seasons and times of day. While ammonium, chloride, and nitrate each were ∼10 % of the C-PM1 for the cooler months, BC and sulfate contributed ∼5 % each. For the warmer periods, the fractional contribution of BC and sulfate to C-PM1 increased, and the chloride contribution decreased to less than 2 %. The seasonal and diurnal variation in absolute mass loadings were generally consistent with changes in ventilation coefficients, with higher concentrations for periods with unfavorable meteorology – low planetary boundary layer height and low wind speeds. However, the variation in C-PM1 composition was influenced by temporally varying sources, photochemistry, and gas–particle partitioning. During cool periods when wind was from the northwest, episodic hourly averaged chloride concentrations reached 50–100 µg m−3, ranking among the highest chloride concentrations reported anywhere in the world.
We estimated the contribution of primary emissions and secondary processes to Delhi's submicron aerosol. Secondary species contributed almost 50 %–70 % of Delhi's C-PM1 mass for the winter and spring months and up to 60 %–80 % for the warmer summer and monsoon months. For the cooler months that had the highest C-PM1 concentrations, the nighttime sources were skewed towards primary sources, while the daytime C-PM1 was dominated by secondary species. Overall, these findings point to the important effects of both primary emissions and more regional atmospheric chemistry on influencing the extreme particle concentrations that impact the Delhi megacity region. Future air quality strategies considering Delhi's situation in both a regional and local context will be more effective than policies targeting only local, primary air pollutants.
- Article
(5666 KB) - Full-text XML
- Companion paper
-
Supplement
(14487 KB) - BibTeX
- EndNote
Outdoor air pollution has detrimental health effects (Pope and Dockery, 2006) and is responsible for more than 4 million deaths every year globally (Cohen et al., 2017), resulting in substantial global and regional decrements in life expectancy (Apte et al., 2018). India experiences high ambient air pollution, with an annual population-weighted PM2.5 (particulate matter with diameter less than 2.5 µm) mean of 74 µg m−3, and experiences the highest number of deaths from ambient air pollution among all countries in the world (∼1.1 million people yr−1, ∼1.5 years of life lost due to air pollution) (Cohen et al., 2017; Apte et al., 2018). Some of the most polluted cities in the world are in India. Delhi (population = 28 million) is the world's most polluted megacity, with recent annual-average PM2.5 concentrations of ∼140 µg m−3 (World Health Organization, 2018).
Previous aerosol characterization campaigns in Delhi have noted the importance of both primary and secondary sources to Delhi's poor ambient air quality (Jaiprakash et al., 2017; Pant et al., 2015). These studies have shown Delhi's PM to be rich in organics throughout the year and to contain high concentrations of inorganic species such as chloride and nitrate during the foggy wintertime. Furthermore, high concentrations of black carbon (BC) and brown carbon attributable to primary emissions such as biomass combustion and diesel exhaust have been observed across north India (Gupta et al., 2017; Satish et al., 2017; Bhat et al., 2017). However, previous studies in Delhi have mostly observed aerosol composition for short periods with limited temporal information (Pant et al., 2016). The Delhi Aerosol Supersite (DAS) study was designed to address current uncertainties in Delhi's aerosol composition by collecting continuous, highly time-resolved data on a long-term basis. In addition to providing insights into the atmospheric processes relevant for a polluted megacity, this study contributes to the understanding of the atmospheric science for South Asia in general. The lessons from Delhi have relevance for the entire Indo-Gangetic Plain (population: ∼400 million; including parts of India, Pakistan, Bangladesh, and Nepal) that experiences similar meteorology and high PM levels, especially during wintertime (Kumar et al., 2017; Singh et al., 2015).
Here we provide a detailed overview of the chemical composition of PM1 in Delhi by season and time of day based on a long-term deployment of a mass spectrometer instrument. We also provide insights into the role of meteorology in the concentration and composition of PM1. Finally, we include a brief overview of the source apportionment results from the positive matrix factorization (PMF) of aerosol mass spectra to understand the contribution of primary emissions and secondary processes to Delhi's PM concentrations, with details of the PMF provided in a companion paper (Bhandari et al., 2019).
2.1 Sampling site and pollutants measured
Delhi experiences a wide range of variation in temperature (T), relative humidity (RH), wind speeds, and precipitation across the year and by time of day (Fig. 1). The winters (December to mid-February) are cool (T∼10–20 ∘C, average diurnal range) and humid (RH ∼45 %–90 %) with low wind speeds (∼2–3 m s−1). Delhi frequently experiences shallow inversion layers (depth <100 m) during the winter, especially at night and in the morning hours. Summers (April to June) are very hot (T∼25–40 ∘C) and dry (RH ∼30 %–55 %). Delhi and most of the Indo-Gangetic Plain experiences episodic heavy rainfall during the monsoon (July to mid-September), accompanied by slightly lower temperatures (T∼25–35 ∘C) than the summers. While the winds throughout most of the year are predominately from the northwest, during the monsoon the wind is from the south during the nighttime. Spring (mid-February to March) and autumn (mid-September to November) are periods of transition between these meteorological extremes. For all seasons, the ventilation coefficient is highest during the daytime when the boundary layer height and the wind speeds reach their diurnal maxima. Changes in ventilation play an important role in the large seasonal and diurnal variation of PM (Trivedi et al., 2014). Unfavorable meteorological conditions often amplify primary emissions to produce spectacularly high PM2.5 concentrations (Guttikunda and Gurjar, 2012).
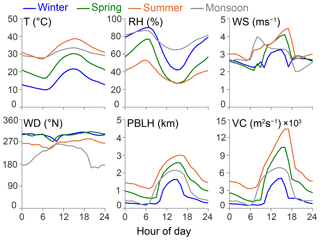
Figure 1Diurnal profiles of meteorological parameters (temperature, relative humidity, wind speed, wind direction, PBLH, and VC) by season. Average values by season and hour of day are presented for all parameters except wind direction. The median value is presented for wind direction. Ventilation coefficient (VC) = PBLH × wind speed.
To investigate the composition of ambient air in New Delhi at high time resolution, we installed a suite of online aerosol measurement instrumentation at the Indian Institute of Technology Delhi (IITD) campus in South Delhi. The instruments are situated in a temperature-controlled laboratory on the top floor of a four-story building. The nearest source of local emissions is an arterial road located 150 m away from the building. We measured chemical composition of nonrefractory PM1 (NR-PM1) was measured using an Aerodyne Aerosol Chemical Speciation Monitor (ACSM; Aerodyne Research, Billerica, MA). BC was measured using a multi-channel aethalometer (Magee Scientific Model AE33, Berkeley, CA) with a multi-spot sampling system designed to minimize the filter loading artifact present on earlier aethalometer systems (Drinovec et al., 2015). Particle size distributions (PSDs) were measured using a scanning mobility particle sizer (SMPS; TSI, Shoreview, MN), consisting of an electrostatic classifier (TSI model 3080), a differential mobility analyzer (DMA; TSI model 3081), an X-ray aerosol neutralizer (TSI model 3088), and a water-based condensation particle counter (CPC; TSI model 3785).
2.2 Instrumentation
The instruments were placed on two separate sampling lines. The first sampling line (SL1) had the ACSM and the SMPS in parallel. The second sampling line (SL2) was for the aethalometer. Both sampling lines had a PM2.5 cyclone at the inlet, followed by a water trap and a Nafion membrane diffusion dryer (Magee Scientific sample stream dryer, Berkeley, CA). The flow rate in SL1 was 3 L min−1, divided as follows: 1 L min−1 pulled by the SMPS, 0.1 L min−1 by the ACSM, and the remaining 1.9 L min−1 by an in-line flow controller which was in parallel with the SMPS and downstream from the ACSM. SL2 had a flow rate of 2 L min−1 pulled by the aethalometer. For the SMPS, the CPC pulled at a 1 L min−1 flow rate, and the electrostatic classifier was operated at a sheath flow rate of 4 L min−1 to enable SMPS scanning over a broad range of particle sizes. We conducted experiments at multiple sheath flow rates from 4 to 10 L min−1 and found the results to be consistent.
The ACSM measures NR-PM1, i.e., those compounds that flash vaporize at the heater temperature of ∼600 ∘C. The flash-vaporized compounds are subsequently ionized in the ACSM via 70 eV electron impact ionization and detected with a quadrupole mass spectrometer (Ng et al., 2011c). The scan speed was set at 200 ms amu−1 and pause setting at 125 for a sampling time (64 s). Detailed operational procedures for the ACSM are provided in Appendix A1. Some submicron aerosol constituents are refractory, including BC, metals, and crustal materials. For our core analyses of PM1 mass, we use the sum of NR-PM1 + BC as a composition-based proxy for total PM1, which we term “C-PM1”. This C-PM1 metric excludes the contribution to submicron mass of refractory metals and crustal materials, which we estimate results in a 5 %–10 % underestimate of total PM1 mass (see below).
2.3 Data processing
The SMPS scanned from 12 to 560 nm, with each subsequent scan 135 s apart. We used a mode fitting algorithm (Hussein et al., 2005) in the mass domain to estimate the PSD between 560 and 1000 nm. We validated the performance of our model by comparing the modeled and observed volume and number concentrations for the observed particle size range. We found that the model predicted the same volume as was observed (slope=1.00, R2=1.00) but slightly overestimated particle number concentrations (slope=1.06, R2=0.96), mostly for smaller particles. In order to develop a supplemental PSD-based estimate of submicron mass, we first estimated a complete (hybrid) PSD up to 1000 nm by combining the observed PSD from 12 to 560 nm and the modeled PSD from 560 to 1000 nm. Estimates of aerosol densities from Asia range between 1.3 and 1.6 g cm−3 (Sarangi et al., 2016; Hu et al., 2012). Using a particle density of 1.6 g cm−3 and the hybrid PSD, we developed a SMPS-based PM1 estimate (“SMPS-PM1”). On an hourly basis, the linear fit between our core C-PM1 and supplemental SMPS-PM1 estimates had a slope of 0.96 and an R2 of 0.85 (Fig. S1 in the Supplement). This linear fit suggested that our speciated PM1 data (NR-PM1 species and BC) agreed reasonably well with the SMPS-PM1 estimates. We used the PSD to estimate the transmission efficiency (TE) of the ACSM. The details of this correction along with other ACSM data processing steps are provided in Appendix A2. We estimate an overall uncertainty of up to 20 %–25 % in the ACSM data, which is within expectations for measurements from this instrument (Crenn et al., 2015).
While we acquired data for each instrument at high time resolution (∼1 min for the aethalometer and the ACSM; ∼2 min for the SMPS), for analytical simplicity we generally present the hourly averaged data for each instrument in this study. We categorize the seasons as winters (December to mid-February), summers (April to June), the monsoon (July to mid-September), and spring (mid-February to March) (Indian National Science Academy, 2018). Autumn (mid-September to November) is not included in our core analyses due to the unavailability of ACSM data for that period. In our analysis, we define day as 07:00–19:00 and night as 19:00–07:00.
We retrieved the hourly temperature and relative humidity (RH) data from the Indira Gandhi International Airport (IGIA; 8 km from our site). To obtain mesoscale (regional) meteorological data for wind speed, direction (10 m from ground), and planetary boundary layer height (PBLH) in Delhi, we used a NASA meteorological reanalysis dataset, MERRA2 (Gelaro et al., 2017). MERRA2 has a spatial resolution of (55 km × 60 km) and an hourly temporal resolution. We retrieved daily precipitation data for Delhi from the European Centre for Medium-Range Weather Forecasts' reanalysis dataset, ERA-Interim (Dee et al., 2011).
The hourly data for all species across the campaign are neither strictly normally nor log-normally distributed (Fig. S2). However, since the data are relatively closer to being log-normally distributed, we have included geometric mean (GM) and geometric standard deviation (GSD) in addition to the arithmetic mean (AM) wherever possible to provide a more complete representation of the central tendency of the data. Furthermore, the annual averages reported in this study are the averages of all the available hourly data from 2017 for the NR-PM1 species and BC. It should be noted that we do not have ACSM data (NR-PM1 species) for autumn and only a few days of aethalometer data (BC) for the monsoon. On the basis of available SMPS-PM1 data (our site) and PM2.5 data (multiple regulatory monitors in Delhi), we estimate that the true annual average differs from the data we collected by within ±20 %. As a sensitivity analysis, we reconstruct annual and campaign averages by giving equal weight to each 2-month period. For example, to calculate the synthetic (reconstructed) annual average for 2017, we averaged the averages of the six 2-month periods (January–February through November–December). In Table S1 we have provided a comparison between the AM, GM, and the synthetic averages of the PM1 components for the 2017 data against the entire campaign data.
3.1 Mass concentration
We observed marked seasonal and diurnal variation in the PM mass concentration owing to the interactions of sources, atmospheric mixing, and physicochemical processing. Figure 2 shows the time series of NR-PM1, individual submicron species, PM2.5 at a background site, and selected meteorological parameters. The daily average NR-PM1 concentrations at our site varied between 12.7 and 392 µg m−3, with an annual average of 87.3 µg m−3. Most C-PM1 mass was nonrefractory – the average NR-PM1 fraction of C-PM1 was highest in the winter (94 %) and lowest for warmer months (85 %) (Fig. 3). The average wintertime NR-PM1 concentration was ∼2 times higher than spring and ∼4 times higher than the warmer months. Using speciated mass concentrations and the PSD, we observed that C-PM1 was highly correlated with SMPS-PM1 (R2=0.83), and we achieved almost complete mass closure (Fig. S1). That most of the PM1 was composed of nonrefractory material and BC was consistent with past literature from Delhi which observed that metals and other nonrefractory crustal materials, which we did not measure in this study, constituted less than 5 % of PM1 (Jaiprakash et al., 2017).
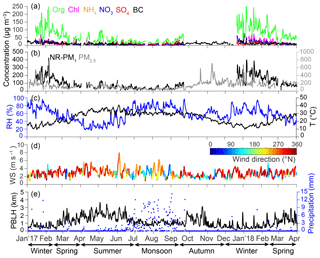
Figure 2Time series of (a) PM1 species (Org, Chl, NH4, NO3, SO4, and BC), (b) NR-PM1 and PM2.5 (DPCC, R.K. Puram – 3 km from our site), (c) relative humidity and temperature, (d) wind speed and direction, and (e) PBLH and precipitation. A 24 h moving average is applied on all time series presented.
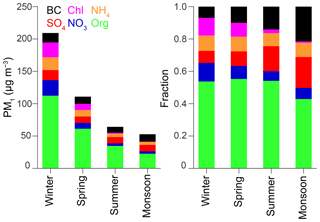
Figure 3Average absolute and fractional composition of PM1 (Org, Chl, NH4, NO3, SO4, and BC) by season. Limited BC data for the monsoon due to instrument downtime.
We estimated that the C-PM1 concentrations observed at our site were generally ∼85 % of the PM2.5 concentrations (R2=0.54 and slope=0.85 for linear fit of hourly C-PM1 and PM2.5 concentrations over entire campaign) measured at the nearest monitoring station that is operated by the Delhi Pollution Control Committee (DPCC), R.K. Puram (3 km away), where the annual average PM2.5 concentration for 2017 was 140 µg m−3. There were strong seasonal and diurnal variations in mass loadings, with winter being the most polluted with average concentration ∼4 times higher than the least polluted summer and monsoon months. The daily average PM2.5 concentration exceeded the daily average Indian National Ambient Air Quality Standards (NAAQS; 60 µg m−3) on more than 80 % of the days and the World Health Organization (WHO) 24 h average air quality guidelines (25 µg m−3) on all but two days. A distinct feature of Delhi's wintertime air pollution is the nearly complete absence of periods of clean air, in contrast to some other polluted megacities (e.g., Beijing), which are characterized by episodic alternation between clean and polluted conditions (Sun et al., 2013). For wintertime, the daily average PM2.5 concentrations exceeded 100 µg m−3 on 94 % of the days, the Indian NAAQS on 99 % of the days, and the WHO guidelines on all days. Daily-average PM2.5 concentrations at R.K. Puram exceeded 500 µg m−3 on four days in 2017.
3.2 PM1 composition: seasonal and diurnal variation
The concentrations and fractional contribution to PM1 of each species varied by season and time of day. Over the campaign, organics comprised 54 % of the submicron mass, inorganics (chloride, ammonium, nitrate, and sulfate) 36 %, and BC 10 %. There was a strong seasonality in C-PM1 loadings, with the wintertime average loadings exceeding the relatively less polluted and warmer summer and monsoon months by 3–4 times. We report the average seasonal concentrations of organics, sulfate, ammonium, nitrate, chloride, and BC in Table 1 and their contribution to C-PM1 in Fig. 3. Within each season there were distinct diurnal (time-of-day) trends for the average concentrations by hour of day for NR-PM1 and PM1 components (Fig. 4). These diurnal swings of the average hourly concentrations were the most prominent for the colder winter and spring months. In winter, average hourly NR-PM1 concentrations ranged between 97.4 and 254 µg m−3 (minimum and maximum concentrations for the average diurnal cycle). Spring conditions were moderately less polluted, with hourly average concentrations ranging diurnally from 37.0 to 167 µg m−3. The NR-PM1 concentrations varied much less during summer (range of concentrations for an average diurnal cycle: 38.7 to 72.4 µg m−3) and the monsoon (32.1 to 47.7 µg m−3). For most seasons, the hourly averaged NR-PM1 concentrations peaked around 07:00–08:00 and then again around 21:00–22:00, with the daily minimum typically occurring around 15:00–16:00. However, for the monsoon months the NR-PM1 average hourly concentrations were similar throughout the day. The diurnal variation in average hourly concentrations and fractional composition of NR-PM1 species for each season is presented in Fig. 5. The day and night averages by season for each PM1 species along with the summary averages of meteorological parameters are presented in Table 2. We did not observe any marked day of the week difference in the levels or composition of C-PM1 (Fig. S3).
Table 1Seasonal summary of PM1 species – arithmetic mean (AM), geometric mean (GM), and geometric standard deviation (GSD) for hourly concentrations.
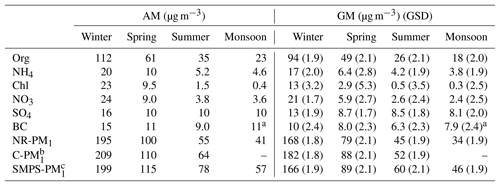
a Based on limited BC data for the monsoon due to instrument downtime. b Composition-based estimate of PM1 (BC + NR-PM1). c SMPS-based estimate using hybrid PSD and assuming a density of 1.6 g cm−3.
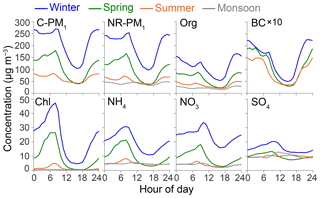
Figure 4Average diurnal profiles of PM1 species by season. Limited BC data for the monsoon due to instrument downtime. Composition-based estimate of PM1 (C-PM1) = BC + NR-PM1.
Table 2Day and night summary of PM1 species and meteorological parameters. Arithmetic mean used for all species and parameters, except wind direction for which we used median to estimate its central tendency.
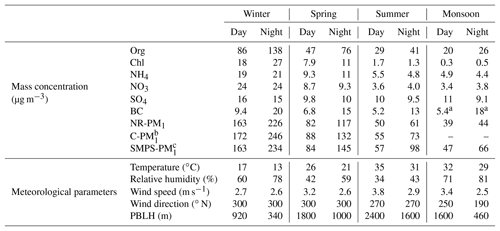
a Based on limited BC data for the monsoon due to instrument downtime. b Composition-based estimate of PM1 (BC + NR-PM1). c SMPS-based estimate using hybrid PSD and assuming a density of 1.6 g cm−3.
Organics were the single largest C-PM1 mass component for all seasons and at all times of the average diurnal cycle. Organics consistently contributed to more than ∼50 % of seasonal C-PM1 mass, with some episodes when their contribution was as high as 80 %. The high organic fraction of PM is consistent with studies from across the world (Zhang et al., 2007; Jimenez et al., 2009). The daily averages of organics at our site varied between 6.4 and 293 µg m−3, with an annual average of 51.5 µg m−3. The average wintertime organic concentration was ∼2 times higher than spring and ∼4–5 times higher than summer and the monsoon. While the wintertime organic concentrations ranged between 53.3 and 166 µg m−3, with the highest concentration during the night, the diurnal variations were less dynamic for the warmer months, with the hourly average organic concentrations ranging between 20.8 and 49.8 µg m−3 for summertime. Comparing daytime and nighttime f43 and f44 values for each season, the bulk organic aerosol was generally more oxidized during the warmer periods (Fig. S4), presumably owing to the higher photochemical activity during that time (Ng et al., 2011a).
Ammonium was the prominent inorganic cation in C-PM1 and generally balanced all the anionic inorganic species (chloride, nitrate, and sulfate). Over the campaign, the molar ratio of the inorganic anions to cations (ammonium) was 0.82 (R2=0.96). Ammonium mass concentrations were consistently around ∼10 % of the observed C-PM1. The daily average of ammonium at our site varied between 1.5 and 37.9 µg m−3, with an annual average of 9.0 µg m−3. The average ammonium concentration for wintertime was ∼2 times higher than spring and ∼4 times higher than summer and the monsoon. Ammonium concentration hourly averages ranged between 10.9 and 30.8 µg m−3 during the winter and between 4.2 and 8.3 µg m−3 during the summer.
We observed some of the highest chloride concentrations reported anywhere in the world, with episodes when hourly averages exceeded 100 µg m−3 (more than 40 such hours across the campaign). The 90th and 95th percentile values of the hourly concentrations of chloride over the campaign were 26.7 and 43.8 µg m−3, respectively. The daily average of chloride concentration at our site varied between 0.1 and 66.6 µg m−3, with an annual average of 6.1 µg m−3. Chloride concentrations showed the strongest seasonal variability, with the average wintertime concentration ∼2 times higher than during spring and more than 20 times higher than during the warm (summer and monsoon) months. During the cooler winter and spring months, chloride concentrations had the largest diurnal variation among all PM species observed, with the average diurnal minimum and maximum hourly concentration ranging between 4.6 and 47.3 µg m−3 in wintertime. The winter chloride peak is notable for its timing in the early morning hours (∼ 07:00), which is considerably later than the diurnal peak in organics and BC, which tended to occur shortly before midnight. While chloride contributed more than 10 % of the submicron mass in the winters, it comprised less than 2 % of the C-PM1 mass concentration during the summer and monsoon. Furthermore, chloride constituted around 12 %–16 % of NR-PM1 for temperatures below 15 ∘C but dropped to less than 4 % of the NR-PM1 concentrations for temperatures above 25 ∘C (Fig. S5). Given that ammonium was nearly always present in sufficient quantities to neutralize the major inorganic anions measured by the ACSM, we infer that the dominant fraction of chloride was usually present as ammonium chloride, for which gas–particle partitioning is strongly temperature dependent. (However, we cannot exclude the possibility that organic chlorides contributed a subsidiary fraction of the chloride mass.) Even for high episodic chloride concentrations, ammonium was present in sufficient levels to neutralize most of the anionic species, with a deficiency of only ∼20 % when considering only hours with chloride concentrations higher than the 90th percentile campaign value (26.7 µg m−3).
To understand whether the sharp drop in chloride concentrations for warmer times of day could be explained by evaporation of ammonium chloride, we used an inorganic aerosol thermodynamics model (Friese and Ebel, 2010). The detailed methodology and results of the model used are presented in Appendix B. Results from inorganic thermodynamic modeling suggest that most of the ammonium chloride observed in the winter is expected to evaporate at summer temperatures and relative humidity, consistent with our observations. The volatile nature of ammonium chloride has also been observed in other parts of the world (Salcedo et al., 2006; Wang et al., 2016) and was consistent with the sharp drop in the chloride fraction that we observed for the warmer periods. We believe that gas–particle partitioning and episodic sources (Sect. 3.4.1) may drive much of the diurnal and seasonal variation in particulate chloride. We would therefore expect a large fraction of chloride to be in the gas phase, especially for warmer periods. We did not collect gas-phase HCl measurements here, but future studies could validate this hypothesis through measurements of gas-phase chloride.
Nitrate comprised 6 %–12 % of Delhi's C-PM1, with daily averages between 0.6 and 58.5 µg m−3, with an annual average of 8.8 µg m−3. The average wintertime nitrate concentration was more than 2 times higher than spring and more than 6 times higher than summer and the monsoon. The average diurnal cycle (lowest and highest hourly average concentration) for wintertime concentrations ranged between 15.9 and 33.6 µg m−3, and the summer concentrations ranged between 2.2 and 6.3 µg m−3. The nitrate fraction of NR-PM1 dropped from 12 % at temperatures below 25 ∘C to 5 %–9 % at temperatures above 25 ∘C, likely due to the thermodynamics of ammonium nitrate. As with chloride, nitrate concentrations were also generally highly correlated with ammonium concentrations (R2=0.69 for hourly data over entire campaign), suggesting that most of the nitrate observed was present as ammonium nitrate. Considering the ubiquitous NOx sources in this megacity, organic nitrates may also contribute to the total nitrate measured by the ACSM.
The daily averages of sulfate at our sites varied between 3.1 and 34.5 µg m−3, with an annual average of 11.8 µg m−3. Sulfate had the least seasonal variability among the NR-PM1 species, with wintertime average concentration ∼1.5 times higher than each of the other seasons (spring, summer, and monsoon). In addition to the low seasonal variability, sulfate was also the chemical constituent with the least diurnal variation and had relatively higher daytime concentrations for the warmer summer months. The diurnal variation in sulfate concentration for the cooler months was similar to that of other PM1 species, with the average early morning concentrations for winter and spring almost 2 times higher than the daytime concentrations. Sulfate was the only NR-PM1 species that had a higher mass fraction during the warmer months, contributing 13 %–30 % to the C-PM1 mass in the warmer months, 8 %–20 % in spring, and 5 %–13 % in winter, with the mass fraction being highest during the daytime for all seasons. The sulfate fraction of NR-PM1 increased from less than 10 % for periods cooler than 25 ∘C to more than 25 % for periods above 35 ∘C. The increase in sulfate mass fraction for warmer periods can be explained by the lower diurnal and seasonal variability in its absolute concentration, possibly due to a combination of increased daytime photochemical formation rates for warmer months and sulfate being well mixed in the atmosphere because of its transport from longer distances (Verma et al., 2012).
BC contributed to 6.4 % of the C-PM1 mass concentration in the winter compared to 10 % in the spring and 14 % in the summer. We had limited monsoon data for BC. The daily average of BC at our site varied between 2.2 and 35.2 µg m−3, with an annual average of 12.4 µg m−3. The average wintertime BC concentration was ∼1.5 times higher than spring and summer. The seasonal differences in the absolute BC concentrations were not as high as any of the other PM1 species. One possible explanation for this result relates to the presence of nearby BC sources within Delhi, including the major ring road with truck traffic near our sampling site. These trucks are often restricted to only passing through Delhi at night (Guttikunda and Calori, 2013). It is plausible that these nearby primary emissions would be incompletely mixed into the boundary layer and are therefore relatively less affected by atmospheric mixing (Sect. 3.3 and 3.5). Accordingly, BC had sharp diurnal variability, with peak nocturnal BC concentrations typically ∼3–4 times higher than during midday hours, with peak concentrations occurring at a similar time to the temporal peak for organics (typically just before midnight).
3.3 Role of meteorology
The planetary boundary layer height (PBLH) had a strong seasonal variation with summer heights 2–4 times larger than those during the cooler months. The seasonal variability in the PBLH along with that in wind speed resulted in the ventilation coefficient (VC = PBLH × wind speed, sometimes referred to as normalized dilution rate) being 4–6 times slower for the wintertime compared to the summer. The VC is often used as a parameter to characterize the role of atmospheric dilution in pollutant concentrations, both in the Indian context (Vittal Murty et al., 1980) and globally (Marshall et al., 2005; Apte et al., 2012). Seasonal variability in the VC appears to reasonably agree with the higher NR-PM1 concentrations in less ventilated cold months and lower concentrations in the warmer months when the VC was higher (Fig. 6). The week with the lowest VC was ∼6 times less ventilated than the most ventilated week and had ∼6 times higher NR-PM1 mass concentrations. For the non-monsoon periods, the VC was generally a good indicator of NR-PM1 concentrations (R2=0.56 for weekly averaged data). For the cooler winter and spring months, the R2 for the linear fit of the weekly averaged VC and NR-PM1 concentrations was 0.79. The monsoon concentrations were lower than those that would be expected by the VC calculated for those periods. This result could be explained by a combination of change in the prominent nighttime wind direction from northwest to south during the monsoon and the washout of PM by the monsoon rain. For the monsoon period, we observed that the average NR-PM1 mass concentration was almost half on days when it rained compared to the dry (no rain) days with no change in the composition of NR-PM1. The strong modulating effect of meteorology on air pollution is well appreciated for Delhi and other Indian cities (Guttikunda and Gurjar, 2012; Tiwari et al., 2015; Sujatha et al., 2016).
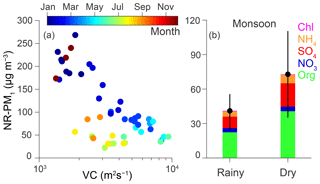
Figure 6(a) Variations of NR-PM1 mass concentrations as a function of ventilation coefficient. Each scatter point is a weekly average and is color coded by month. Note that July to mid-September is the monsoon season. (b) Average NR-PM1 composition for days with (rainy) and without rain (dry). The vertical lines are the 25th (bottom) and 75th (top) percentiles.
Even within each season, the VC showed large time-of-day variations, with highest hourly average values 5–10 times larger than the lowest. For each season, times of day with a lower VC had the highest NR-PM1 concentrations, and the concentrations decreased as the VC value increased (Fig. S7). The large diurnal range of the VC seemed to explain most of the variability in NR-PM1 concentrations by time of day for most seasons (; ; ). For the monsoon, the diurnal variability of most PM1 species was generally low, even though the VC varied by time of day, possibly due to precipitation washout of PM and a change in characteristic wind direction during the monsoon (as discussed above).
In general, the sharp variation in the VC by season and time of day appear to explain much of the variability in NR-PM1 concentrations. Furthermore, volatile species (e.g., ammonium chloride and ammonium nitrate) evaporate to the gas phase during warmer periods, further lowering the mass concentrations compared to the cooler periods. While there are seasonal differences in emissions from sources such as crop burning and local biomass burning for heat (Guttikunda and Calori, 2013), our analysis suggests that in addition to episodic sources, meteorology being unfavorable is an important factor for some of the high PM concentrations observed.
3.4 Episodic high concentrations
3.4.1 Chloride episodes and wind direction
Delhi experiences a prominently northwestern wind (Fig. 1). However, we observed that for brief periods during winter and spring when the wind was from the south, the peak chloride concentrations dropped from as high as 50–100 µg m−3 on one day to less than 10 µg m−3 on the next (Fig. 7). Furthermore, the highest decile of chloride concentrations in the campaign were mostly observed when the wind was from the northwest (Fig. 8). During winter mornings, when chloride concentrations were generally highest, the chloride fraction of C-PM1 was almost 2 times higher for periods with a northwestern wind compared to periods with wind from any other direction (Fig. S8). These findings suggest a large source of chloride in the northwest of Delhi. The high levels of chloride observed in Delhi are neither observed in other South Asian countries (Kim et al., 2015; Stone et al., 2010; Salam et al., 2003), nor in other parts of India (Gupta and Mandariya, 2013; Guttikunda et al., 2013; Gupta et al., 2007), suggesting that these extreme levels of chloride probably come from more than just the usual type of biomass and waste burning (Goetz et al., 2018), which is ubiquitous across South Asia (Streets et al., 2003). While filter-based studies can cause underreporting of volatile species such as ammonium chloride, the levels of chloride we observe in Delhi are much higher than those reported from studies in South Asia (outside Delhi) that use online aerosol instrumentation (Goetz et al., 2018; Chakraborty et al., 2015). Furthermore, the PMF factor for biomass burning organic aerosol of Bhandari et al. (2019) does not correlate with chloride. There are many industrial sites in the northwest of Delhi, including metal processing plants that use HCl for steel pickling (Jaiprakash et al., 2017). The fugitive HCl fumes from these industries along with the high ammonia in Delhi (Warner et al., 2017) could be a pathway for these high observed particulate chloride concentrations (Pio and Harrison, 1987). Other possible sources of HCl are from the combustion of polyvinyl chloride, coal, and biomass burning (Yudovich and Ketris, 2006; Lightowlers and Cape, 1988; Palmer, 1976). Our findings are based on measurement of particulate chloride and inorganic thermodynamic modeling and can be tested by future studies that measure both gas and particulate chloride.
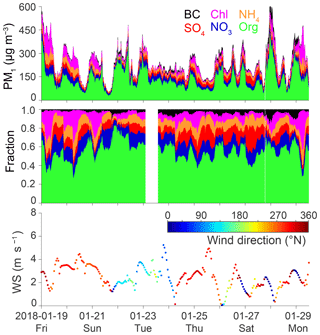
Figure 7Time series of PM1 species (Org, Chl, NH4, NO3, SO4, and BC) – stacked absolute concentrations and fraction of PM1 – along with wind speed and wind direction for a period with high chloride concentrations.
3.4.2 High organic episode
While organics contributed to almost half of the C-PM1 for all seasons and times of day, there were episodes for which the contribution of organics increased to as high as 80 % of the C-PM1. One such episode was around Lohri (13 January 2018), a festival celebrated in many parts of north India (including Delhi and regions upwind of Delhi), with bonfires burnt at night. In 2018, Lohri was on a weekend (Saturday), and we observed a sharp increase in nighttime C-PM1 concentrations, almost 2–3 times higher than the weekday nights preceding Lohri (Fig. 9). The contribution of both organics and BC increased for this period, with organics concentrations as high as 300 µg m−3 during these bonfire nights, contributing to ∼60 %–70 % of the C-PM1.
3.4.3 Autumn PM2.5 episodes
The PM2.5 concentrations in Delhi ramp up during the autumn, with some of the highest episodic concentrations observed during this period and often attributed to agricultural burning (Vijayakumar et al., 2016; Liu et al., 2018; Jethva et al., 2018). In 2017 the most polluted episodes were in the autumn, with the highest PM2.5 hourly concentrations exceeding 500 µg m−3 for 75–228 h across various locations in Delhi (DPCC monitoring stations and US Embassy). These autumn episodes constituted 80 %–100 % (across sites) of the hours for which PM2.5 exceeded 500 µg m−3 in 2017 across Delhi. The highest PM concentrations within autumn were observed during the periods with a relatively lower VC and when the wind was from the north or the northwest (Fig. 2). The concentrations were relatively lower for periods with higher VC values and when the wind was from the south. While some of these observations seem to support the role of agricultural burning in these episodic PM concentrations, we plan to strengthen this hypothesis in a future study using composition data that we have collected during the autumn of 2018.
3.5 Primary vs. secondary organic aerosol
Positive matrix factorization (PMF) conducted on the ACSM mass spectra provided further information on the sources and atmospheric processes that affect NR-PM1 concentrations in Delhi (Bhandari et al., 2019). The organic aerosol (OA) was separated into two factors: primary OA (POA) and oxygenated OA (OOA), with periods when the POA factor further separated into hydrocarbon-like OA (HOA) and biomass-burning OA (BBOA). POA exhibited strong diurnal variability, reflecting the impact of primary combustion emissions modulated by diurnal cycles in the PBLH. The POA fraction of organics was generally highest during the nighttime (∼50 % for winter and ∼40 % for summer) and lowest during the daytime (∼20 % for both winter and summer). As observed in other megacities, OOA was the largest constituent of the organic aerosol throughout the year (Jimenez et al., 2009), demonstrating the profound influence of secondary formation on particle concentrations in Delhi. OOA contributed to 50 %–80 % of the organics almost year-round (Fig. S9). We estimated primary particulate matter () and secondary particulate matter () following Sun et al. (2013). Since chloride was considered primary, and ammonium was generally highly correlated with chloride, we apportioned a chloride-equimolar amount of ammonium as primary and the remaining as secondary organic aerosol. In Fig. 11 we separate C-PM1 into PPM and SPM by season and time of day. We observed that almost 50 %–70 % of Delhi's C-PM1 was secondary in nature for the winter and spring months and up to 60 %–80 % for the warmer summer and monsoon months. Our results show that secondary aerosol accounts for the dominant fraction of Delhi's ambient NR-PM1 under most conditions. While our analyses do not provide direct evidence for the origin of the secondary fraction of PM1, consideration of typical advection timescales from the upwind boundaries of Delhi (∼2–3 h at typical wind speeds) suggests that a substantial fraction of Delhi's secondary aerosol may be transported from upwind regions, which also experience high PM mass loadings (Guttikunda and Goel, 2013). These findings suggest that improving Delhi's air quality will require a concerted effort at both at the local and the regional level. Future work could usefully apportion the composition of PM1 at receptor sites upwind of Delhi.
BC was found to be well correlated (R2=0.65) with HOA (for periods when HOA was a separate factor) (Bhandari et al., 2019), suggesting that traffic, diesel generators, and other liquid fossil fuel combustion contribute substantially to the BC inventory for Delhi. Furthermore, unlike chloride, the highest BC concentrations were uncorrelated with any particular wind direction (Fig. 8) and also showed less seasonal variation than other PM1 species (Fig. 4), potentially indicating a nearby year-round source that was less affected by atmospheric mixing. We suspect that trucks (and other diesel vehicles) were a major source of the high BC concentrations that we observed, similar to what has been observed in other urban environments in India (Latha et al., 2004). While BC absolute concentrations did not vary as much as other PM1 species, the fractional contribution to BC was as high as 20 % during periods when the C-PM1 was lower (Fig. 10). These findings indicate the large local nature of BC emissions and the potential to reduce BC concentrations by targeting high emitters such as heavy-duty trucks and diesel generation systems (Baidya and Borken-Kleefeld, 2009). Previous studies have shown that a small fraction (10 %–20 %) of high-emitting heavy-duty trucks contribute to almost half of the total BC emissions from heavy-duty trucks (Ban-Weiss et al., 2009).
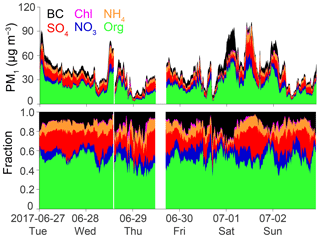
Figure 10Time series of PM1 species (Org, Chl, NH4, NO3, SO4, and BC) – stacked absolute concentrations and fraction of PM1 – for a relatively less polluted (warm) period.
We used continuous, highly time-resolved, and long-term data to provide a detailed seasonal and diurnal characterization of Delhi's PM1. We included data for organics, chloride, ammonium, nitrate, sulfate, and BC from January 2017 to April 2018. The submicron mass for each species varied dynamically by season and by time of day. Meteorology was found to be an important factor in the modulation of PM levels, specifically by change in the VC that varied dynamically as the PBLH varied by season and time of day. The PM levels were generally the highest during the cooler months and times of day, periods when the VC values were the lowest. Furthermore, concentrations of volatile species (e.g., ammonium chloride) were further enhanced during the cooler periods, when they had a higher tendency to be in the particle phase. While organics from biomass burning were enhanced during the cooler months, organics in general consistently (across seasons and times of day) contributed to ∼60 % of Delhi's PM1. We observed some of the highest chloride concentrations measured anywhere in the world, with average concentrations higher than 50 µg m−3 for periods during winter mornings when winds were from the northwest, resulting in part from what we suspect to be an industrial source.
We estimate that substantially more than half of Delhi's PM1 is of secondary origin. In combination with other evidence, including the high levels of remotely sensed PM2.5 observed across the upwind states of Haryana and Punjab (Dey et al., 2012; van Donkelaar et al., 2015), this finding points to the likely conclusion that the high pollution observed in Delhi is not merely a local problem but one with a widespread regional source as well. Accordingly, reducing the PM levels in Delhi will require both a local and a regional effort, with benefits that will be felt across the Indo-Gangetic Plain. At the same time, primary PM1 levels in Delhi are extremely high in absolute mass terms and are likely driven principally by local emissions within the Delhi National Capital Region. Delhi's air pollution has many critical sources; some are local, and some are regional (Chowdhury et al., 2007; Guttikunda and Goel, 2013; Health Effects Institute, 2018). Coordinated regional and local control of nearly all contributors will be required to bring about the order-of-magnitude concentration reductions that will ultimately make the air safe to breathe (Kumar et al., 2013, 2015; Bhanarkar et al., 2018).
Long-term monitoring campaigns such as the DAS can contribute previously unavailable information on the evolving role of sources and other processes that govern air pollution in Indian cities. In particular, continuous, highly time-resolved data provide a basis for evaluating the intended and unintended impact of policies and natural events on Delhi's air quality in near-real time. However, air pollution is spatially variable, and a single site generally does not provide sufficient information for the complete assessment of air quality in a large urban area like Delhi. Future work could usefully expand on this study through coordinated measurements of aerosol chemical composition at other locations. One key research need is to conduct similar measurements at sites upwind and downwind of Delhi to help quantify the role of local and regional sources in driving Delhi's air pollution more precisely. Long-term studies of the changing nature of air pollution in South Asian cities can help inform much-needed efforts to protect a large part of the world's population from the adverse effects of poor air quality.
Hourly concentrations for PM1 species used in this study are available via the Texas Data Repository, https://doi.org/10.18738/T8/9L33CI (Gani et al., 2019).
A1 ACSM: calibration and operational procedures
Lens alignment and flow calibration were conducted at the start of the campaign. Ionizer tuning, quadrupole resolution adjustment, adjustment of multiplier voltage, and m∕z calibration were conducted bimonthly. The pinhole was cleaned at least biweekly. Calibrations for the response factor (RF) of nitrate and the relative ionization efficiencies (RIEs) of ammonium and sulfate were conducted several times throughout the campaign (Table S2). For the RF and RIE calibrations, 300 nm particles, generated from 5 mM solutions of ammonium nitrate and ammonium sulfate, were injected simultaneously into the ACSM and CPC. The size-selected particles were sampled in jump mode (for all calibrations) as well as single scan mode (September 2017 and January 2018), which is now the recommended procedure for this calibration. The RF∕N2 air beam ratio was consistent in all jump mode calibrations, suggesting a consistent sensitivity of the instrument. Thus, the RF and RIE values from the two single scan mode calibrations were used for all data (one value up to September 2017 and another value for the data post September 2017).
A2 ACSM: data processing
Time-dependent air beam corrections were applied to the raw data based on N2 signal changes relative to the reference N2 signal (when the calibration was performed). Relative ion transmission (RIT) correction was applied using the default RIT curve (not the measured RIT curve) because of the occurrence of a low naphthalene signal due to high concentration of m∕z fragments in sampling that build up and desorb during the filter sampling period (Philip Croteau, Aerodyne Research, personal communication, 2017). Detection limits were applied to species concentrations (Ng et al., 2011c), and data below the detection limit were replaced with 0.5 times the detection limit. Collection efficiency (CE) was applied to account for inefficient aerosol collection due to effects such as particle bounce at the vaporizer. A composition-dependent CE was calculated based on the method described in Middlebrook et al. (2012). An inline Nafion dryer lowered RH levels to well within 50 % (<80 %), and the ammonium nitrate fraction was less than 40 % throughout the campaign. Accordingly, we only applied the acidity-dependent CE. This method assumes that the particles are internally mixed, and hence a single correction factor was applied for all species.
To account for particle loss during transmission through the aerodynamic lens, a transmission efficiency (TE) correction factor was computed using hourly averaged SMPS data. The following method was used to compute the TE correction factor.
Hourly particle density was computed using hourly averaged ACSM composition (DeCarlo et al., 2004). Ammonium was attributed to each of the other inorganic species, assuming that ammonium would first neutralize sulfate, followed by nitrate and then chloride (Du et al., 2010). The tracer-based method was used to compute average organics composition and density (Ng et al., 2011b; Cross et al., 2007; Kuwata et al., 2012). Mobility diameter was converted to vacuum aerodynamic diameter (Dva) using the method described in DeCarlo et al. (2004), by assuming the Jayne shape factor to be 1 and using the calculated density. The averaged experimental TE curve of an aerodynamic focusing lens system (Liu et al., 2007) was applied to the particle size distribution, and the TE correction factor was calculated as the ratio of total particle volume to the volume after applying the TE curve. Finally, average TE factors were computed for every hour of the day for every season (Fig. S10), and the ACSM concentrations were multiplied by this correction factor.
The Extended Aerosol Thermodynamics Model (E-AIM) is used for interpreting the effect of gas–particle partitioning on the seasonality of concentrations (Friese and Ebel, 2010). The focus of this modeling is on inorganic species concentrations. While E-AIM can account for organic–inorganic interactions, since the identity of organic-phase compounds is unknown, these interactions are ignored. Further, model IV of E-AIM is employed as it permits the variation of temperature and RH in the presence of the chloride anion. However, there are at least two limitations to the approach:
-
The model always requires that the charge balance be maintained, although charged aerosols have been previously reported in literature. Further, the model does not provide a route to account for periods with excess cations; no additional anions are available in the model. Na+, the only additional cation available and used as a surrogate for metal cations not measured in this study, is used to balance the charge for periods with excess anions.
-
Periods with RH less than 60 % cannot be run in the presence of chloride. To deal with this, RH for all such periods is set to 60 %.
Due to data limitations and the above conditions, only periods between 00:00 and 03:00 and between 11:00 and 24:00 are analyzed. Hourly averaged diurnal NR-PM1 species concentrations and gas-phase NH3 concentrations (obtained when available from the nearest central regulatory monitoring stations) for the winter of 2017 are input into the model. This technique of running the model has been recently validated considering newly discovered issues in such thermodynamic models (Song et al., 2018; Murphy et al., 2017). The model is run in two modes – a “constrained” and an “unconstrained” mode. In the first run, diurnal data for the winter of 2017 are input, together with actual temperature and constrained RH; this mode forces the model to prevent gas–aerosol partitioning of the input data and instead generate equilibrium concentrations of gas-phase species HCl and HNO3. Together with the measured NR-PM1 speciated concentrations and NH3, these concentrations are used to obtain total concentration estimates for NH3 (), NO3 (), Cl (), and H+ (HCl+HNO3). Other species are non-volatile, and their particle-phase concentrations are their total concentrations. The obtained actual concentrations corrected for VC effects are run with the temperature and RH of summer 2017. Thus, to estimate maximum PM formation potential relative to the sources in winter 2017, diurnal “source” concentration averages for winter 2017 are applied to summer. We run the model in an unconstrained mode – the goal being to allow repartitioning for achieving equilibrium.
For the winter of 2017, chloride and nitrate were almost completely in aerosol phase except between 12:00 and 17:00 (for analyzed periods >55 % of chloride and >85 % of nitrate in particle phase). Applying winter 2017 source strength to summer, we observe a significant shift – maximum nitrate in particle phase never exceeds 40 % (10 µg m−3), and chloride never exceeds 10 % (3.5 µg m−3). Thus, temperature and RH can explain the dramatic drop in concentrations of particle-phase chloride and nitrate.
The supplement related to this article is available online at: https://doi.org/10.5194/acp-19-6843-2019-supplement.
JSA, LHR, GH, SG, and SB designed the study. SG, SB, PS, ZA, and SS carried out the data collection. SG, SB, KP, and SS carried out the data processing and analysis. SG, SB, KP, DSW, LHR, and JSA assisted with the interpretation of results. All co-authors contributed to the writing and reviewing of the paper.
The authors declare that they have no conflict of interest.
Joshua S. Apte was supported by the Climate Works Foundation. We are thankful to the Indian Institute of Technology Delhi (IITD) for institutional support. We are grateful to all student and staff members of the Aerosol Research Characterization laboratory (especially Nisar Ali Baig and Mohammad Yawar) and the Environmental Engineering laboratory (especially Sanjay Gupta) at IITD for their constant support. We are thankful to Philip Croteau (Aerodyne Research) and Maynard Havlicek (TSI) for always providing timely technical support for the instrumentation.
This paper was edited by Alex Lee and reviewed by three anonymous referees.
Apte, J. S., Bombrun, E., Marshall, J. D., and Nazaroff, W. W.: Global intraurban intake fractions for primary air pollutants from vehicles and other distributed sources, Environ. Sci. Technol., 46, 3415–3423, https://doi.org/10.1021/es204021h, 2012. a
Apte, J. S., Brauer, M., Cohen, A. J., Ezzati, M., and Pope, C. A.: Ambient PM2.5 reduces global and regional life expectancy, Environ. Sci. Technol. Lett., 5, 546–551, https://doi.org/10.1021/acs.estlett.8b00360, 2018. a, b
Baidya, S. and Borken-Kleefeld, J.: Atmospheric emissions from road transportation in India, Energ. Policy, 37, 3812–3822, https://doi.org/10.1016/j.enpol.2009.07.010, 2009. a
Ban-Weiss, G. A., Lunden, M. M., Kirchstetter, T. W., and Harley, R. A.: Measurement of black carbon and particle number emission factors from individual heavy-duty trucks, Environ. Sci. Technol., 43, 1419–1424, https://doi.org/10.1021/es8021039, 2009. a
Bhanarkar, A. D., Purohit, P., Rafaj, P., Amann, M., Bertok, I., Cofala, J., Rao, P. S., Vardhan, B., Kiesewetter, G., Sander, R., Schöpp, W., Majumdar, D., Srivastava, A., Deshmukh, S., Kawarti, A., and Kumar, R.: Managing future air quality in megacities: Co-benefit assessment for Delhi, Atmos. Environ., 186, 158–177, https://doi.org/10.1016/j.atmosenv.2018.05.026, 2018. a
Bhandari, S., Gani, S., Patel, K., Wang, D. S., Soni, P., Arub, Z., Habib, G., Apte, J. S., and Hildebrandt Ruiz, L.: Sources and atmospheric dynamics of organic aerosol in New Delhi, India: Insights from receptor modeling, submitted to Atmospheric Chemistry and Physics Discussions, also available as preprint: https://doi.org/10.1002/essoar.10500949.1, 2019. a, b, c, d
Bhat, M. A., Romshoo, S. A., and Beig, G.: Aerosol black carbon at an urban site-Srinagar, Northwestern Himalaya, India: Seasonality, sources, meteorology and radiative forcing, Atmos. Environ., 165, 336–348, https://doi.org/10.1016/j.atmosenv.2017.07.004, 2017. a
Chakraborty, A., Bhattu, D., Gupta, T., Tripathi, S. N., and Canagaratna, M. R.: Real-time measurements of ambient aerosols in a polluted Indian city: Sources, characteristics, and processing of organic aerosols during foggy and nonfoggy periods, J. Geophys. Res.-Atmos., 120, 9006–9019, https://doi.org/10.1002/2015JD023419, 2015. a
Chowdhury, Z., Zheng, M., Schauer, J. J., Sheesley, R. J., Salmon, L. G., Cass, G. R., and Russell, A. G.: Speciation of ambient fine organic carbon particles and source apportionment of PM2.5 in Indian cities, J. Geophys. Res.-Atmos., 112, D15305, https://doi.org/10.1029/2007JD008386, 2007. a
Cohen, A. J., Brauer, M., Burnett, R., Anderson, H. R., Frostad, J., Estep, K., Balakrishnan, K., Brunekreef, B., Dandona, L., Dandona, R., Feigin, V., Freedman, G., Hubbell, B., Jobling, A., Kan, H., Knibbs, L., Liu, Y., Martin, R., Morawska, L., Pope, C. A., Shin, H., Straif, K., Shaddick, G., Thomas, M., van Dingenen, R., van Donkelaar, A., Vos, T., Murray, C. J. L., and Forouzanfar, M. H.: Estimates and 25-year trends of the global burden of disease attributable to ambient air pollution: An analysis of data from the Global Burden of Diseases Study 2015, Lancet, 389, 1907–1918, https://doi.org/10.1016/S0140-6736(17)30505-6, 2017. a, b
Crenn, V., Sciare, J., Croteau, P. L., Verlhac, S., Fröhlich, R., Belis, C. A., Aas, W., Äijälä, M., Alastuey, A., Artiñano, B., Baisnée, D., Bonnaire, N., Bressi, M., Canagaratna, M., Canonaco, F., Carbone, C., Cavalli, F., Coz, E., Cubison, M. J., Esser-Gietl, J. K., Green, D. C., Gros, V., Heikkinen, L., Herrmann, H., Lunder, C., Minguillón, M. C., Mocnik, G., O'Dowd, C. D., Ovadnevaite, J., Petit, J.-E., Petralia, E., Poulain, L., Priestman, M., Riffault, V., Ripoll, A., Sarda-Estève, R., Slowik, J. G., Setyan, A., Wiedensohler, A., Baltensperger, U., Prévôt, A. S. H., Jayne, J. T., and Favez, O.: ACTRIS ACSM intercomparison – Part 1: Reproducibility of concentration and fragment results from 13 individual Quadrupole Aerosol Chemical Speciation Monitors (Q-ACSM) and consistency with co-located instruments, Atmos. Meas. Tech., 8, 5063–5087, https://doi.org/10.5194/amt-8-5063-2015, 2015. a
Cross, E. S., Slowik, J. G., Davidovits, P., Allan, J. D., Worsnop, D. R., Jayne, J. T., Lewis, D. K., Canagaratna, M., and Onasch, T. B.: Laboratory and ambient particle density determinations using light scattering in conjunction with aerosol mass spectrometry, Aerosol Sci. Tech., 41, 343–359, https://doi.org/10.1080/02786820701199736, 2007. a
DeCarlo, P. F., Slowik, J. G., Worsnop, D. R., Davidovits, P., and Jimenez, J. L.: Particle morphology and density characterization by combined mobility and aerodynamic diameter measurements. Part 1: Theory, Aerosol Sci. Tech., 38, 1185–1205, https://doi.org/10.1080/027868290903907, 2004. a, b
Dee, D. P., Uppala, S. M., Simmons, A. J., Berrisford, P., Poli, P., Kobayashi, S., Andrae, U., Balmaseda, M. A., Balsamo, G., Bauer, P., Bechtold, P., Beljaars, A. C. M., van de Berg, L., Bidlot, J., Bormann, N., Delsol, C., Dragani, R., Fuentes, M., Geer, A. J., Haimberger, L., Healy, S. B., Hersbach, H., Hólm, E. V., Isaksen, L., Kållberg, P., Köhler, M., Matricardi, M., McNally, A. P., Monge-Sanz, B. M., Morcrette, J.-J., Park, B.-K., Peubey, C., de Rosnay, P., Tavolato, C., Thépaut, J.-N., and Vitart, F.: The ERA-Interim reanalysis: Configuration and performance of the data assimilation system, Q. J. Roy. Meteor. Soc., 137, 553–597, https://doi.org/10.1002/qj.828, 2011. a
Dey, S., Girolamo, L. D., van Donkelaar, A., Tripathi, S., Gupta, T., and Mohan, M.: Variability of outdoor fine particulate (PM2.5) concentration in the Indian Subcontinent: A remote sensing approach, Remote Sens. Environ., 127, 153–161, https://doi.org/10.1016/j.rse.2012.08.021, 2012. a
Drinovec, L., Mocnik, G., Zotter, P., Prévôt, A. S. H., Ruckstuhl, C., Coz, E., Rupakheti, M., Sciare, J., Müller, T., Wiedensohler, A., and Hansen, A. D. A.: The “dual-spot” Aethalometer: an improved measurement of aerosol black carbon with real-time loading compensation, Atmos. Meas. Tech., 8, 1965–1979, https://doi.org/10.5194/amt-8-1965-2015, 2015. a
Du, H., Kong, L., Cheng, T., Chen, J., Yang, X., Zhang, R., Han, Z., Yan, Z., and Ma, Y.: Insights into ammonium particle-to-gas conversion: Non-sulfate ammonium coupling with nitrate and chloride, Aerosol Air Qual. Res., 10, 589–595, https://doi.org/10.4209/aaqr.2010.04.0034, 2010. a
Friese, E. and Ebel, A.: Temperature dependent thermodynamic model of the system , J. Phys. Chem. A, 114, 11595–11631, https://doi.org/10.1021/jp101041j, 2010. a, b
Gani, S., Bhandari, S., Seraj, S., Wang, D. S., Patel, K., Soni, P., Arub, Z., Habib, G., Hildebrandt Ruiz, L., and Apte, J.: Submicron aerosol composition in the world's most polluted megacity: The Delhi Aerosol Supersite campaign, Texas Data Repository Dataverse, V1, https://doi.org/10.18738/T8/9L33CI, 2019. a
Gelaro, R., McCarty, W., Suárez, M. J., Todling, R., Molod, A., Takacs, L., Randles, C. A., Darmenov, A., Bosilovich, M. G., Reichle, R., Wargan, K., Coy, L., Cullather, R., Draper, C., Akella, S., Buchard, V., Conaty, A., da Silva, A. M., Gu, W., Kim, G.-K., Koster, R., Lucchesi, R., Merkova, D., Nielsen, J. E., Partyka, G., Pawson, S., Putman, W., Rienecker, M., Schubert, S. D., Sienkiewicz, M., and Zhao, B.: The Modern-Era Retrospective Analysis for Research and Applications, Version 2 (MERRA-2), J. Climate, 30, 5419–5454, https://doi.org/10.1175/JCLI-D-16-0758.1, 2017. a
Goetz, J. D., Giordano, M. R., Stockwell, C. E., Christian, T. J., Maharjan, R., Adhikari, S., Bhave, P. V., Praveen, P. S., Panday, A. K., Jayarathne, T., Stone, E. A., Yokelson, R. J., and DeCarlo, P. F.: Speciated online PM1 from South Asian combustion sources – Part 1: Fuel-based emission factors and size distributions, Atmos. Chem. Phys., 18, 14653–14679, https://doi.org/10.5194/acp-18-14653-2018, 2018. a, b
Gupta, A., Karar, K., and Srivastava, A.: Chemical mass balance source apportionment of PM10 and TSP in residential and industrial sites of an urban region of Kolkata, India, J. Hazard. Mater., 142, 279–287, https://doi.org/10.1016/j.jhazmat.2006.08.013, 2007. a
Gupta, P., Singh, S. P., Jangid, A., and Kumar, R.: Characterization of black carbon in the ambient air of Agra, India: Seasonal variation and meteorological influence, Adv. Atmos. Sci., 34, 1082–1094, https://doi.org/10.1007/s00376-017-6234-z, 2017. a
Gupta, T. and Mandariya, A.: Sources of submicron aerosol during fog-dominated wintertime at Kanpur, Environ. Sci. Pollut. R., 20, 5615–5629, https://doi.org/10.1007/s11356-013-1580-6, 2013. a
Guttikunda, S. K. and Calori, G.: A GIS based emissions inventory at 1 km × 1 km spatial resolution for air pollution analysis in Delhi, India, Atmos. Environ., 67, 101–111, https://doi.org/10.1016/j.atmosenv.2012.10.040, 2013. a, b
Guttikunda, S. K. and Goel, R.: Health impacts of particulate pollution in a megacity – Delhi, India, Environmental Development, 6, 8–20, https://doi.org/10.1016/j.envdev.2012.12.002, 2013. a, b
Guttikunda, S. K. and Gurjar, B. R.: Role of meteorology in seasonality of air pollution in megacity Delhi, India, Environ. Monit. Assess., 184, 3199–3211, https://doi.org/10.1007/s10661-011-2182-8, 2012. a, b
Guttikunda, S. K., Kopakka, R. V., Dasari, P., and Gertler, A. W.: Receptor model-based source apportionment of particulate pollution in Hyderabad, India, Environ. Monit. Assess., 185, 5585–5593, https://doi.org/10.1007/s10661-012-2969-2, 2013. a
Health Effects Institute: Burden of disease attributable to major air pollution sources in India, available at: https://www.healtheffects.org/publication/gbd-air-pollution-india, last access: 17 September 2018. a
Hu, M., Peng, J., Sun, K., Yue, D., Guo, S., Wiedensohler, A., and Wu, Z.: Estimation of size-resolved ambient particle density based on the measurement of aerosol number, mass, and chemical size distributions in the winter in Beijing, Environ. Sci. Technol., 46, 9941–9947, https://doi.org/10.1021/es204073t, 2012. a
Hussein, T., Dal Maso, M., Petäjä, T., Koponen, I. K., Paatero, P., Aalto, P. P., Hämeri, K., and Kulmala, M.: Evaluation of an automatic algorithm for fitting the particle number size distributions, Boreal Environ. Res., 10, 337–355, 2005. a
Indian National Science Academy: Seasons of Delhi, available at: https://www.insaindia.res.in/climate.php, last access: 20 August 2018. a
Jaiprakash, Singhai, A., Habib, G., Raman, R. S., and Gupta, T.: Chemical characterization of PM1 aerosol in Delhi and source apportionment using positive matrix factorization, Environ. Sci. Pollut. Res., 24, 445–462, https://doi.org/10.1007/s11356-016-7708-8, 2017. a, b, c
Jethva, H., Chand, D., Torres, O., Gupta, P., Lyapustin, A., and Patadia, F.: Agricultural burning and air quality over Northern India: A aynergistic analysis using NASA's A-train satellite data and ground measurements, Aerosol Air Qual. Res., 18, 1756–1773, https://doi.org/10.4209/aaqr.2017.12.0583, 2018. a
Jimenez, J. L., Canagaratna, M. R., Donahue, N. M., Prevot, A. S. H., Zhang, Q., Kroll, J. H., DeCarlo, P. F., Allan, J. D., Coe, H., Ng, N. L., Aiken, A. C., Docherty, K. S., Ulbrich, I. M., Grieshop, A. P., Robinson, A. L., Duplissy, J., Smith, J. D., Wilson, K. R., Lanz, V. A., Hueglin, C., Sun, Y. L., Tian, J., Laaksonen, A., Raatikainen, T., Rautiainen, J., Vaattovaara, P., Ehn, M., Kulmala, M., Tomlinson, J. M., Collins, D. R., Cubison, M. J., Dunlea, J., Huffman, J. A., Onasch, T. B., Alfarra, M. R., Williams, P. I., Bower, K., Kondo, Y., Schneider, J., Drewnick, F., Borrmann, S., Weimer, S., Demerjian, K., Salcedo, D., Cottrell, L., Griffin, R., Takami, A., Miyoshi, T., Hatakeyama, S., Shimono, A., Sun, J. Y., Zhang, Y. M., Dzepina, K., Kimmel, J. R., Sueper, D., Jayne, J. T., Herndon, S. C., Trimborn, A. M., Williams, L. R., Wood, E. C., Middlebrook, A. M., Kolb, C. E., Baltensperger, U., and Worsnop, D. R.: Evolution of organic aerosols in the atmosphere, Science, 326, 1525–1529, https://doi.org/10.1126/science.1180353, 2009. a, b
Kim, B. M., Park, J.-S., Kim, S.-W., Kim, H., Jeon, H., Cho, C., Kim, J.-H., Hong, S., Rupakheti, M., Panday, A. K., Park, R. J., Hong, J., and Yoon, S.-C.: Source apportionment of PM10 mass and particulate carbon in the Kathmandu Valley, Nepal, Atmos. Environ., 123, 190–199, https://doi.org/10.1016/j.atmosenv.2015.10.082, 2015. a
Kumar, M., Raju, M. P., Singh, R., Singh, A., Singh, R. S., and Banerjee, T.: Wintertime characteristics of aerosols over middle Indo-Gangetic Plain: Vertical profile, transport and radiative forcing, Atmos. Res., 183, 268–282, https://doi.org/10.1016/j.atmosres.2016.09.012, 2017. a
Kumar, P., Jain, S., Gurjar, B., Sharma, P., Khare, M., Morawska, L., and Britter, R.: New Directions: Can a “blue sky” return to Indian megacities?, Atmos. Environ., 71, 198–201, https://doi.org/10.1016/j.atmosenv.2013.01.055, 2013. a
Kumar, P., Khare, M., Harrison, R. M., Bloss, W. J., Lewis, A. C., Coe, H., and Morawska, L.: New directions: Air pollution challenges for developing megacities like Delhi, Atmos. Environ., 122, 657–661, https://doi.org/10.1016/j.atmosenv.2015.10.032, 2015. a
Kuwata, M., Zorn, S. R., and Martin, S. T.: Using elemental ratios to predict the density of organic material composed of carbon, hydrogen, and oxygen, Environ. Sci. Technol., 46, 787–794, https://doi.org/10.1021/es202525q, 2012. a
Latha, K. M., Badrinath, K., and Moorthy, K.: Impact of diesel vehicular emissions on ambient black carbon concentration at an urban location in India, Curr. Sci. India, 86, 451–453, 2004. a
Lightowlers, P. and Cape, J.: Sources and fate of atmospheric HCl in the U.K. and Western Europe, Atmos. Environ., 22, 7–15, https://doi.org/10.1016/0004-6981(88)90294-6, 1988. a
Liu, P. S. K., Deng, R., Smith, K. A., Williams, L. R., Jayne, J. T., Canagaratna, M. R., Moore, K., Onasch, T. B., Worsnop, D. R., and Deshler, T.: Transmission efficiency of an aerodynamic focusing lens system: Comparison of model calculations and laboratory measurements for the Aerodyne aerosol mass spectrometer, Aerosol Sci. Tech., 41, 721–733, https://doi.org/10.1080/02786820701422278, 2007. a
Liu, T., Marlier, M. E., DeFries, R. S., Westervelt, D. M., Xia, K. R., Fiore, A. M., Mickley, L. J., Cusworth, D. H., and Milly, G.: Seasonal impact of regional outdoor biomass burning on air pollution in three Indian cities: Delhi, Bengaluru, and Pune, Atmos. Environ., 172, 83–92, https://doi.org/10.1016/j.atmosenv.2017.10.024, 2018. a
Marshall, J. D., Teoh, S.-K., and Nazaroff, W. W.: Intake fraction of nonreactive vehicle emissions in US urban areas, Atmos. Environ., 39, 1363–1371, https://doi.org/10.1016/j.atmosenv.2004.11.008, 2005. a
Middlebrook, A. M., Bahreini, R., Jimenez, J. L., and Canagaratna, M. R.: Evaluation of composition-dependent collection efficiencies for the Aerodyne aerosol mass spectrometer using field data, Aerosol Sci. Tech., 46, 258–271, https://doi.org/10.1080/02786826.2011.620041, 2012. a
Murphy, G. J., Gregoire, P., Tevlin, A., Wentworth, G., Ellis, R., Markovic, M., and VandenBoer, T.: Observational constraints on particle acidity using measurements and modelling of particles and gases, Faraday Discuss., 200, 379–395, https://doi.org/10.1039/C7FD00086C, 2017. a
Ng, N. L., Canagaratna, M. R., Jimenez, J. L., Chhabra, P. S., Seinfeld, J. H., and Worsnop, D. R.: Changes in organic aerosol composition with aging inferred from aerosol mass spectra, Atmos. Chem. Phys., 11, 6465–6474, https://doi.org/10.5194/acp-11-6465-2011, 2011a. a
Ng, N. L., Canagaratna, M. R., Jimenez, J. L., Zhang, Q., Ulbrich, I. M., and Worsnop, D. R.: Real-time methods for estimating organic component mass concentrations from aerosol mass spectrometer data, Environ. Sci. Technol., 45, 910–916, https://doi.org/10.1021/es102951k, 2011b. a
Ng, N. L., Herndon, S. C., Trimborn, A., Canagaratna, M. R., Croteau, P. L., Onasch, T. B., Sueper, D., Worsnop, D. R., Zhang, Q., Sun, Y. L., and Jayne, J. T.: An Aerosol Chemical Speciation Monitor (ACSM) for routine monitoring of the composition and mass concentrations of ambient aerosol, Aerosol Sci. Tech., 45, 780–794, https://doi.org/10.1080/02786826.2011.560211, 2011c. a, b
Palmer, T. Y.: Combustion sources of atmospheric chlorine, Nature, 263, 44–46, https://doi.org/10.1038/263044a0, 1976. a
Pant, P., Shukla, A., Kohl, S. D., Chow, J. C., Watson, J. G., and Harrison, R. M.: Characterization of ambient PM2.5 at a pollution hotspot in New Delhi, India and inference of sources, Atmos. Environ., 109, 178–189, https://doi.org/10.1016/j.atmosenv.2015.02.074, 2015. a
Pant, P., Guttikunda, S. K., and Peltier, R. E.: Exposure to particulate matter in India: A synthesis of findings and future directions, Environ. Res., 147, 480–496, https://doi.org/10.1016/j.envres.2016.03.011, 2016. a
Pio, C. A. and Harrison, R. M.: The equilibrium of ammonium chloride aerosol with gaseous hydrochloric acid and ammonia under tropospheric conditions, Atmos. Environ., 21, 1243–1246, https://doi.org/10.1016/0004-6981(87)90253-8, 1987. a
Pope, C. A. and Dockery, D. W.: Health effects of fine particulate air pollution: Lines that connect, J. Air Waste Manage., 56, 709–742, https://doi.org/10.1080/10473289.2006.10464485, 2006. a
Salam, A., Bauer, H., Kassin, K., Ullah, S. M., and Puxbaum, H.: Aerosol chemical characteristics of a mega-city in Southeast Asia (Dhaka – Bangladesh), Atmos. Environ., 37, 2517–2528, https://doi.org/10.1016/S1352-2310(03)00135-3, 2003. a
Salcedo, D., Onasch, T. B., Dzepina, K., Canagaratna, M. R., Zhang, Q., Huffman, J. A., DeCarlo, P. F., Jayne, J. T., Mortimer, P., Worsnop, D. R., Kolb, C. E., Johnson, K. S., Zuberi, B., Marr, L. C., Volkamer, R., Molina, L. T., Molina, M. J., Cardenas, B., Bernabé, R. M., Márquez, C., Gaffney, J. S., Marley, N. A., Laskin, A., Shutthanandan, V., Xie, Y., Brune, W., Lesher, R., Shirley, T., and Jimenez, J. L.: Characterization of ambient aerosols in Mexico City during the MCMA-2003 campaign with Aerosol Mass Spectrometry: results from the CENICA Supersite, Atmos. Chem. Phys., 6, 925–946, https://doi.org/10.5194/acp-6-925-2006, 2006. a
Sarangi, B., Aggarwal, S. G., Sinha, D., and Gupta, P. K.: Aerosol effective density measurement using scanning mobility particle sizer and quartz crystal microbalance with the estimation of involved uncertainty, Atmos. Meas. Tech., 9, 859–875, https://doi.org/10.5194/amt-9-859-2016, 2016. a
Satish, R., Shamjad, P., Thamban, N., Tripathi, S., and Rastogi, N.: Temporal characteristics of brown carbon over the Central Indo-Gangetic plain, Environ. Sci. Technol., 51, 6765–6772, https://doi.org/10.1021/acs.est.7b00734, 2017. a
Singh, A., Rastogi, N., Sharma, D., and Singh, D.: Inter and intra-annual variability in aerosol characteristics over Northwestern Indo-Gangetic plain, Aerosol Air Qual. Res., 15, 376–386, https://doi.org/10.4209/aaqr.2014.04.0080, 2015. a
Song, S., Gao, M., Xu, W., Shao, J., Shi, G., Wang, S., Wang, Y., Sun, Y., and McElroy, M. B.: Fine-particle pH for Beijing winter haze as inferred from different thermodynamic equilibrium models, Atmos. Chem. Phys., 18, 7423–7438, https://doi.org/10.5194/acp-18-7423-2018, 2018. a
Stone, E., Schauer, J., Quraishi, T. A., and Mahmood, A.: Chemical characterization and source apportionment of fine and coarse particulate matter in Lahore, Pakistan, Atmos. Environ., 44, 1062–1070, https://doi.org/10.1016/j.atmosenv.2009.12.015, 2010. a
Streets, D. G., Yarber, K. F., Woo, J.-H., and Carmichael, G. R.: Biomass burning in Asia: Annual and seasonal estimates and atmospheric emissions, Global Biogeochem. Cy., 17, 1099, https://doi.org/10.1029/2003GB002040, 2003. a
Sujatha, P., Mahalakshmi, D., Ramiz, A., Rao, P., and Naidu, C.: Ventilation coefficient and boundary layer height impact on urban air quality, Cogent Environmental Science, 2, 1125284, https://doi.org/10.1080/23311843.2015.1125284, 2016. a
Sun, Y. L., Wang, Z. F., Fu, P. Q., Yang, T., Jiang, Q., Dong, H. B., Li, J., and Jia, J. J.: Aerosol composition, sources and processes during wintertime in Beijing, China, Atmos. Chem. Phys., 13, 4577–4592, https://doi.org/10.5194/acp-13-4577-2013, 2013. a, b
Tiwari, S., Pandithurai, G., Attri, S., Srivastava, A., Soni, V., Bisht, D., Kumar, V. A., and Srivastava, M. K.: Aerosol optical properties and their relationship with meteorological parameters during wintertime in Delhi, India, Atmos. Res., 153, 465–479, https://doi.org/10.1016/j.atmosres.2014.10.003, 2015. a
Trivedi, D. K., Ali, K., and Beig, G.: Impact of meteorological parameters on the development of fine and coarse particles over Delhi, Sci. Total Environ., 478, 175–183, https://doi.org/10.1016/j.scitotenv.2014.01.101, 2014. a
van Donkelaar, A., Martin, R. V., Brauer, M., and Boys, B. L.: Use of satellite observations for long-term exposure assessment of global concentrations of fine particulate matter, Environ. Health Persp., 123, 135–143, https://doi.org/10.1289/ehp.1408646, 2015. a
Verma, S., Boucher, O., Shekar Reddy, M., Upadhyaya, H. C., Le Van, P., Binkowski, F. S., and Sharma, O. P.: Tropospheric distribution of sulphate aerosols mass and number concentration during INDOEX-IFP and its transport over the Indian Ocean: a GCM study, Atmos. Chem. Phys., 12, 6185–6196, https://doi.org/10.5194/acp-12-6185-2012, 2012. a
Vijayakumar, K., Safai, P., Devara, P., Rao, S. V. B., and Jayasankar, C.: Effects of agriculture crop residue burning on aerosol properties and long-range transport over northern India: A study using satellite data and model simulations, Atmos. Res., 178–179, 155–163, https://doi.org/10.1016/j.atmosres.2016.04.003, 2016. a
Vittal Murty, K. P. R., Viswanadham, D. V., and Sadhuram, Y.: Mixing heights and ventilation coefficients for urban centres in India, Bound.-Lay. Meteorol., 19, 441–451, https://doi.org/10.1007/BF00122344, 1980. a
Wang, J., Ge, X., Chen, Y., Shen, Y., Zhang, Q., Sun, Y., Xu, J., Ge, S., Yu, H., and Chen, M.: Highly time-resolved urban aerosol characteristics during springtime in Yangtze River Delta, China: insights from soot particle aerosol mass spectrometry, Atmos. Chem. Phys., 16, 9109–9127, https://doi.org/10.5194/acp-16-9109-2016, 2016. a
Warner, J. X., Dickerson, R. R., Wei, Z., Strow, L. L., Wang, Y., and Liang, Q.: Increased atmospheric ammonia over the world's major agricultural areas detected from space, Geophys. Res. Lett., 44, 2875–2884, https://doi.org/10.1002/2016GL072305, 2017. a
World Health Organization: AAP Air Quality Database, available at: http://www.who.int/phe/health_topics/outdoorair/databases/cities/en/, last access: 20 August 2018. a
Yudovich, Y. and Ketris, M.: Chlorine in coal: A review, Int. J. Coal Geol., 67, 127–144, https://doi.org/10.1016/j.coal.2005.09.004, 2006. a
Zhang, Q., Jimenez, J. L., Canagaratna, M. R., Allan, J. D., Coe, H., Ulbrich, I., Alfarra, M. R., Takami, A., Middlebrook, A. M., Sun, Y. L., Dzepina, K., Dunlea, E., Docherty, K., DeCarlo, P. F., Salcedo, D., Onasch, T., Jayne, J. T., Miyoshi, T., Shimono, A., Hatakeyama, S., Takegawa, N., Kondo, Y., Schneider, J., Drewnick, F., Borrmann, S., Weimer, S., Demerjian, K., Williams, P., Bower, K., Bahreini, R., Cottrell, L., Griffin, R. J., Rautiainen, J., Sun, J. Y., Zhang, Y. M., and Worsnop, D. R.: Ubiquity and dominance of oxygenated species in organic aerosols in anthropogenically-influenced Northern Hemisphere midlatitudes, Geophys. Res. Lett., 34, L13801, https://doi.org/10.1029/2007GL029979, 2007. a