the Creative Commons Attribution 4.0 License.
the Creative Commons Attribution 4.0 License.
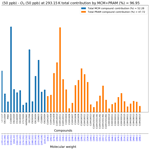
Aerosol mass yields of selected biogenic volatile organic compounds – a theoretical study with nearly explicit gas-phase chemistry
Carlton Xavier
Anton Rusanen
Putian Zhou
Chen Dean
Lukas Pichelstorfer
Pontus Roldin
Michael Boy
In this study we modeled secondary organic aerosol (SOA) mass loadings from the oxidation (by O3, OH and NO3) of five representative biogenic volatile organic compounds (BVOCs): isoprene, endocyclic bond-containing monoterpenes (α-pinene and limonene), exocyclic double-bond compound (β-pinene) and a sesquiterpene (β-caryophyllene). The simulations were designed to replicate an idealized smog chamber and oxidative flow reactors (OFRs). The Master Chemical Mechanism (MCM) together with the peroxy radical autoxidation mechanism (PRAM) were used to simulate the gas-phase chemistry. The aim of this study was to compare the potency of MCM and MCM + PRAM in predicting SOA formation. SOA yields were in good agreement with experimental values for chamber simulations when MCM + PRAM was applied, while a stand-alone MCM underpredicted the SOA yields. Compared to experimental yields, the OFR simulations using MCM + PRAM yields were in good agreement for BVOCs oxidized by both O3 and OH. On the other hand, a stand-alone MCM underpredicted the SOA mass yields. SOA yields increased with decreasing temperatures and NO concentrations and vice versa. This highlights the limitations posed when using fixed SOA yields in a majority of global and regional models. Few compounds that play a crucial role (>95 % of mass load) in contributing to SOA mass increase (using MCM + PRAM) are identified. The results further emphasized that incorporating PRAM in conjunction with MCM does improve SOA mass yield estimation.
- Article
(2930 KB) - Full-text XML
-
Supplement
(850 KB) - BibTeX
- EndNote
Atmospheric secondary organic aerosols, formed from gas-to-particle phase conversion of the oxidation products of volatile organic compounds (VOCs), significantly impact the organic aerosol mass loadings (Griffin, 1999; Kanakidou et al., 2005). However, the scale of SOA contribution to the aerosol particle mass is still subject to high uncertainties (Hao et al., 2011; Glasius and Goldstein, 2016). The elevated aerosol particle concentrations are shown to have inimical effects on health (Miller et al., 2007) and a varying degree of influence on the climate by forming cloud condensation nuclei (CCN), altering the cloud properties and radiative balance (Rosenfeld et al., 2014; Schmale et al., 2018). Therefore, it is acutely necessary to understand the contributions and role of SOA in the particle loading in the atmosphere. Biogenic VOCs from forest are estimated to contribute to about 90 % of VOC emissions globally (Guenther et al., 1995, 1999, 2000). The most important BVOCs for SOA formation are isoprene (C5H8), monoterpenes (C10H16) and sesquiterpenes (C15H24). These compounds are all alkenes containing at least one carbon–carbon double bond, enabling them to undergo oxidation by the dominant atmospheric oxidants: the hydroxyl radical (OH), ozone (O3) and the nitrate radical (NO3). For some of the terpenes, initial oxidation steps can lead to formation of highly oxygenated organic molecules (HOMs). These HOMs generally have low volatilities and can condense nearly irreversibly, thereby producing SOA (Ehn et al., 2014). HOMs, detected in both the ambient atmosphere and chamber experiments (Ehn et al., 2012) are formed by autoxidation (Berndt et al., 2016; Crounse and Nielsen, 2013) wherein peroxy radicals (RO2) undergo subsequent intramolecular H shifts accompanied by rapid reactions with O2. Autoxidation hence results in compounds containing multiple functional groups such as hydroxyls, peroxides and carbonyls (Bianchi et al., 2017, 2019).
A majority of chamber and flow-tube experiments have focused on HOM formation from the oxidation of various VOCs and their contribution to SOA mass loadings (Ehn et al., 2014; Kristensen et al., 2017). Oxidation of isoprene (Liu et al., 2016), endocyclic monoterpenes containing reactive double bonds such as α-pinene and limonene (Zhao et al., 2015), or exocyclic double-bond-containing compounds such as β-pinene (Jokinen et al., 2015) and sesquiterpenes such as β-caryophyllene (Chen et al., 2012) have been investigated. The SOA forming potential of various BVOCs depends on the isomeric structures (Friedman and Farmer, 2018; Keywood et al., 2004). Ozonolysis of compounds containing reactive endocyclic bonds such as α-pinene produces higher SOA mass yields of 41 % in comparison to those with exocyclic bonds (β-pinene), which produce mass yields of 17 % (Lee et al., 2006a). One explanation for this dependence on the isomeric structure is attributed to the formation of HOMs (Ehn et al., 2014). Another important factor influencing HOM formation is the initial oxidant, as pointed out by Zhao et al. (2015). They showed that the SOA formation by OH oxidation of α-pinene and limonene was lower when compared to their SOA formed by ozonolysis. Further they measured a lower H∕C ratio for SOA produced by monoterpene ozonolysis (experiments were carried out in the dark with CO as an OH scavenger), in comparison to OH oxidation of α-pinene and limonene, while the O∕C ratios were similar for both oxidation cases. This was attributed to the formation of RO2 radicals (monoterpenes + O3), which undergo internal hydrogen shifts and subsequently react with another RO2 radical to form compounds containing carbonyl groups while losing hydrogen atoms in the process. A similar analysis was conducted by Draper et al. (2015), who showed that an increase in NO2 concentration reduced α-pinene ozonolysis SOA mass yields, while no appreciable reduction in mass yields is reported for β-pinene and Δ3-carene ozonolysis. On the other hand, the mass yields from limonene ozonolysis increased with increasing NO2 concentrations (Draper et al., 2015). This disparity in mass yields for different BVOCs in the presence of NO2 is possibly caused by the formation of high-molecular-weight oligomers (or lack of in case of α-pinene) through oxidation with NO3 that contribute to SOA mass loadings (Draper et al., 2015).
Due to computational limitations, many regional- and canopy-scale atmospheric chemistry models generally use isoprene and/or a representative monoterpene (generally α-pinene) to model SOA yields (Friedman and Farmer, 2018). The SOA yields of different monoterpenes vary with structure, NOx and temperature (Friedman and Farmer, 2018; Kristensen et al., 2017; Presto et al., 2005). This poses a limitation on using representative monoterpene fixed SOA yields in many of the global models and increases uncertainties in predicting cloud condensation nuclei concentrations, cloud droplet number concentrations and radiative balance due to aerosol loadings.
This work aims to investigate the SOA mass loading from the oxidation products of BVOCs with the atmospheric oxidants OH, O3 and NO3 with a specific focus on the BVOCs isoprene, α-pinene, β-pinene, limonene and β-caryophyllene. Further we study the effect of varying temperature (258.15–313.15 K) and NO concentrations (0–5 ppb) on α-pinene oxidation mass yields. We use the master chemical mechanism (MCMv3.3.1) (Jenkin et al., 1997, 2012, 2015; Saunders et al., 2003), a nearly explicit gas-phase chemical mechanism together with peroxy radical autoxidation mechanism (PRAM; Roldin et al., 2019) (PRAM + MCM). The aim is to understand the importance and contribution of peroxy radical autoxidation products to the SOA mass yields from terpenes.
2.1 MALTE-Box
MALTE (Model to predict new Aerosol formation in Lower TropospherE) is a one-dimensional model consisting of modules calculating boundary layer meteorology, emissions of BVOCs, gas-phase chemistry and aerosol dynamics with the aim to simulate particle distribution and growth in the lower troposphere (Boy et al., 2006). In this study, a zero-dimensional version, MALTE-Box, is applied to simulate an ideal chamber and flow-tube environment (i.e., no wall loss effects are considered in this study). For the simulations performed in this study the emission module was switched off while only employing the gas-phase chemistry and aerosol dynamics module.
Kinetic PreProcessor (KPP) is used to generate a system of coupled differential equations to solve the gas-phase chemistry schemes (Damian et al., 2002). The peroxy radical autoxidation mechanism (PRAM; Roldin et al., 2019; Qi et al., 2018; Öström et al., 2017), formulated based on the oxidation of monoterpenes as described by Ehn et al. (2014), was incorporated alongside MCMv3.3.1. PRAM explicitly describes the formation and evolution of peroxy radicals (RO2) from the ozonolysis and OH oxidation of monoterpenes, driven by subsequent H shifts and O2 additions. The current version of PRAM based on experimental and theoretical studies considers HOM autoxidation for a fraction of the peroxy radicals formed during the ozonolysis of α-pinene and limonene and OH oxidation of α-pinene, β-pinene and limonene. This is achieved by assigning species specific molar yields for the formation of first RO2, which subsequently initiates the autoxidation chain (Roldin et al., 2019). Currently, in PRAM maximum first-generation RO2 yields of 9 % for α-pinene ozonolysis, 21.9 % for limonene ozonolysis, 2.5 % for α-pinene + OH, and 1 % for both limonene + OH and β-pinene + OH first-generation products are allowed to initiate autoxidation (Roldin et al., 2019). For β-pinene ozonolysis the molar yield of RO2 is minor (<0.1 %) (Roldin et al., 2019; Ehn et al., 2014) and hence not considered in this work. The abovementioned RO2 molar yields used in this work are close to the experimental values obtained in both smog chamber and flow-tube experiments. Ehn et al. (2014) measured an RO2 yield of ∼7 % for α-pinene ozonolysis and ∼17 % for limonene ozonolysis, whereas Jokinen et al. (2015) measured 0.58 % and 0.93 % for OH oxidation of β-pinene and limonene, respectively. The autoxidation is terminated by bimolecular reactions, wherein the RO2 formed reacts with NO, HO2 or other peroxy radicals, thereby forming alkoxy radicals, closed shell monomers or dimers (Roldin et al., 2019). The PRAM considers temperature-dependent autoxidation reaction rates, which is important when investigating the SOA mass yields at varying temperatures. The temperature dependence in PRAM is based on quantum chemical calculations wherein the autoxidation rates correspond to an activation energy of 24 kcal mol−1. The activation energies vary for autoxidation of different RO2 from α-pinene ozonolysis between 22 and 29 kcal mol−1 (Rissanen et al., 2015), leading to varying autoxidation rates at different temperatures (Roldin et al., 2019). It should be noted that the temperature dependence in PRAM is the first of its kind but needs further evaluation using recent measurements of HOM formation at different temperatures (e.g., Quéléver et al., 2019).
The aerosol dynamics are simulated using the University of Helsinki Multicomponent Aerosol model (UHMA) originally from Korhonen et al. (2004). The model has undergone significant development since then to allow simulation with all the compounds from MCM. It now supports an unlimited number of condensing vapors and solves condensation using the analytical predictor of the condensation method from Jacobson (1997). The condensation algorithm considers both, the Kelvin effect and Raoult's law. The processes included in the model are nucleation, condensation, evaporation, coagulation and deposition. The discretization of the size distribution and the time evolution is modeled with the moving section approach, with optional redistribution to a fixed grid. In this work, the redistribution is active to make the coagulation more accurate since it requires that grid points are available near the size of the coagulated particles. In this study nucleation and deposition are not active and hence are not considered. A total of 100 size bins ranging from 1nm to 20 µm with the fixed grid was applied for this study.
A group contribution method based on Nannoolal et al. (2008) using the UManSysProp online system (Topping et al., 2016) was used to estimate the pure liquid saturation vapor pressures (p0) of the organic compounds in MCMv3.3.1. For the PRAM species, p0 values were estimated using the functional group method SIMPOL (Pankow and Asher, 2008; see Roldin et al., 2019, for details). Temperature was used as an input to estimate p0 for both the group contribution methods.
2.2 Simulations
The simulations performed in this study are aimed to closely resemble an idealized smog chamber (batch mode setup) and an oxidative flow reactor (OFR) without interactions between the gas phase and the system walls. For the chamber runs, the VOC and oxidants were introduced at the beginning (time, t=0 s), set to certain concentrations (Table 1a) and then allowed to react. Both chamber and OFR simulations are performed using ammonium sulfate seed particles, which are introduced at time t=0. The condensation sink (CS) was inferred from the size distribution of seed particles used in the model. The CS for the chamber and OFR simulations was set to 0.00067 and 0.067 s−1, respectively. SOA mass yields obtained using an OFR are sensitive to the short residence time used; hence the seed particle surface area should be chosen in order to overcome the mass yield underestimation (Ahlberg et al., 2019). CS sensitivity runs (Supplement Fig. S1) were performed for α-pinene–O3 to determine the CS for which there is no appreciable change in mass yields with increasing particle surface.
Table 1(a) Concentrations of different BVOCs. (b) Concentrations of different oxidants for chamber and flow-tube runs. (c) NO concentrations and temperatures used for α-pinene ozonolysis.
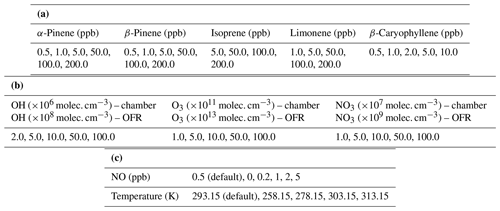
The simulation for the chamber setup is run for a maximum time of 24 h and ends when either of the two criteria are satisfied: (1) the simulation time reaches the 24 h mark or (2) 90 % of the initial precursor VOC has reacted away. In the latter case the simulation is continued for an additional 2 h to ensure enough time for the vapors to condense onto the seed particles. By contrast, the OFR runs were simulated for a maximum residence time of 100 s, ensuring all initial precursor vapors were oxidized. Seed particles were also added in the OFR simulations. The oxidant concentrations used for the OFR simulations (Table 1b) are significantly higher in comparison to the simulated chamber runs (∼2 orders of magnitude larger). The time steps for the chamber and flow-tube simulations are set to t=10 s and t=0.1 s, respectively. The runs performed were oxidant specific (i.e., VOCs would be oxidized by only one specific oxidant at any given time). For the O3 specific simulations no OH could form in both, OFR and chamber setups, thus making oxidation of O3 the only pathway.
The simulations were performed at atmospherically relevant NOx () concentrations, corresponding to [NO] = 0.5 ppb and [NO2] = 2.0 ppb conditions with the relative humidity (RH) set to 60 % and temperature to 293.15 K. The RH value considered in this study is based on previous published experimental studies performed at ∼60 % in both the smog chamber (Bruns et al., 2015; Ehn et al., 2014; Stirnweis et al., 2017) and OFR (Ahlberg et al., 2019). The α-pinene ozonolysis runs were performed at four different temperatures: 258.15, 278.15, 303.15 and 313.15 K, respectively (Table 1c). SOA mass yields are expected to increase with decreasing temperature (Saathoff et al., 2009). A similar temperature dependence was observed by Kristensen et al. (2017), who observed SOA mass yield from α-pinene ozonolysis at ∼40 % and ∼20 % at 258 and 293 K, respectively. Analogous to analyzing the effect of varying temperature on SOA yields, we study the variation in α-pinene ozonolysis SOA mass yields by varying the NOx concentrations (Table 1c). SOA yields for α-pinene ozonolysis at high NOx conditions should be suppressed (Ng et al., 2007), which could be due to the production of relatively volatile organic nitrates under high-NOx conditions compared to less volatile products during low-NOx conditions (Presto et al., 2005).
Furthermore, two different chemistry schemes were applied for the simulations. One scheme consisted of only the MCM chemistry mechanism and the second included the MCM + PRAM chemistry mechanism. Table 1a shows the concentrations of different BVOCs and Table 1b shows the oxidant concentrations used for the simulations.
2.3 Mass yields
The SOA mass yields (Y) are determined by calculating the ratio of the amount of SOA or mass concentration of organic aerosol formed (COA) to the amount of VOC (ΔVOC) reacted:
A volatility basis set is fit to the data to obtain the volatility distribution. In this study equilibrium partitioning was only assumed for deriving the volatility distribution based on the model simulations. Following Donahue et al. (2006), the SOA is assumed to be in equilibrium with the gas phase and using the effective saturation concentration spaced logarithmically. The individual product partitioning to the particle phase can be estimated using
where Ei is the fraction of species in the condensed particle phase. The above equation determines the fraction of species in the particle phase as well as in the gas phase. For example, if we assume COA=10 µg m−3, a species with µg m−3 will partition 50 % to the condensed phase and the other 50 % will reside in the gas phase. The fidelity of this equilibrium partitioning enables the parameterization of product vapors in volatility C∗ bins that are near the COA concentrations (Henry et al., 2012).
SOA mass yields were simulated for the oxidation of various biogenic volatile organic compounds (isoprene, α-pinene, limonene and β-caryophyllene, β-pinene) by dominant atmospheric oxidants OH, O3 and NO3. The following section examines the comparison between the yields derived using MCM + PRAM and a stand-alone MCM for chamber and flow-tube experiments.
3.1 BVOCs – O3 chamber and flow-tube simulations
Figure 1a indicates the SOA mass yields derived on applying a coupled MCM + PRAM mechanism to ozonolysis of α-pinene and limonene (PRAM is only available for ozonolysis of α-pinene and limonene) and panel (b) shows the ratio of yields obtained by MCM and coupled MCM + PRAM.
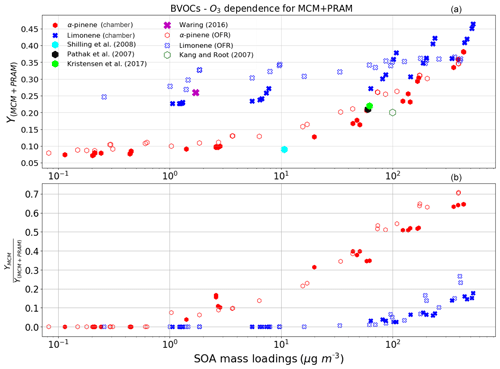
Figure 1The mass yields from the ozonolysis of BVOCs α-pinene (red heptagon) and limonene (blue crosses) modeled after chamber (filled symbols) and flow-tube settings (open symbols). The figure shows a comparison of SOA mass yields obtained from simulations with MCM + PRAM (a) and ratio of yields from MCM and MCM + PRAM (b). Currently PRAM is available for ozonolysis of limonene and α-pinene. The clumps are a result of SOA mass yields for the oxidation of specific oxidant concentrations with varying BVOC concentration.
The abscissa, depicted on a log scale, considers the entire range of SOA mass loadings from 1 to 800 µg m−3. Each data point is representative of simulated SOA mass yields resulting from variable BVOC loading. The resulting mass yields for α-pinene in the range shown in Table 2a are consistent with the yields found in various smog chamber experiments. The mass yields derived using MCM + PRAM for α-pinene ozonolysis are in good agreement with the experimental yields measured for similar mass loadings by Kristensen et al. (2017) and Pathak et al. (2007). The stand-alone MCM, on the other hand, severely underpredicts the mass yields for α-pinene ozonolysis. The MCM + PRAM also shows better agreement with experiments when estimating the lower range mass yields for SOA mass loadings of <15 µg m−3. This is supported by the values obtained by Shilling et al. (2008), where the authors measured a 0.09 yield from α-pinene ozonolysis for SOA mass loading of 10.6 µg m−3. Limonene ozonolysis mass yields using MCM + PRAM in comparison to stand-alone MCM are much closer to the values given by Waring (2016).
Table 2(a) Mass yields for BVOC ozonolysis at 293 K for different ranges of mass loadings using a chamber setup. The values in parentheses in the column “Experimental yields” indicate the corresponding experimental mass loadings in micrograms per cubic meter. (b) Mass yields for BVOC ozonolysis at 293 K for different ranges of mass loadings using an OFR setup.
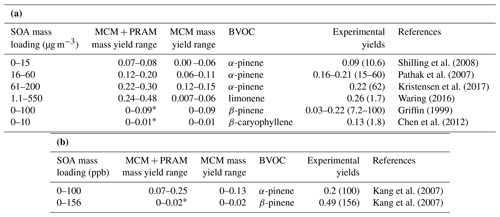
∗ Indicates that no PRAM mechanism is available yet; i.e the yields are the same as the MCM yields.
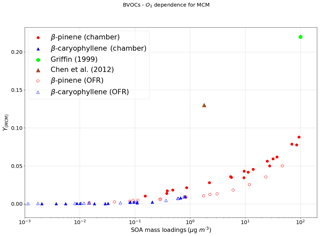
Figure 2The mass yields from the ozonolysis of BVOCs β-pinene and β-caryophyllene modeled after chamber (filled symbols) and flow-tube (open symbols) settings. The figure shows a comparison of SOA mass yields obtained from simulations with only MCM as currently there is no PRAM available for these compounds. The experimental values are provided for comparison.
The formation of HOM from β-pinene ozonolysis is low (Ehn et al., 2014; Jokinen et al., 2015) and hence not considered in PRAM. The peroxy radical autoxidation mechanism for β-caryophyllene ozonolysis has not yet been developed and therefore is not considered in PRAM. When comparing the measured mass yield values for β-caryophyllene (Chen et al., 2012) and β-pinene ozonolysis (Griffin, 1999; Pathak et al., 2008) to the modeled values using the MCM scheme, it is evident that the MCM scheme drastically underpredicts the SOA mass yields (Fig. 2).
Today oxidation flow reactor (OFR) experiments complement the traditional batch mode smog chamber experiments. The OFR generally exhibits lower mass yields compared to the smog chamber experiments at ranges of equivalent oxidant exposure (Lambe et al., 2015). We modeled flow-tube simulation after the potential aerosol mass (PAM) OFR, where the residence time is on the order of a few to several minutes (Lambe et al., 2011). The model simulations are performed with a maximum residence time of 100 s with O3 exposures ranging from 1.0×1015 to 1.0×1017 molecules cm−3 s (residence time × [O3]). Kang et al. (2007) measured a yield of 0.2 for ozonolysis of α-pinene for an initial precursor VOC concentration of 100 ppbv, while we obtain ∼0.25 (MCM + PRAM) for the similar initial precursor concentrations. The OFR yields for β-pinene (MCM-only) are significantly lower (0.02) than the values measured by Kang et al. (2007), wherein they measured a yield of 0.49 for similar initial precursor concentrations. Addition of seed particles promotes condensation, leading to increased SOA yields (Lambe et al., 2015), which was confirmed by Ahlberg et al. (2019). Kang et al. (2007) found that when using seed particles the yield from α-pinene ozonolysis increased by a factor of ∼1.4, which can explain our yields for α-pinene ozonolysis simulations. The mass spectra plot (Fig. S2) shows that PRAM contributes the majority of dimers to the particle phase, while MCM dominates the monomer contribution. Another interesting facet of Fig. S2 are the different condensing compounds in both OFR and chamber simulations. The higher absolute RO2 concentrations in the OFR simulations explain the lower concentration of HOM monomers and dimers relative to the chamber simulations; i.e., the high RO2 concentrations in the OFR cause termination of the peroxy radical autoxidation chain before the RO2 becomes highly oxygenated, thereby influencing SOA yields. Hence, this should be taken into account when using yields from OFR as inputs to regional and global models.
3.2 BVOCs – OH chamber and flow-tube simulations
The mass yields obtained by MCM + PRAM for α-pinene–OH oxidation are close to the measured values (Kristensen et al., 2017), while using only MCM underpredicts the mass yields (Fig. 3a and b and Table 3). The maximum SOA mass yield for OH oxidation of α-pinene is lower than the yield from ozonolysis, which is suspected to arise due to the formation of more volatile oxidation products produced during OH oxidation (Bonn and Moortgat, 2002; Kristensen et al., 2014). The OH oxidation of β-pinene results in mass yields similar to the measurements obtained by Lee et al. (2006b) for similar mass loadings. The β-pinene SOA yields are comparatively well represented by MCM + PRAM in comparison to the stand-alone MCM. On the other hand, the limonene mass yields are underpredicted by MCM + PRAM for similar mass loadings. Yields for limonene SOA mass loadings of 350 µg m−3 are around 0.31, which is lower than the experimental values of 0.58, measured by Lee et al. (2006b).
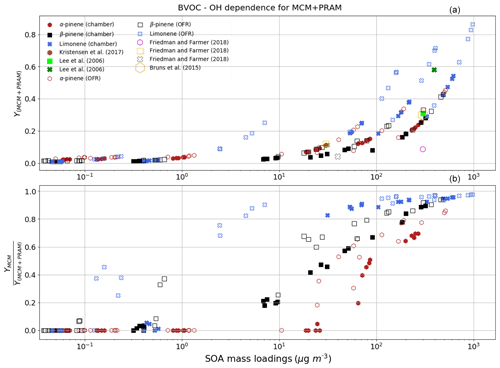
Figure 3The mass yields from OH oxidation of BVOCs α-pinene (red heptagons), β-pinene (black squares) and limonene (blue crosses) modeled after chamber (filled symbols) and flow-tube settings (open symbols). The figure shows a comparison of SOA mass yields obtained from application of MCM + PRAM (a) and ratio of yields from MCM and coupled MCM + PRAM (b). Currently PRAM is available for OH oxidation of limonene, α-pinene and β-pinene.
Table 3Mass yields for OH oxidation of BVOCs at 293 K for different ranges of mass loadings using a chambera and OFRb setup.
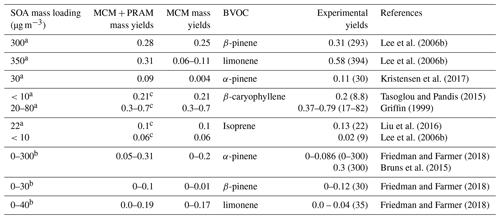
c Indicates that no PRAM mechanism is available yet; i.e., the yields are the same as the MCM yields.
For β-caryophyllene, the modeled values are in good agreement with experimental measured yields in the range of mass loadings provided by Griffin (1999) and Tasoglou and Pandis (2015). Currently there are no experiments providing HOM yields from OH oxidation of β-caryophyllene, and hence those species are not included in PRAM. The simulation results for yields from OH oxidation of β-caryophyllene indicate that the MCM scheme is able to reproduce the experimental values (Fig. 4). Only MCM was used for modeling the mass yields for OH oxidation of isoprene due to the current lack of a PRAM mechanism for isoprene. The mass yields derived from OH oxidation of isoprene vary from 0.01 to 0.31 covering a range of mass loadings from 0.003 to 132 µg m−3. At low mass loadings <10 µg m−3 the maximum yield obtained is ∼0.06, which is a factor of 3 greater than the experimental results obtained by Lee et al. (2006b), who measured a yield of 0.02. The mass yields are in good agreement with the experimental results from Liu et al. (2016), wherein they measured a yield of 0.13 for 22 µg m−3 (Table 3).
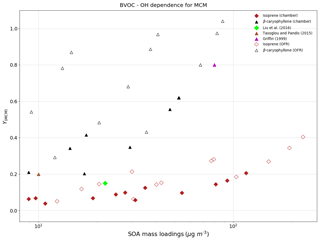
Figure 4The mass yields from OH oxidation of BVOCs β-caryophyllene (black triangles) and isoprene (maroon diamonds) modeled after chamber (filled symbols) and flow-tube settings (open symbols). The figure shows a comparison of SOA mass yields obtained from application of MCM as currently there is no PRAM available for these compounds.
The OFR simulation results for the OH oxidation of BVOCs with an equivalent exposure range from 2.0×1010 to 2.0×1012 molecules cm−3 s are shown in Fig. 3. Our yields for α-pinene agree well with the yields obtained by Bruns et al. (2015), who measured a yield of ∼0.3 for a mass loading of ∼300 µg m−3 at equivalent OH exposures. Friedman and Farmer (2018) found mass yields of 0–0.086 for α-pinene (ammonium sulfate seeded experiment), 0–0.12 for β-pinene (no seed particles) and 0–0.04 for limonene (no seed particles), by varying the OH exposures between 4.7×1010 and 7.4×1011 molecules cm−3 s. Our simulated yields for OH oxidation of α-pinene, β-pinene and limonene suggest higher mass yields for α-pinene and limonene at equivalent mass loadings, while mass yields for β-pinene are in good agreement with the experimental yields. Friedman and Farmer (2018) suggest that the reason for this underestimation in mass yields could be the exclusion of large particle sizes in the experiments, and they propose that these yields could represent lower bounds.
3.3 BVOC – NO3 chamber and OFR simulations
Figure 5 shows the yields derived from the oxidation of BVOCs by NO3. Currently, as no PRAM is available for NO3 oxidation, Fig. 5 represents SOA yields derived using MCM. Due to limited experimental constraints, PRAM presently does not consider autoxidation of RO2 formed from NO3 oxidation of VOCs, which could explain the huge discrepancy between the measured and simulated mass yields (Fig. 5). The yields obtained for oxidation of α-pinene (0.002–0.007) by NO3 are low in comparison to those obtained by Nah et al. (2016), where they measured a yield of 0.036. Measured mass yields for limonene oxidation by NO3 result in mass yields between 0.25 and 0.4 (Fry et al., 2011), whereas we obtain negligible (∼0.0003) mass yields.
3.4 NOx dependence
Varying NOx concentrations changes the fate of RO2 radicals formed during organic oxidations by altering the HO2∕RO2 ratio, thereby impacting the distribution of reaction products and aerosol formation (Presto et al., 2005; Zhao et al., 2018; Sarrafzadeh et al., 2016). We modeled the SOA mass yields for the α-pinene–O3 setup with varying NOx concentrations (NO was varied whereas NO2 was kept constant for all the runs), for initial α-pinene mixing ratios in the range 0.5–200 ppb (Fig. 6). A maximum SOA yield value of 0.55 is obtained for a combination of the lowest values of NO (0 ppb, red circles). As the NO concentrations increase from 0.2 ppb (blue squares) to 5 ppb (green inverted triangles) the yields begin to decrease, and this pattern is observable and valid for all concentration ranges of reacted precursor VOC. The NOx dependence of α-pinene ozonolysis is consistent with the findings of Draper et al. (2015) and Presto et al. (2005), who observed a trend of decreasing SOA mass yields for α-pinene ozonolysis with increasing NOx concentrations.
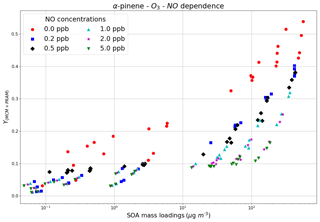
Figure 6The SOA mass yields from O3 oxidation of α-pinene modeled for different NO concentrations with the chamber setup. The model runs were performed using MCM + PRAM.
At low NOx concentrations RO2 radicals undergo rapid autoxidation until they react with HO2 or RO2, resulting in production of low-volatility hydroperoxide products (Sarrafzadeh et al., 2016), closed shell monomers or dimers (Ehn et al., 2014; Roldin et al., 2019), which increase SOA mass. This contrasts with high-NOx conditions where the RO2+NO reactions dominate over reactions with HO2 or RO2, resulting in the formation of more volatile products such as aldehydes, ketones and organonitrates (Presto et al., 2005; Sarrafzadeh et al., 2016) and likely suppressing the autoxidation process leading to a decrease in SOA mass loadings (Ehn et al., 2014).
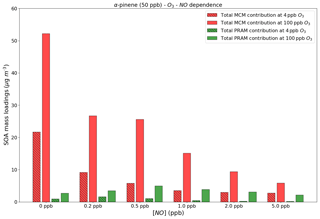
Figure 7Contribution to the SOA mass loadings by total PRAM and MCM compounds at different NO levels and O3 concentrations. For comparison we use 4 and 100 ppb O3 concentrations, respectively, at 50 ppb α-pinene.
Figure 7 shows the absolute contributions to SOA mass loadings by PRAM and MCM compounds at two different O3 concentrations of 4 and 100 ppb and varying NO concentrations. The figure shows that with an increase in NO concentrations of more than 1 ppb the contribution of PRAM compounds to the particle phase decreases at both 4 and 100 ppb of O3 concentrations. In PRAM the RO2+NO reaction leads to the formation of organonitrate HOM, closed-shell monomers with a carbonyl group or fragmentation products with higher volatility (Roldin et al., 2019). HOM dimer formation is suppressed with increasing NO concentrations in PRAM (Roldin et al., 2019), which explains the lower contribution by PRAM compounds to SOA mass loadings with increasing NO. At NO concentrations <1 ppb the PRAM contribution increases as first-generation RO2 products are capable of undergoing autoxidation, forming highly oxygenated RO2, which then reacts with NO to form organonitrates (Ehn et al., 2014). As NO concentrations exceed 1ppb the first-generation RO2 is scavenged by NO, thereby reducing the concentration of organonitrate HOM (Ehn et al., 2014), possibly affecting SOA yields. The MCM contribution also decreases with increasing NO concentrations mostly due to the formation of more volatile organonitrates (Jenkin et al., 2019).
3.5 Temperature dependence
The formation of SOA from α-pinene ozonolysis in the temperature range of 258.15–313.15 K was investigated in this study using MCM + PRAM. Strong dependence of SOA mass yield on temperature was reported by Saathoff et al. (2009), wherein they measured the decreasing mass yields from 0.42 at 273.15 K to 0.09 to 313.15 K for SOA loadings of 53 and 92 µg m−3, respectively. Our results in Fig. 8 show increasing SOA mass yields for α-pinene ozonolysis with decreasing temperature, which is attributed to the augmented condensation of oxidation products termed semi-volatile organic compounds (SVOCs) (Kristensen et al., 2017) at lower temperatures.
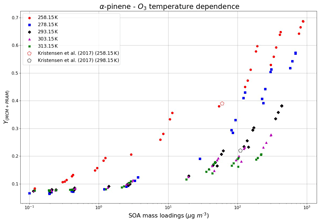
Figure 8Temperature dependence of SOA mass yields at different temperatures using the MCM + PRAM. The open pentagons represent measurement data from Kristensen et al. (2017) at 258.15 and 298.15 K.
For α-pinene maximum mass loading <150 µg m−3 the mass yields reach a maximum value of 0.38 at temperatures as low as 258.15 K and decrease to 0.27 for a temperature of 293.15 K and to 0.1 for a temperature of 313.15 K. These yields are comparable to the results obtained by Kristensen et al. (2017), who measured yields of 0.39 for 258.15 K and 0.22 for 293.15 K for mass loading <150 µg m−3. The results show a weak dependence of SOA mass yields on temperatures in the range of 278.15–313.15 K at low SOA mass loadings, which become more pronounced as the mass loadings increase. At the lowest temperature of 258.15 K the mass yields are higher in comparison to other temperatures regardless of the mass loadings. These results are in good agreement with the findings by Pathak et al. (2007), who found a strong temperature dependence of SOA mass yields at lower temperature (0–15 ∘C), which decreases as the temperature increases. Furthermore, similar to the measurements made by Pathak et al. (2007), our simulations were able to reproduce the experimental findings that show no appreciable differences in the SOA mass yields for loadings below 1 µg m−3 (initial mixing ratio of 1 ppb) for temperatures >273.15 K.
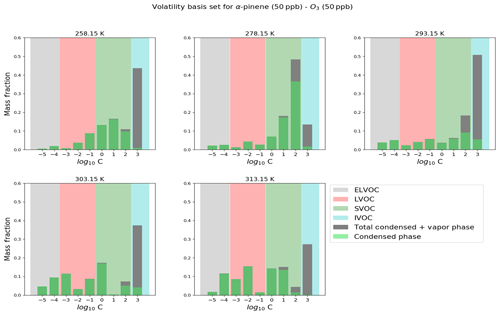
Figure 9Modeled volatility distribution of SOA at different temperatures. The volatility bins span a range of effective saturation vapor pressures to 103 µg m−3. The VBS distribution is based on a reference temperature of 298 K.
Figure 9 shows the volatility distribution of α-pinene-ozonolysis-derived SOA at different temperatures. The saturation vapor pressure limits for defining extremely low-volatility (ELVOCs – grey shaded), low-volatility (LVOCs – red shaded), semi-volatile (SVOCs – green shaded) and intermediate-volatility (IVOCs – cyan shaded) organic compounds used in the volatility basis set (VBS) are set according to the values suggested in Donahue et al. (2012). In this work, we categorize compounds (ELVOCs, LVOCs, SVOCs and IVOCs) based on effective saturation vapor pressures (C∗) in the range of 10−5 to 103 µg m−3 and temperature of 298 K (Donahue et al., 2009). At the lowest temperature of 258.15 K, the SVOC contribution to the particle phase is dominant in comparison to LVOCs and ELVOCs, a trend which is subsequently reversed as the temperatures are increased. At 293.15 K a majority of SVOCs and IVOCs are in the gas phase while the contribution of LVOCs and ELVOCs to the particle phase increases. These results are in good agreement with observations made by Kristensen et al. (2017), who observed an increasing contribution of SVOCs at subzero temperatures of 258.15 K, which decrease the fraction of SOA formed from ELVOCs. Again, it should be noted that the temperature dependence of peroxy radical autoxidation product formation still needs further validation based on recent experiments (e.g., Quéléver et al., 2019).
3.6 Composition
MCM + PRAM can be used to narrow down and compile a list of compounds playing a pivotal role in contributing to SOA mass loadings and also compare the relative importance of implementing PRAM alongside the MCM. Figure 10 shows the most important compounds from both the MCM and PRAM that together contribute to more than 95 % of α-pinene ozonolysis SOA mass loading at 293.15 K.
Figure 10 shows that contribution to SOA mass loadings by PRAM compounds is ∼48 % (of 97 %) while MCM compounds contribute ∼52 % (of 97 %). On lowering the temperature to 258 K the relative contributions of PRAM drop to 15 % (of ∼99 %), while MCM dominates by contributing ∼85 % (of ∼99 %), respectively (Fig. S3a). The contribution of PRAM increases to ∼64 % (of ∼97 %) and the MCM contribution drops to 36 % (of ∼97 %) at 313 K (Fig. S3b). These results reflect the importance of PRAM as its contribution plays an increasingly dominant role with increasing temperatures and highlights the crucial few compounds that contribute to maximum SOA mass loadings for α-pinene ozonolysis. The list of abundant compounds which together add up to contribute more than 95 % of SOA mass loadings at 258, 293 and 313 K are presented in the Supplement (Table S1a, b, c). At 258 K MCM compounds, namely pinonic acid (C10H16O3, 4.4 %), C920PAN (C10H15NO7, 9.3 %), C108NO3 (C10H15NO6, 8.9 %), C811PAN (C9H13NO7, 10.1 %) and C717NO3 (C7H9NO6, 11.3 %) contribute significantly to the total SOA mass loadings while PRAM compounds such as C10H14O7 (0.88 %), C10H16O4 (1.3 %) and C10H16O6 (1.13 %) contribute significantly less. An increase in temperature to 293 K results in an overall increase in contribution by PRAM compounds, with C10H14O10 (3.6 %), C10H14O11 (6.2 %) and C10H16O10 (3.2 %) playing an important role in contributing to the SOA mass loadings. This trend of relative increase in the contribution by PRAM compounds over MCM compounds to SOA mass loadings is also evident as the temperatures are further increased to 313 K, where the PRAM compounds C10H14O11 (18.3 %), C10H14O12 (6 %) and C10H16O12 (6.6 %) play a dominant role in increasing SOA mass loadings.
We simulated SOA mass yields derived from the oxidation of various BVOCs (isoprene, α-pinene, β-pinene, limonene and β-caryophyllene) by the oxidants O3, OH and NO3 using the zero-dimensional model MALTE-Box. The gas-phase chemistry was simulated using the MCM in conjunction with PRAM. The aim was to verify the efficacy of MCM + PRAM in simulating the SOA mass yields. Additional simulations were performed to test the MCM + PRAM under varying temperature and NO concentrations. A few important compounds playing a major role in increasing the SOA mass yields for α-pinene ozonolysis at different temperatures are also highlighted.
The simulations were designed to resemble ideal smog chamber experiments and experiments in oxidative flow reactors (OFR). No interactions between the gas phase and chamber walls were considered during the simulations. For the smog chamber setting, the stand-alone MCM generally underpredicts the mass yields obtained by the ozonolysis and OH oxidation of BVOCs. In contrast, the yields derived using MCM + PRAM for the smog chamber setup are in good agreement with the experimental results. For an idealized OFR setup, MCM + PRAM yields are in good agreement with experimental yields, while again the MCM underpredicts the SOA yields. The relative contribution of HOM monomers and dimers to the particle phase in OFR simulations is low when compared to the chamber simulations. This is due to higher RO2 concentrations in OFR leading to termination of peroxy radical autoxidation, thereby affecting SOA yields. This needs to be considered when applying yields based on OFR simulations in regional or global chemical transport models
The model does not simulate appreciable SOA mass yields for oxidation of BVOCs with NO3, as PRAM currently does not consider autoxidation of RO2 formed from NO3 oxidation of VOCs. This underlines the need for developing a NO3 oxidation scheme which can better constrain and predict SOA mass yields. In accordance with the previous studies, the simulated SOA yields tend to decrease at higher temperatures. The PRAM contribution to mass yields at low temperatures (258.15 K) is ∼14 %, which is substantially lower than that of MCM (∼86 %). As the temperature is increased to 313.15 K, the contribution of PRAM to SOA mass yields begins to dominate over MCM. This most likely is due to MCM producing more SVOCs (compounds classified as SVOCs at 298 K), which show a stronger contribution to the particle phase at lower temperatures, due to decrease in saturation vapor pressures with temperature. It should be noted that the present temperature dependency of mass yields using PRAM are a first and currently the best estimate in understanding the influence of temperature on the peroxy radical autoxidation formation. The simulated SOA yields with varying NO concentrations agree well with experimental results; i.e., SOA yields decrease with increasing NO concentrations due to the formation of more volatile compounds such as organonitrates and ketones.
Using PRAM coupled with MCM helps us bridge the gap in understanding the role and contribution of peroxy radical autoxidation to SOA formation. The variation in SOA yields for temperature and NO concentrations indicates the limitations of global and regional models in predicting cloud condensation nuclei (CCN) effects using fixed SOA yields. The good agreement of modeled and experimental yields from smog chambers could further help us parameterize the SOA yields, which could be applied at global and regional model scales to more accurately predict the direct and indirect impact of aerosol particles on radiation balance by aerosol scattering/absorption and CCN concentrations. Furthermore, implementation of a condensed PRAM version to regional and global models has been tested but still needs further validation (Roldin et al., 2019).
The complete PRAM mechanism written in a format compatible with the Kinetic PreProcessor (KPP) together with all species information can also be downloaded from https://doi.org/10.1594/PANGAEA.905102 (Roldin, 2019).
The supplement related to this article is available online at: https://doi.org/10.5194/acp-19-13741-2019-supplement.
CX and MB served as the chief authors and editors of the paper. CX performed the model simulations. The study was designed by CX, MB and PR. All other co-authors contributed to the analysis and writing of the paper.
The authors declare that they have no conflict of interest.
The presented research has been funded by the Academy of Finland (Center of Excellence in Atmospheric Sciences) grant no. 4100104 and the Swedish Research Council FORMAS, project no. 2018-01745. We would also like to acknowledge the invaluable contribution of computational resources from CSC – IT Center for Science, Finland.
This research has been supported by the Academy of Finland (Center of Excellence in Atmospheric Sciences
(grant no. 4100104)) and the Swedish
Research Council FORMAS (project no. 2018-01745).
Open access funding provided by Helsinki University Library.
This paper was edited by Barbara Ervens and reviewed by two anonymous referees.
Ahlberg, E., Eriksson, A., Brune, W. H., Roldin, P., and Svenningsson, B.: Effect of salt seed particle surface area, composition and phase on secondary organic aerosol mass yields in oxidation flow reactors, Atmos. Chem. Phys., 19, 2701–2712, https://doi.org/10.5194/acp-19-2701-2019, 2019.
Berndt, T., Richters, S., Jokinen, T., Hyttinen, N., Kurtén, T., Otkjær, R. V., Kjaergaard, H. G., Stratmann, F., Herrmann, H., Sipilä, M., Kulmala, M., and Ehn, M.: Hydroxyl radical-induced formation of highly oxidized organic compounds, Nat. Commun., 7, 13677, https://doi.org/10.1038/ncomms13677, 2016.
Bianchi, F., Garmash, O., He, X., Yan, C., Iyer, S., Rosendahl, I., Xu, Z., Rissanen, M. P., Riva, M., Taipale, R., Sarnela, N., Petäjä, T., Worsnop, D. R., Kulmala, M., Ehn, M., and Junninen, H.: The role of highly oxygenated molecules (HOMs) in determining the composition of ambient ions in the boreal forest, Atmos. Chem. Phys., 17, 13819–13831, https://doi.org/10.5194/acp-17-13819-2017, 2017.
Bianchi, F., Kurtén, T., Riva, M., Mohr, C., Rissanen, M. P., Roldin, P., Berndt, T., Crounse, J. D., Wennberg, P. O., Mentel, T. F., Wildt, J., Junninen, H., Jokinen, T., Kulmala, M., Worsnop, D. R., Thornton, J. A., Donahue, N., Kjaergaard, H. G., and Ehn, M.: Highly Oxygenated Organic Molecules (HOM) from Gas-Phase Autoxidation Involving Peroxy Radicals: A Key Contributor to Atmospheric Aerosol, Chem. Rev., 119, 3472–3509, https://doi.org/10.1021/acs.chemrev.8b00395, 2019.
Bonn, B. and Moorgat, G. K.: New particle formation during α- and β-pinene oxidation by O3, OH and NO3, and the influence of water vapour: particle size distribution studies, Atmos. Chem. Phys., 2, 183–196, https://doi.org/10.5194/acp-2-183-2002, 2002.
Boy, M., Hellmuth, O., Korhonen, H., Nilsson, E. D., ReVelle, D., Turnipseed, A., Arnold, F., and Kulmala, M.: MALTE – model to predict new aerosol formation in the lower troposphere, Atmos. Chem. Phys., 6, 4499–4517, https://doi.org/10.5194/acp-6-4499-2006, 2006.
Bruns, E. A., El Haddad, I., Keller, A., Klein, F., Kumar, N. K., Pieber, S. M., Corbin, J. C., Slowik, J. G., Brune, W. H., Baltensperger, U., and Prévôt, A. S. H.: Inter-comparison of laboratory smog chamber and flow reactor systems on organic aerosol yield and composition, Atmos. Meas. Tech., 8, 2315–2332, https://doi.org/10.5194/amt-8-2315-2015, 2015.
Chen, Q., Li, Y. L., McKinney, K. A., Kuwata, M., and Martin, S. T.: Particle mass yield from β-caryophyllene ozonolysis, Atmos. Chem. Phys., 12, 3165–3179, https://doi.org/10.5194/acp-12-3165-2012, 2012.
Crounse, J. D. and Nielsen, L. B.: Autoxidation of Organic Compounds in the Atmosphere, J. Phys. Chem. Lett., 24, 3513–3520, https://doi.org/10.1021/jz4019207, 2013.
Damian, V., Sandu, A., Damian, M., Potra, F., and Carmichael, G. R.: The kinetic preprocessor KPP – A software environment for solving chemical kinetics, Comput. Chem. Eng., 26, 1567–1579, https://doi.org/10.1016/S0098-1354(02)00128-X, 2002.
Donahue, N. M., Robinson, A. L., Stanier, C. O., and Pandis, S. N.: Coupled Partitioning, Dilution, and Chemical Aging of Semivolatile Organics, Environ. Sci. Technol., 40, 2635–2643, https://doi.org/10.1021/es052297c, 2006.
Donahue, N. M., Robinson, A. L., and Pandis, S. N.: Atmospheric organic particulate matter: From smoke to secondary organic aerosol, Atmos. Environ., 43, 94–106, https://doi.org/10.1016/j.atmosenv.2008.09.055, 2009.
Donahue, N. M., Kroll, J. H., Pandis, S. N., and Robinson, A. L.: A two-dimensional volatility basis set – Part 2: Diagnostics of organic-aerosol evolution, Atmos. Chem. Phys., 12, 615–634, https://doi.org/10.5194/acp-12-615-2012, 2012.
Draper, D. C., Farmer, D. K., Desyaterik, Y., and Fry, J. L.: A qualitative comparison of secondary organic aerosol yields and composition from ozonolysis of monoterpenes at varying concentrations of NO2, Atmos. Chem. Phys., 15, 12267–12281, https://doi.org/10.5194/acp-15-12267-2015, 2015.
Ehn, M., Kleist, E., Junninen, H., Petäjä, T., Lönn, G., Schobesberger, S., Dal Maso, M., Trimborn, A., Kulmala, M., Worsnop, D. R., Wahner, A., Wildt, J., and Mentel, Th. F.: Gas phase formation of extremely oxidized pinene reaction products in chamber and ambient air, Atmos. Chem. Phys., 12, 5113–5127, https://doi.org/10.5194/acp-12-5113-2012, 2012.
Ehn, M., Thornton, J. A., Kleist, E., Sipilä, M., Junninen, H., Pullinen, I., Springer, M., Rubach, F., Tillmann, R., Lee, B., Lopez-Hilfiker, F., Andres, S., Acir, I. H., Rissanen, M., Jokinen, T., Schobesberger, S., Kangasluoma, J., Kontkanen, J., Nieminen, T., Kurtén, T., Nielsen, L. B., Jørgensen, S., Kjaergaard, H. G., Canagaratna, M., Maso, M. D., Berndt, T., Petäjä, T., Wahner, A., Kerminen, V. M., Kulmala, M., Worsnop, D. R., Wildt, J., and Mentel, T. F.: A large source of low-volatility secondary organic aerosol, Nature, 506, 476–479, https://doi.org/10.1038/nature13032, 2014.
Friedman, B. and Farmer, D. K.: SOA and gas phase organic acid yields from the sequential photooxidation of seven monoterpenes, Atmos. Environ., 187, 335–345, https://doi.org/10.1016/j.atmosenv.2018.06.003, 2018.
Fry, J. L., Kiendler-Scharr, A., Rollins, A. W., Brauers, T., Brown, S. S., Dorn, H.-P., Dubé, W. P., Fuchs, H., Mensah, A., Rohrer, F., Tillmann, R., Wahner, A., Wooldridge, P. J., and Cohen, R. C.: SOA from limonene: role of NO3 in its generation and degradation, Atmos. Chem. Phys., 11, 3879–3894, https://doi.org/10.5194/acp-11-3879-2011, 2011.
Glasius, M. and Goldstein, A. H.: Recent Discoveries and Future Challenges in Atmospheric Organic Chemistry, Environ. Sci. Technol., 50, 2754–2764, https://doi.org/10.1021/acs.est.5b05105, 2016.
Griffin, R. J.: Organic aerosol formation from the oxidation of biogenic hydrocarbons, J. Geophys. Res.-Atmos., 104, 3555–3567, 1999.
Guenther, A., Nicholas Hewitt, C., David, E., Fall, R., Chris, G., Tom, G., Peter, H., Klinger, L., Manuel, L., Mckay, W. A., Tom, P., Scholes, B., Steinbrecher, R., Tallamraju, R., Taylor, J., and Zimmerman, P.: A global model of natural volatile organic compound emissions s Raja the balance Triangle changes in the atmospheric accumulation rates of greenhouse Triangle Several inventories of natural and Exposure Assessment global scales have been two classes Fores, J. Geophys. Res., 100, 8873–8892, https://doi.org/10.1029/94JD02950, 1995.
Guenther, A., Baugh, B., Brasseur, G., Greenberg, J., Harley, P., Klinger, L., Serca, D., and Vierling, L.: Isoprene emission estimates and uncertainties for the Central African EXPRESSO study domain, J. Geophys. Res.-Atmos., 104, 30625–30639, https://doi.org/10.1029/1999JD900391, 1999.
Guenther, A., Geron, C., Pierce, T., Lamb, B., Harley, P., and Fall, R.: Natural emissions of non-methane volatile organic compounds, carbon monoxide, and oxides of nitrogen from North America, Atmos. Environ., 34, 2205–2230, https://doi.org/10.1016/S1352-2310(99)00465-3, 2000.
Hao, L. Q., Romakkaniemi, S., Yli-Pirilä, P., Joutsensaari, J., Kortelainen, A., Kroll, J. H., Miettinen, P., Vaattovaara, P., Tiitta, P., Jaatinen, A., Kajos, M. K., Holopainen, J. K., Heijari, J., Rinne, J., Kulmala, M., Worsnop, D. R., Smith, J. N., and Laaksonen, A.: Mass yields of secondary organic aerosols from the oxidation of α-pinene and real plant emissions, Atmos. Chem. Phys., 11, 1367–1378, https://doi.org/10.5194/acp-11-1367-2011, 2011.
Henry, K. M., Lohaus, T., and Donahue, N. M.: Organic Aerosol Yields from α-Pinene Oxidation: Bridging the Gap between First-Generation Yields and Aging Chemistry, Environ. Sci. Technol., 46, 12347–12354, https://doi.org/10.1021/es302060y, 2012.
Jacobson, M. Z.: Numerical techniques to solve condensational and dissolutional growth equations when growth is coupled to reversible reactions, Aerosol Sci. Tech., 27, 491–498, https://doi.org/10.1080/02786829708965489, 1997.
Jenkin, M. E., Saunders, S. M., and Pilling, M. J.: The tropospheric degradation of volatile organic compounds: A protocol for mechanism development, Atmos. Environ., 31, 81–104, https://doi.org/10.1016/S1352-2310(96)00105-7, 1997.
Jenkin, M. E., Wyche, K. P., Evans, C. J., Carr, T., Monks, P. S., Alfarra, M. R., Barley, M. H., McFiggans, G. B., Young, J. C., and Rickard, A. R.: Development and chamber evaluation of the MCM v3.2 degradation scheme for β-caryophyllene, Atmos. Chem. Phys., 12, 5275–5308, https://doi.org/10.5194/acp-12-5275-2012, 2012.
Jenkin, M. E., Young, J. C., and Rickard, A. R.: The MCM v3.3.1 degradation scheme for isoprene, Atmos. Chem. Phys., 15, 11433–11459, https://doi.org/10.5194/acp-15-11433-2015, 2015.
Jenkin, M. E., Valorso, R., Aumont, B., and Rickard, A. R.: Estimation of rate coefficients and branching ratios for reactions of organic peroxy radicals for use in automated mechanism construction, Atmos. Chem. Phys., 19, 7691–7717, https://doi.org/10.5194/acp-19-7691-2019, 2019.
Jokinen, T., Berndt, T., Makkonen, R., Kerminen, V.-M., Junninen, H., Paasonen, P., Stratmann, F., Herrmann, H., Guenther, A. B., Worsnop, D. R., Kulmala, M., Ehn, M., and Sipilä, M.: Production of extremely low volatile organic compounds from biogenic emissions: Measured yields and atmospheric implications, P. Natl. Acad. Sci. USA, 112, 7123–7128, https://doi.org/10.1073/pnas.1423977112, 2015.
Kanakidou, M., Seinfeld, J. H., Pandis, S. N., Barnes, I., Dentener, F. J., Facchini, M. C., Van Dingenen, R., Ervens, B., Nenes, A., Nielsen, C. J., Swietlicki, E., Putaud, J. P., Balkanski, Y., Fuzzi, S., Horth, J., Moortgat, G. K., Winterhalter, R., Myhre, C. E. L., Tsigaridis, K., Vignati, E., Stephanou, E. G., and Wilson, J.: Organic aerosol and global climate modelling: a review, Atmos. Chem. Phys., 5, 1053–1123, https://doi.org/10.5194/acp-5-1053-2005, 2005.
Kang, E., Root, M. J., Toohey, D. W., and Brune, W. H.: Introducing the concept of Potential Aerosol Mass (PAM), Atmos. Chem. Phys., 7, 5727–5744, https://doi.org/10.5194/acp-7-5727-2007, 2007.
Keywood, M. D., Varutbangkul, V., Bahreini, R., Flagan, R. C., and Seinfeld, J. H.: Secondary organic aerosol formation from the ozonolysis of cycloalkenes and related compounds, Environ. Sci. Technol., 38, 4157–4164, https://doi.org/10.1021/es035363o, 2004.
Korhonen, H., Lehtinen, K. E. J., and Kulmala, M.: Multicomponent aerosol dynamics model UHMA: model development and validation, Atmos. Chem. Phys., 4, 757–771, https://doi.org/10.5194/acp-4-757-2004, 2004.
Kristensen, K., Cui, T., Zhang, H., Gold, A., Glasius, M., and Surratt, J. D.: Dimers in α-pinene secondary organic aerosol: effect of hydroxyl radical, ozone, relative humidity and aerosol acidity, Atmos. Chem. Phys., 14, 4201–4218, https://doi.org/10.5194/acp-14-4201-2014, 2014.
Kristensen, K., Jensen, L. N., Glasius, M., and Bilde, M.: The effect of sub-zero temperature on the formation and composition of secondary organic aerosol from ozonolysis of alpha-pinene, Environ. Sci. Process. Impacts, 19, 1220–1234, https://doi.org/10.1039/c7em00231a, 2017.
Lambe, A. T., Onasch, T. B., Massoli, P., Croasdale, D. R., Wright, J. P., Ahern, A. T., Williams, L. R., Worsnop, D. R., Brune, W. H., and Davidovits, P.: Laboratory studies of the chemical composition and cloud condensation nuclei (CCN) activity of secondary organic aerosol (SOA) and oxidized primary organic aerosol (OPOA), Atmos. Chem. Phys., 11, 8913–8928, https://doi.org/10.5194/acp-11-8913-2011, 2011.
Lambe, A. T., Chhabra, P. S., Onasch, T. B., Brune, W. H., Hunter, J. F., Kroll, J. H., Cummings, M. J., Brogan, J. F., Parmar, Y., Worsnop, D. R., Kolb, C. E., and Davidovits, P.: Effect of oxidant concentration, exposure time, and seed particles on secondary organic aerosol chemical composition and yield, Atmos. Chem. Phys., 15, 3063–3075, https://doi.org/10.5194/acp-15-3063-2015, 2015.
Lee, A., Goldstein, A. H., Keywood, M. D., Gao, S., Varutbangkul, V., Bahreini, R., Ng, N. L., Flagan, R. C., and Seinfeld, J. H.: Gas-phase products and secondary aerosol yields from the ozonolysis of ten different terpenes, J. Geophys. Res.-Atmos., 111, 1–18, https://doi.org/10.1029/2005JD006437, 2006a.
Lee, A., Goldstein, A. H., Kroll, J. H., Ng, N. L., Varutbangkul, V., Flagan, R. C., and Seinfeld, J. H.: Gas-phase products and secondary aerosol yields from the photooxidation of 16 different terpenes, J. Geophys. Res.-Atmos., 111, 1–25, https://doi.org/10.1029/2006JD007050, 2006b.
Liu, J., D'Ambro, E. L., Lee, B. H., Lopez-Hilfiker, F. D., Zaveri, R. A., Rivera-Rios, J. C., Keutsch, F. N., Iyer, S., Kurten, T., Zhang, Z., Gold, A., Surratt, J. D., Shilling, J. E., and Thornton, J. A.: Efficient Isoprene Secondary Organic Aerosol Formation from a Non-IEPOX Pathway, Environ. Sci. Technol., 50, 9872–9880, https://doi.org/10.1021/acs.est.6b01872, 2016.
Miller, K. A., Siscovick, D. S., Sheppard, L., Shepherd, K., Sullivan, J. H., Anderson, G., L., and Kaufman J. D.: Long-Term Exposure to Air Pollution and Incidence of Cardiovascular Events in Women, New Engl. J. Med., 356, 447–458, https://doi.org/10.1002/anie.201206370, 2007.
Nah, T., Sanchez, J., Boyd, C. M., and Ng, N. L.: Photochemical Aging of α-pinene and β-pinene Secondary Organic Aerosol formed from Nitrate Radical Oxidation, Environ. Sci. Technol., 50, 222–231, https://doi.org/10.1021/acs.est.5b04594, 2016.
Nannoolal, Y., Rarey, J., and Ramjugernath, D.: Estimation of pure component properties part 3. Estimation of the vapor pressure of non-electrolyte organic compounds via group contribution and group interactions, Fluid Phase Equilib., 269, 117–133, https://doi.org/10.1016/j.fluid.2008.04.020, 2008.
Ng, N. L., Chhabra, P. S., Chan, A. W. H., Surratt, J. D., Kroll, J. H., Kwan, A. J., McCabe, D. C., Wennberg, P. O., Sorooshian, A., Murphy, S. M., Dalleska, N. F., Flagan, R. C., and Seinfeld, J. H.: Effect of NOx level on secondary organic aerosol (SOA) formation from the photooxidation of terpenes, Atmos. Chem. Phys., 7, 5159–5174, https://doi.org/10.5194/acp-7-5159-2007, 2007.
Öström, E., Putian, Z., Schurgers, G., Mishurov, M., Kivekäs, N., Lihavainen, H., Ehn, M., Rissanen, M. P., Kurtén, T., Boy, M., Swietlicki, E., and Roldin, P.: Modeling the role of highly oxidized multifunctional organic molecules for the growth of new particles over the boreal forest region, Atmos. Chem. Phys., 17, 8887–8901, https://doi.org/10.5194/acp-17-8887-2017, 2017.
Pankow, J. F. and Asher, W. E.: SIMPOL.1: a simple group contribution method for predicting vapor pressures and enthalpies of vaporization of multifunctional organic compounds, Atmos. Chem. Phys., 8, 2773–2796, https://doi.org/10.5194/acp-8-2773-2008, 2008.
Pathak, R., Donahue, N. M., and Pandis, S. N.: Ozonolysis of β-pinene: Temperature dependence of secondary organic aerosol mass fraction, Environ. Sci. Technol., 42, 5081–5086, https://doi.org/10.1021/es070721z, 2008.
Pathak, R. K., Stanier, C. O., Donahue, N. M., and Pandis, S. N.: Ozonolysis of α-pinene at atmospherically relevant concentrations: Temperature dependence of aerosol mass fractions (yields), J. Geophys. Res.-Atmos., 112, 1–8, https://doi.org/10.1029/2006JD007436, 2007.
Presto, A. A., Huff Hartz, K. E., and Donahue, N. M.: Secondary organic aerosol production from terpene ozonolysis. 2. Effect of NOx concentration, Environ. Sci. Technol., 39, 7046–7054, https://doi.org/10.1021/es050400s, 2005.
Qi, X., Ding, A., Roldin, P., Xu, Z., Zhou, P., Sarnela, N., Nie, W., Huang, X., Rusanen, A., Ehn, M., Rissanen, M. P., Petäjä, T., Kulmala, M., and Boy, M.: Modelling studies of HOMs and their contributions to new particle formation and growth: comparison of boreal forest in Finland and a polluted environment in China, Atmos. Chem. Phys., 18, 11779–11791, https://doi.org/10.5194/acp-18-11779-2018, 2018.
Quéléver, L. L. J., Kristensen, K., Normann Jensen, L., Rosati, B., Teiwes, R., Daellenbach, K. R., Peräkylä, O., Roldin, P., Bossi, R., Pedersen, H. B., Glasius, M., Bilde, M., and Ehn, M.: Effect of temperature on the formation of highly oxygenated organic molecules (HOMs) from alpha-pinene ozonolysis, Atmos. Chem. Phys., 19, 7609–7625, https://doi.org/10.5194/acp-19-7609-2019, 2019.
Rissanen, M. P., Kurtén, T., Sipilä, M., Thornton, J. A., Kausiala, O., Garmash, O., Kjaergaard, H. G., Petäjä, T., Worsnop, D. R., Ehn, M., and Kulmala, M.: Effects of chemical complexity on the autoxidation mechanisms of endocyclic alkene ozonolysis products: From methylcyclohexenes toward understanding α-pinene, J. Phys. Chem. A, 119, 4633–4650, https://doi.org/10.1021/jp510966g, 2015.
Roldin, P.: Peroxy Radical Autoxidation Mechanism (PRAM), PANGAEA, https://doi.org/10.1594/PANGAEA.905102, 2019.
Roldin, P., Ehn, M., Kurtén, T., Olenius, T., Rissanen, M. P., Sarnela, N., Elm, J., Rantala, P., Hao, L., Hyttinen, N., Heikkinen, L., Worsnop, D. R., Pichelstorfer, L., Xavier, C., Clusius, P., Öström, E., Petäjä, T., Kulmala, M., Vehkamäki, H., Virtanen, A., Riipinen, I., and Boy, M.: The role of highly oxygenated organic molecules in the Boreal aerosol-cloud-climate system, Nat. Commun., 10, 4370, https://doi.org/10.1038/s41467-019-12338-8, 2019.
Rosenfeld, D., Andreae, M. O., Asmi, A., Chin, M., De Leeuw, G., Donovan, D. P., Kahn, R., Kinne, S., Kivekäs, N., Kulmala, M., Lau, W., Schmidt, K., S., Suni, T., Wagner, T., Wild, M., and Quaas, J.: Global obser-vations of aerosol-cloud-precipitation-climate interactions, Rev. Geophys., 52, 750–808, https://doi.org/10.1002/2013RG000441, 2014.
Saathoff, H., Naumann, K.-H., Möhler, O., Jonsson, Å. M., Hallquist, M., Kiendler-Scharr, A., Mentel, Th. F., Tillmann, R., and Schurath, U.: Temperature dependence of yields of secondary organic aerosols from the ozonolysis of α-pinene and limonene, Atmos. Chem. Phys., 9, 1551–1577, https://doi.org/10.5194/acp-9-1551-2009, 2009.
Sarrafzadeh, M., Wildt, J., Pullinen, I., Springer, M., Kleist, E., Tillmann, R., Schmitt, S. H., Wu, C., Mentel, T. F., Zhao, D., Hastie, D. R., and Kiendler-Scharr, A.: Impact of NOx and OH on secondary organic aerosol formation from β-pinene photooxidation, Atmos. Chem. Phys., 16, 11237–11248, https://doi.org/10.5194/acp-16-11237-2016, 2016.
Saunders, S. M., Jenkin, M. E., Derwent, R. G., and Pilling, M. J.: Protocol for the development of the Master Chemical Mechanism, MCM v3 (Part A): tropospheric degradation of non-aromatic volatile organic compounds, Atmos. Chem. Phys., 3, 161–180, https://doi.org/10.5194/acp-3-161-2003, 2003.
Schmale, J., Henning, S., Henzing, B., Keskinen, H., Sellegri, K., Ovadnevaite, J., Bougiatioti, A., Kalivitis, N., Stavroulas, I., Jefferson, A., Park, M., Schlag, P., Kristensson, A., Iwamoto, Y., Pringle, K., Reddington, C., Aalto, P., Äijälä, M., Baltensperger, U., Bialek, J., Birmili, W., Bukowiecki, N., Ehn, M., Fjæraa, A. M., Fiebig, M., Frank, G., Fröhlich, R., Frumau, A., Furuya, M., Hammer, E., Heikkinen, L., Herrmann, E., Holzinger, R., Hyono, H., Kanakidou, M., Kiendler-Scharr, A., Kinouchi, K., Kos, G., Kulmala, M., Mihalopoulos, N., Motos, G., Nenes, A., O'Dowd, C., Paramonov, M., Petäjä, T., Picard, D., Poulain, L., Prévôt, A. S. H., Slowik, J., Sonntag, A., Swietlicki, E., Svenningsson, B., Tsurumaru, H., Wiedensohler, A., Wittbom, C., Ogren, J. A., Matsuki, A., Yum, S. S., Myhre, C. L., Carslaw, K., Stratmann, F., and Gysel, M.: Corrigendum: Collocated observations of cloud condensation nuclei, particle size distributions, and chemical composition, Sci. Data, 5, 180094, https://doi.org/10.1038/sdata.2018.94, 2018.
Shilling, J. E., Chen, Q., King, S. M., Rosenoern, T., Kroll, J. H., Worsnop, D. R., McKinney, K. A., and Martin, S. T.: Particle mass yield in secondary organic aerosol formed by the dark ozonolysis of α-pinene, Atmos. Chem. Phys., 8, 2073–2088, https://doi.org/10.5194/acp-8-2073-2008, 2008.
Stirnweis, L., Marcolli, C., Dommen, J., Barmet, P., Frege, C., Platt, S. M., Bruns, E. A., Krapf, M., Slowik, J. G., Wolf, R., Prévôt, A. S. H., Baltensperger, U., and El-Haddad, I.: Assessing the influence of NOx concentrations and relative humidity on secondary organic aerosol yields from α-pinene photo-oxidation through smog chamber experiments and modelling calculations, Atmos. Chem. Phys., 17, 5035–5061, https://doi.org/10.5194/acp-17-5035-2017, 2017.
Tasoglou, A. and Pandis, S. N.: Formation and chemical aging of secondary organic aerosol during the β-caryophyllene oxidation, Atmos. Chem. Phys., 15, 6035–6046, https://doi.org/10.5194/acp-15-6035-2015, 2015.
Topping, D., Barley, M., Bane, M. K., Higham, N., Aumont, B., Dingle, N., and McFiggans, G.: UManSysProp v1.0: an online and open-source facility for molecular property prediction and atmospheric aerosol calculations, Geosci. Model Dev., 9, 899–914, https://doi.org/10.5194/gmd-9-899-2016, 2016.
Waring, M. S.: Secondary organic aerosol formation by limonene ozonolysis: Parameterizing multi-generational chemistry in ozone- and residence time-limited indoor environments, Atmos. Environ., 144, 79–86, https://doi.org/10.1016/j.atmosenv.2016.08.051, 2016.
Zhao, D., Schmitt, S. H., Wang, M., Acir, I.-H., Tillmann, R., Tan, Z., Novelli, A., Fuchs, H., Pullinen, I., Wegener, R., Rohrer, F., Wildt, J., Kiendler-Scharr, A., Wahner, A., and Mentel, T. F.: Effects of NOx and SO2 on the secondary organic aerosol formation from photooxidation of α-pinene and limonene, Atmos. Chem. Phys., 18, 1611–1628, https://doi.org/10.5194/acp-18-1611-2018, 2018.
Zhao, D. F., Kaminski, M., Schlag, P., Fuchs, H., Acir, I.-H., Bohn, B., Häseler, R., Kiendler-Scharr, A., Rohrer, F., Tillmann, R., Wang, M. J., Wegener, R., Wildt, J., Wahner, A., and Mentel, Th. F.: Secondary organic aerosol formation from hydroxyl radical oxidation and ozonolysis of monoterpenes, Atmos. Chem. Phys., 15, 991–1012, https://doi.org/10.5194/acp-15-991-2015, 2015.