the Creative Commons Attribution 4.0 License.
the Creative Commons Attribution 4.0 License.
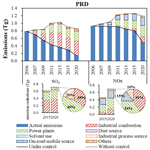
Evolution of anthropogenic air pollutant emissions in Guangdong Province, China, from 2006 to 2015
Yahui Bian
Zhijiong Huang
Jiamin Ou
Zhuangmin Zhong
Yuanqian Xu
Zhiwei Zhang
Xiao Xiao
Xiao Ye
Yuqi Wu
Xiaohong Yin
Cheng Li
Liangfu Chen
Min Shao
Junyu Zheng
Guangdong Province (GD), one of the most prosperous and populous regions in China, still experiences haze events and growing ozone pollution in spite of the substantial air-quality improvement in recent years. Integrated control of fine particulate matter (PM2.5) and ozone in GD calls for a systematic review of historical emissions. In this study, emission trends, spatial variations, source-contribution variations, and reduction potentials of sulfur dioxide (SO2), nitrogen oxides (NOx), PM2.5, inhalable particles (PM10), carbon monoxide (CO), ammonia (NH3), and volatile organic compounds (VOCs) in GD from 2006 to 2015 were first examined using a dynamic methodology, taking into account economic development, technology penetration, and emission controls. The relative change rates of anthropogenic emissions in GD during 2006–2015 are −48 % for SO2, −0.5 % for NOx, −16 % for PM2.5, −22 % for PM10, 13 % for CO, 3 % for NH3, and 13 % for VOCs. The declines of SO2, NOx, PM2.5, and PM10 emissions in the whole province mainly resulted from the stringent emission control in the Pearl River delta (PRD) region, where most previous control measures were focused, especially on power plants (SO2 and NOx), industrial combustion (SO2, PM2.5, PM10), on-road mobile sources (NOx), and dust sources (PM2.5 and PM10). Emissions from other areas (non-PRD, NPRD), nevertheless, remain relatively stable due to the lax control measures and rapidly growing energy consumption. In addition, emission leaks of SO2 and NOx from industries are observed from PRD to NPRD in 2010 and 2011. As a result, emissions in NPRD are increasingly important in GD, particularly those from industrial combustion. The contribution of NPRD to the total SO2 emissions in GD, for example, increased from 27 % in 2006 to 48 % in 2015. On-road mobile sources and solvent use are the two key sources that should receive more effective control measures in GD. Current control-driven emission reductions from on-road mobile sources are neutralized by the substantial growth of the vehicle population, while VOC emissions in GD steadily increase due to the growth of solvent use and the absence of effective control measures. Besides, future work could focus on power plants and industrial combustion in GD and industrial process sources in NPRD, which still have large emission reduction potentials. The historical emission inventory developed in this study not only helps to understand the emission evolution in GD, but also provides robust data to quantify the impact of emission and meteorology variations on air quality and unveil the primary cause of significant air-quality change in GD in the recent decade.
- Article
(5094 KB) - Full-text XML
-
Supplement
(9084 KB) - BibTeX
- EndNote
Guangdong Province (GD), comprising 9 major cities in the Pearl River delta (PRD) region and 11 less developed cities in the non-PRD (NPRD), is the most prosperous and populous regions adjacent to the coast of southern China (Fig. S1 in the Supplement). With only 1.9 % of China's land coverage, GD contributed 10.0 % of China's gross domestic product (GDP) and 8.0 % of the population in 2017 (GDPBS, 2018). Particularly, the PRD region, dubbed “the world's factory” and encompassing a wide variety of industries, represented about 80.0 % and 53.4 % of GD's total GDP and population, respectively (GDPBS, 2018). Benefitting from China's opening-up policies, GD has experienced rapid economic growth accompanied by serious and complex air pollution issues.
To improve air quality, great efforts have been made to formulate various control measures and policies, especially after 2013, when the Action Plan on the Prevention and Control of Air Pollution (PCAP, 2013–2017) was launched. The PCAP required the PRD region to reduce PM2.5 concentration by 15 % by the year 2017 compared with the 2013 levels. These policies and control measures, which were summarized in Table S1 in the Supplement, partly alleviated regional air pollution in GD. Ground-level observations showed that GD saw an obvious air-quality improvement, with SO2 and PM10 concentrations decreasing by 63.3 % and 17.7 %, respectively, from 2006 to 2017 (EPGD, 2006–2017). In particular, the PRD region is the first region to meet China's national standard of PM2.5 standard (35 µg m−3) for 3 consecutive years (34 µg m−3 in 2015, 32 µg m−3 in 2016, and 34 µg m−3 in 2017).
However, air pollution in GD is still a major concern. The annual PM2.5 levels still far exceed the WHO's PM2.5 guideline value of 10 µg m−3 (World Health Organization, 2006), and haze events frequently occur in winter (Tao et al., 2017). Also, the ambient ozone concentrations have been growing in recent years. The 90th percentile of the maximum 8 h average ozone concentration (90 %–8 h ozone) in the PRD region has increased by 24 % since 2015, reaching 165 µg m−3 in 2017 (GDEMC et al., 2018).
In contrast to the need for further air-quality improvement, the unclear causes of recent air-quality change in GD hinder the development of evidence-based air-quality control strategies. Although several studies had assessed the impact of emission and meteorology change on air quality, their results are inconsistent with each other. For instance, Lin et al. (2018) suggested that emission controls helped improve local air quality in the PRD region, according to a high consistency of ambient PM2.5 concentrations and emissions. However, Mao et al. (2018) argued that meteorological and climate conditions rather than PM emissions dominate the interannual variabilities and trends of winter haze days in PRD based on an observation-based approach. Yang et al. (2019) concluded that the inappropriate NOx∕VOC control ratio within PRD over the past years is likely responsible for the ozone increase, while Li et al. (2019) argued that the decrease in PM2.5 concentration is the main driver.
Further mitigation of air pollution in GD calls for a systematic review of historical emissions. First, the long-term historical emission data could help policymakers understand the evolution of emissions, quantify the cuts in emissions that have been achieved by control measures, and identify those sources with the greatest potential for large future emission reductions (Gurjar et al., 2004; Ohara et al., 2007; Zhong et al., 2013). This is particularly important for GD, whose potential for further emission reduction is shrinking as control measures tighten. Second, with the use of atmospheric chemical transport models (CTMs), the historical emission can be used to examine the linkages between air-quality improvements and control measures and thus identify the main causes of air-quality change and key control measures. All this information is crucial to guide future air-quality management and formulate robust evidence-based policies.
GD is one of the first areas to compile its own emission inventories in China (Zhong et al., 2013). However, no attempts have been made to estimate the historical emissions of GD. Most of the previous emission studies mainly focused on the PRD region but ignored the NPRD region that also has increased emissions. For instance, Zheng et al. (2009b) developed the first high-resolution emission inventory for the PRD region in 2006, followed by emission inventories for speciated volatile organic compounds (VOCs) (Zheng et al., 2009a), biogenic VOCs (Zheng et al., 2010), ammonia (NH3) (Yin et al., 2015), biomass burning (He et al., 2011; Zhang et al., 2013), sea salt (Liu et al., 2015), and multiple-year anthropogenic sources (Lu et al., 2013). Due to the strengthened emission controls in PRD, the relocation of industries is on course for those sectors that are energy-intensive, or highly polluting, or have excess production capacity from the PRD region to NPRD areas (Chun, 2012; Yin et al., 2017) (Fig. S2). As a result, emissions in the PRD and NPRD regions have experienced substantial changes in recent years. This means that the emissions of PRD cannot fairly represent Guangdong as a whole. Although several emission inventories in GD were developed recently (Huang et al., 2015; Pan et al., 2015; Liu et al., 2017; Zhong et al., 2018), they were carried out in a single year and were limited in spatial coverage and source categories. Therefore, there is a need to develop a long-term historical emission inventory in GD using a consistent methodology and the same underlying driver data to fill the data gaps and to assist with future air pollution control measures.
In this study, we developed a multi-year anthropogenic emission inventory for SO2, NOx, PM10, PM2.5, CO, VOCs, and NH3 for the years from 2006 to 2015 using a dynamic methodology that considers economic development, technological penetration, and emission controls. The emission trends were validated by ground-based measurements and satellite observations. Based on the long-term historical inventory, the emission changes, contribution variations, possible causes of the observed air-quality improvement, and reduction potentials in 2020 in PRD and NPRD were analyzed and compared, which could provide scientific evidence for future air-quality regulations in GD. The long-term emission inventories developed in this study are also essential to evaluate the effectiveness of emission control measures and identify the dominant cause of significant air-quality change in GD.
2.1 Methods for emission estimations
In this study, emissions of seven pollutants (SO2, NOx, PM10, PM2.5, VOCs, CO, and NH3) from 13 major categories, including power plants, industrial combustion, residential combustion, on-road mobile source, non-road mobile source, dust source, industrial process source, industrial-solvent use, non-industrial-solvent use, storage and transportation, agricultural source, biomass burning, and other sources, were estimated. On the basis of Zhong et al. (2018), Pan et al. (2015), and the guidelines for the development of an air pollutant emission inventory for Chinese cities (MEPC, 2017), the 13 major categories were further broken down into 70 sub-categories to improve emission estimation. Detail categories applied in this study are listed in Table S2.
Unlike the single-year emission inventory, the estimation of a long-term emission inventory is generally more complicated since it requires the data sources, estimation method, and source category for all years to be consistent. Although top-down methods or online estimation methods based on big data can promote the estimation, obtaining the long-term activity data, such as Automatic Identification System (AIS) data for ship emission and Global Positioning System (GPS) data for the on-road mobile source, is challenging. Therefore, most studies (Streets et al., 2006; Zhang et al., 2007; Lu et al., 2013; Zheng et al., 2018) still generally applied the top-down method to develop long-term emission inventories. Following previous studies, we applied a dynamic technology-based methodology that considers economic development, technological penetration, and emission controls to estimate the anthropogenic emission trends in GD.
Except for on-road mobile and construction dust sources, emissions of most anthropogenic sources were calculated as follows:
where i, j, k, m, n, and z represent the city, the emission source, the type of fuel or product, the production technology, the year, and the control technology, respectively, A represents the activity level (such as the fuel consumption or material production), X represents the percentage of fuel or production for a sector consumed or produced by a specific technology m, EF is the unabated emission factor, is the net EF after applying the control technology, C is the penetration of the control technology z, η is the removal efficiency of the control technology z, and S and SR represent the sulfur content in fuel and the sulfur retention in ash, respectively. For fuel combustion, the EF of SO2 was estimated using the following equation:
For the on-road mobile source and construction dust, emissions were estimated by Eqs. (3) and (4), respectively. The methods from previous studies employed for the other emission sources are listed in detail in Table S2.
where i, j, and n represent the city, the vehicle type, and the year, respectively, P is the vehicle population, and VKT is annual average vehicle kilometers traveled. i and n represent the city and the year, respectively. S is the construction area, and T is the construction cycle.
An accurate representation of the annual change in activity data and emission factors is critical for estimating long-term emissions. Here, we provided a detailed description of activity data and emission factors applied in this study.
2.1.1 Activity data
Most of the activity data during 2006–2015 were obtained from officially released statistics or relevant reports. Either surrogate data or data interpolation were used to fill in the data for some sources that lack continuous and consistent long-term activity data. Notably, the activity data that specify an individual industry or power plant, defined as point data, were preferentially used, since these data generally have detailed information on the location, technical level, and control measures. Otherwise, activity data at the city level, known as areal data, were adopted as a second choice. In this study, for power plants and industrial combustion, we used a combination of point data and areal data to characterize the activity level, while the other sources all relied on areal data. The detailed data sources are summarized in Table S3. Here, we describe the processing of activity data for some major sources, e.g., industrial combustion, construction dust, and marine and on-road mobile sources.
For industrial combustion, we used the total consumption of different energy types during 2006–2015 from the GD Statistical Yearbook (GDPBS, 2007–2016) to represent the activity level of each city. Also, we used a detailed dataset from GD pollutant statistical reports to estimate the consumption value of different energy types, the averaged sulfur contents, and removal efficiencies of industrial combustion in each city. This dataset, which records the annual fuel consumption, sulfur contents, control devices, removal efficiencies, product output, and the geographic location of each plant, contains about 85 % of the plants in GD and covers the years of 2006, 2010, 2012, 2014, and 2015. For the years that lack a detailed dataset, the averaged sulfur contents and removal efficiencies were estimated by linear interpolation and emission control policy.
For the construction dust source, we used the total annual construction area and construction cycle time to represent the activity level. The construction area data were derived from the GD city statistical yearbook (GDPCSY, 2007–2016), and the construction cycle time was determined by the time required for different construction phases, i.e., earthwork excavation, foundations, earthwork backfill, and general construction. Considering the effect of rainfall in suppressing the dust source, we revised the construction cycle time by combining our previous study (Yang, 2014) with rainfall information for each year obtained from the GD Meteorological Service (GDMS, 2007–2016).
Regarding marine sources, the characterization of activity level was based on heavy and diesel fuel consumption. However, it is challenging to acquire detailed consumption of various fuel types directly. Thus, we used the method described in Li et al. (2018) to convert the cargo volumes and transport distances of major navigation routes into fuel consumption data via fuel consumption rates. Fuel consumption rates were taken from the IMO report (IMO, 2009). The cargo volumes in each city were obtained from the GD Statistical Yearbooks (GDPBS, 2007–2016). Transport distances of major navigation route data were measured by the historical AIS-based digital map.
For the on-road mobile source, population data of different vehicle types (i.e., passenger trucks, buses, taxis, and motorcycles), and the gross weight (heavy and light duty) from the 2006–2015 statistical yearbooks, and annual average vehicle kilometers traveled from a field survey of eight cities and 111 roads by types (i.e., arterial road, secondary arterial road, and branch) in GD were used to characterize the annual change in activity level. We further divided the vehicle type into diesel and gasoline vehicles to obtain a more accurate estimate, based on the vehicle ratios (diesel∕gasoline) from our previous study (Che et al., 2009) in 2006, and the field survey covered eight cities in the years of 2010, 2012, 2014, and 2015. The vehicle ratios in other years (2007–2009, 2011, and 2013) were estimated using an interpolation method.
2.1.2 Emission factors (EFs)
EFs could have changed with the implementation of emission controls in GD during 2006–2015, which involve technological penetration and evolution. To deal with that possibility, we developed a dynamic method to reflect the response of EFs to control measures and technological penetration. First, we established the unabated EF of each source to represent what the emission level would have been without any treatment. The unabated EFs for most emission sources of various pollutants (i.e., NOx, PM10, PM2.5, VOCs, CO, and NH3) used in this study are listed in Tables S4–S9, and are based mainly on the latest research results and values recommended in related manuals of air-pollutant emission coefficients. Next, we estimated the net EFs of each source according to the corresponding processing technologies, control technologies, and removal efficiencies that might vary with years. In this study, we applied the dynamic method to all emission sources. In the following subsection, we mainly describe their application to major sources that have received intensive control measures in the past decade, including power plants, industrial combustion, VOC-related sources, and on-road mobile sources.
The net EFs of SO2 for industries and power plants in GD were determined based on removal efficiencies and fuel sulfur content. The annual removal efficiencies and sulfur content in 2006, 2010, 2012, 2014, and 2015 were obtained from GD pollutant statistical reports (GDPSR, 2006, 2010, 2012, 2014, 2015) for industries; those parameters for power plants in 2006–2014 were obtained from power-plant reports (China Southern Power Grid (CSPG), 2006–2014). For years without these documented data, an interpolation method that considers newly released regulations of SO2 emission controls and expert judgment were used to estimate the removal efficiency and sulfur contents (2007–2009, 2011, and 2013 for industries and 2015 for power plants). For instance, the sulfur content of coal and oil in industrial sources can be estimated as <0.7 % and 0.8 %, respectively, according to the Guangdong industrial boiler pollution remediation program (2012–2015) released in 2012.
For VOC-related sources, such as industrial-solvent use, non-industrial-solvent use, and industrial process sources, the net EFs of VOCs were determined based on the installation rate of VOC control technologies and the removal efficiencies, which were acquired by an on-site investigation of VOC-related industries in GD. Additionally, the new VOC emission standards were also used to determine in which year VOC control technologies were implemented. For example, emission standards for furniture surface coating and shoemaking were implemented in 2010 (Emission standard of volatile organic compounds for furniture manufacturing operations (DB44/814-2010), Emission standard of volatile organic compounds for shoe-making industry (DB44/817-2010)). Thus, we estimated the net VOC EFs for furniture surface coating and shoemaking with the consideration of VOC removal efficacies since 2010. For the vehicular EFs, we used the same method employed in our previous study (Lu et al., 2013). The vehicular EFs were calculated based on the 2007 International Vehicle Emissions (IVE) model (International Sustainable Systems Research Center (ISSRC), 2008), while the EFs for other years were derived from 2007-based EFs in consideration of emission standards, fuel standards, and vehicle lifespans.
2.2 Data for validation
In this study, satellite observations, including SO2, NO2, and AOD column, and ground-level observations (SO2, NO2, and PM2.5 concentrations) in PRD were used to evaluate the long-term total emission in the GD area. The SO2 column amount (OMSO2e v003) and the NO2 tropospheric column (OMNO2d v003) were retrieved from the Ozone Monitoring Instrument (OMI) with a spatial resolution of 0.25∘ × 0.25∘ (available at https://giovanni.gsfc.nasa.gov/giovanni/, last access: 3 September 2019). Aerosol optical depth (AOD) data were taken from the Moderate-Resolution Imaging Spectroradiometer (MODIS) aerosol product MOD04 with a high resolution of 10 km (available at https://ladsweb.modaps.eosdis.nasa.gov/, last access: 3 September 2019). The ground-level observations in PRD during 2006–2015 were obtained from the PRD Regional Air Quality Monitoring Network (RAQMN) (GDEMC et al., 2007–2016). The RAQMN, which came into operation at the end of 2005 and has provided accurate air-quality data to local governments and the public, was adopted because of the high reliability of the operating procedures on quality assurance and quality controls (QA/QC). We also collected the ground-level observations averaged over the GD from annual reports of the Guangdong provincial environment published by the department of environmental protection of Guangdong Province. However, these data are less representative since the ground-level stations in NPRD are much sparser and the QA/QC procedures are not well implemented in NPRD.
We focused on the evaluation of emission trends. Therefore, we mainly compared annual observations averaged over the PRD/GD region with annual emissions in PRD/GD. Also, emissions and satellite observations in the PRD and NPRD region were compared. Since emissions were mainly concentrated in urban areas, where ground-level observations in RAQMN are located, the sparsity of ground-level observations was ignored in the evaluation.
3.1 Overall emission trends
The overall emission trends of SO2, NOx, PM10, PM2.5, VOCs, CO, and NH3 in GD during 2006–2015 are presented in Fig. 1. From 2006 to 2015, anthropogenic emissions decreased by 48 % for SO2, 0.5 % for NOx, 16 % for PM2.5, and 22 % for PM10, but increased for CO, NH3, and VOCs, by 13 %, 3 %, and 33 %, respectively. Specifically, SO2 emissions fell steadily during 2006–2015 due to the strict controls on SO2 emissions implemented in the 11th Five Year Plan (FYP) (2006–2010) and 12th FYP (2011–2015). These two FYP all required the total national SO2 emission to be cut by 15 %, relative to the 2005 and 2010 levels, respectively. NOx emissions overall showed an upward trend in the early period, reaching a peak in 2011. After the implementation of the Planning for Guangdong province environmental protection and ecological construction in 12th FYP (PGGP, 2011) in 2011, in which NOx emission caps of all industrial sectors are proposed, NOx emissions decreased, declining by 9 % in 2015. The PM10 and PM2.5 emissions showed an increasing trend during 2006–2009 but then decreased steadily. Similarly, CO emissions showed a small rise during 2006–2013, followed by a sharp decline. NH3 emissions changed a little, while VOC emissions steadily increased over the 2006–2015 period, mainly fueled by the absence of effective emission-control measures.
Although emissions of SO2, NOx, PM, and CO declined in recent years, the per capita GDP, fuel consumption, and vehicle population in GD, which account for most anthropogenic pollution activity, saw growth from 2006 to 2015, as shown in Fig. 1. From 2006 to 2015, the per capita GDP and vehicle population significantly increased, by 135 % and 66 %, respectively. Obviously, anthropogenic emissions in GD were decoupling from economic and energy consumption growth. This means that the emission regulations and control measures enacted in GD have alleviated emissions despite the growth of economic activity. For instance, NOx emissions are closely related to fuel consumptions because a large proportion of their emissions are from industries or power plants that consume a great deal of fuel. However, the trends of NOx emissions and fuel consumption have deviated from each other since 2011, when low NOx-combustion (LNB) control measures and flue-gas denitrification technology, i.e., selective catalytic reduction (SCR) and selective non-catalytic reduction (SNCR), in power plants were enacted.
We have also compared emission trends in PRD and NPRD (Fig. S3). Since emissions in GD were mainly concentrated in the PRD region, emission trends in PRD were similar to those in GD, except NOx emissions, which started off steady until 2012 and then fell slightly. Compared with 2006, the 2015 emissions of SO2, NOx, PM2.5, and PM10 in PRD decreased by 63 %, 14 %, 35 %, and 27 %, respectively, while CO and VOC emissions increased by 2 % and 35 %, respectively. In NPRD, SO2, NOx, PM2.5, and PM10 emission trends differed from those in PRD. Compared with 2006, the 2015 emissions of SO2, PM2.5, and PM10 in NPRD decreased by only 8 %, 8 %, and 5 %, respectively, but emissions of VOCs, NH3, CO, and NOx significantly increased by 30 %, 10 %, 31 %, and 29 %, respectively. The discrepancy of emission trends between PRD and NPRD increased because these two regions achieved different levels of progress on air-quality management in the past decade. Overall, most previous control strategies still focused on PRD (Table S1). In Table S1, all of these 11 control strategies involve PRD, while only 6 of them cover NPRD. Moreover, due to the policies to “vacate the cage and change birds” (in Chinese, Teng Long Huan Niao) initiated by the Guangdong provincial government in 2008 (Yang, 2012), many low-tech industries in PRD were relocated to NPRD. As a result, emissions of NPRD became more and more obvious in GD. For instance, the contribution of NPRD to SO2 emissions in GD had increased from 27.1 % in 2006 to 48.2 % in 2015 (Table 1). For NOx, PM2.5, and PM10 emissions, the proportions increased from 31.6 % to 40.6 %, from 49.5 % to 56.0 %, and from 50.3 % to 54.0 %, respectively. Detailed emission evolutions and the corresponding causes are discussed in Sect. 3.3.
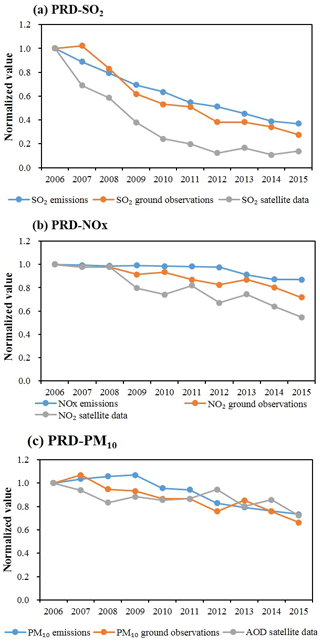
Figure 2Comparison of emission trends of (a) SO2, (b) NOx, and (c) PM10 with ground-level/satellite measurements in PRD from 2006 to 2015. (All data are normalized to the year 2006.)
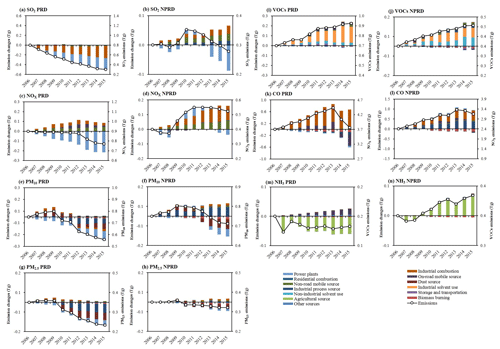
Figure 3Emission evolutions by source categories and year in PRD and NPRD for (a)–(b) SO2, (c)–(d) NOx, (e)–(f) PM10, (g)–(h) PM2.5, (i)–(j) VOCs, (k)–(l) CO, and (m)–(n) NH3 from 2006 to 2015. Here, the additional emissions compared to the level in 2006 are presented using a bar graph (left axis). The total annual emissions were also shown using a point plot (right axis).
3.2 Validation of emission trends
In this section, we validated emission trends in PRD using ground observations and satellite data (Fig. 2). The comparison of emission trends with measurements in GD is also presented in Fig. S4. In general, these two data sources are consistent for emission trends of SO2, NOx, and PM10. During 2006–2015, SO2 emissions in PRD decreased by 63 % (emission trends in PRD are shown in Fig. 3), slightly less than the 68 % and 86 % observed in ground-level and satellite data, respectively. NOx emissions and observations all showed a declining trend during 2006–2015. For PM10 emissions, the declining trend also closely followed the fluctuant downward trend of ambient PM2.5 concentrations and AOD. The fluctuations of observations are associated with PM2.5 formation influenced by annual variations of meteorology.
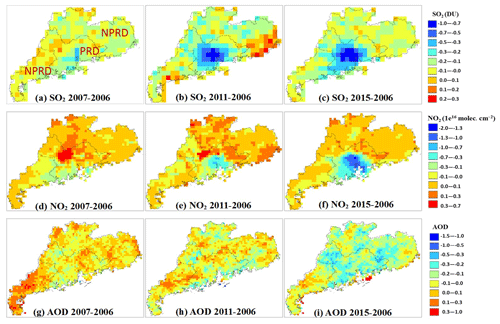
Figure 4Spatial distributions of satellite observation changes over GD during 2007–2006, 2011–2006, and 2015–2006 for (a)–(c) SO2, (d)–(f) NO2, and (g)–(i) AOD. The blue color in the legends represents a decline in SO2 concentrations/NO2 concentrations/AOD, and the red color denotes an increase. The central zone inside the inner black line presents the PRD region, and outside presents the NPRD region.
The change in the spatial variation of emissions in GD was also evaluated using satellite measurements. Here, the relative change in column concentrations of SO2, NO2, and AOD in 2007, 2011, and 2015 to 2006 are illustrated in Fig. 4. The annual column concentrations during 2006–2015 are all displayed in Fig. S5. Overall, the spatial patterns of the satellite measurements also reveal the different emission trends between PRD and NPRD. For example, SO2 column concentration in PRD decreased by 71 % from 2007 to 2011, but in NPRD increased by 26 %. This agrees with the emission trends, in which SO2 emissions in PRD decreased by 39 % while those in NPRD increased by 15 %. From 2011 to 2015, SO2 column concentration decreased by 31 % and 42 % in PRD and NPRD, respectively, and SO2 emissions decreased by 32 % and 20 % in PRD and NPRD, respectively. From 2007 to 2011, NO2 column concentration decreased by 16 % in PRD but increased by 16 % in NPRD. These trends also coincided with emission changes where NOx emissions in PRD decreased by 13 % but increased by 36 % in NPRD. From 2011 to 2015, NO2 column concentration and NOx emissions both decreased in PRD and NPRD. AOD displayed a decrease of approximately 23 % in PRD and NPRD from 2007 to 2015. A similar pattern is also found in PM2.5 emission trends, with decreases of 35 % in PRD and 9 % in NPRD.
Overall, the above-mentioned validations using satellite observations and ground measurements demonstrate that emission trends of SO2, NOx, and PM estimated in this study are reliable.
3.3 Evolution of source emissions in Guangdong Province
To further understand the evolution of emissions in GD, we estimated the changes in anthropogenic emissions by emission category in PRD and NPRD during 2006–2015. Here, we examine the PRD and NPRD regions separately since these two regions may have experienced diverse emission evolutions due to their different levels of progress on air-quality management. The annual source contributions during 2006–2015 are also presented in Fig. 5 (GD) and Fig. S6 (PRD and NPRD).
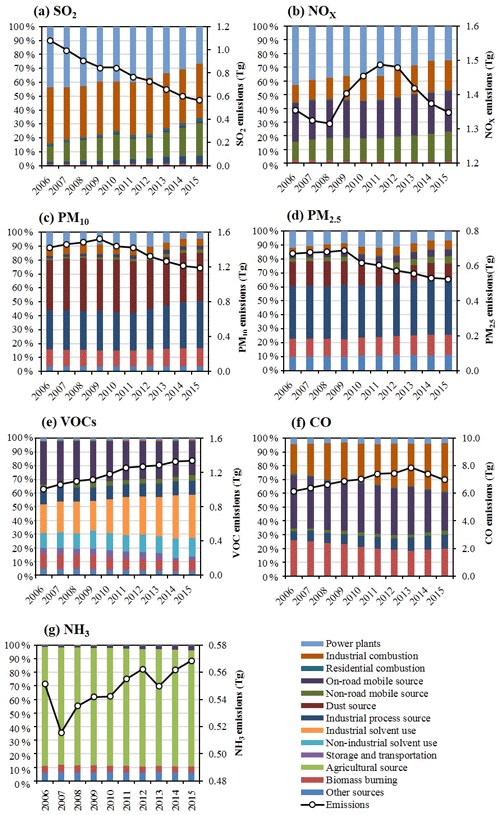
Figure 5Source emission evolutions in Guangdong Province for (a) SO2, (b) NOx, (c) PM10, (d) PM2.5, (e) VOCs, (f) CO, and (g) NH3 from 2006 to 2015. The stacked column graphs show the emission contributions by source category and year (left axis). The point plots show the total annual emissions in Guangdong Province (right axis).
3.3.1 SO2
In PRD, SO2 emissions steadily declined, from 0.788 Tg in 2006 to 0.292 Tg in 2015. The decline is dominated by power plants and industrial combustion, accounting for 50.0 % and 46.8 % of the total decrease, respectively (Fig. 3a). The decline of SO2 emissions from power plants and industrial combustion is highly associated with the control measures enacted during the 11th FYP and 12th FYP. In response to the SO2 emission cap in 11th FYP, Guangdong Province required the elimination of small thermal power units with high energy consumption and outdated combustion technology in 2007. By the end of 2011, 12.2 million kilowatts of small thermal power units had been eliminated during the 11th FYP. In addition, the action plan for air pollution prevention and control in Guangdong province (2014–2017) (PGGP, 2014) released in the 12th FYP further strengthened the emission control of power plants. As a result, the flue-gas desulfurization (FGD) penetration in coal-fired power plants rose to 87 % in 2013. For the industrial combustion, its fuel consumption in PRD decreased by 47.5 % during 2006–2015, which can be explained by energy structural adjustment regulations, including the “total amount control of coals” and “changing fuel from coal to natural gas” control measures (Fig. S7). The detailed regulations on energy structural adjustment are summarized in Table S1.
In NPRD, by contrast, SO2 emission increased until 2010, and then saw a downturn. Before 2010, the SO2 emission growth was mainly associated with the increase in industrial combustion and non-road mobile sources (Fig. 3b). These two sources still maintained a slight rise after 2010, but their increased emissions were offset by a plunge of emissions from power plants. To reveal the cause of emission growth from industrial combustion in NPRD, we compared the standard fuel-consumption trends from industrial combustion in PRD and NPRD (Fig. 6). In PRD, the fuel consumption from industrial combustion in PRD steadily dropped by 47 % during 2006–2015, with an average annual descending rate of 6.9 %. In NPRD, by contrast, the fuel consumption increased by 99 %. In fact, the average annual growth rate of fuel consumption in NPRD was only 3.2 % before 2010, but after that, the increase accelerated, with an average annual growth rate of 11.9 % during 2011–2015. Particularly, the growth rate peaked in 2011 (17.1 %) and 2012 (14.5 %). Meanwhile, the descending rates of fuel consumption in PRD also reached their peak in 2011 (9.9 %) and 2012 (11.4 %), when the shift of industries was implemented. This fact indicates the existence of an emission leak from PRD to NPRD due to the policy to “vacate the cage and change birds” that brought many energy-intensive industries from PRD to NPRD. Statistical data showed that the NPRD region had undertaken 33 industrial parks that migrated from PRD, as shown in Table S10 (GDEI, 2014). Consequently, the industrial shift might, in turn, promote the emission of power plants in NPRD to some extent.
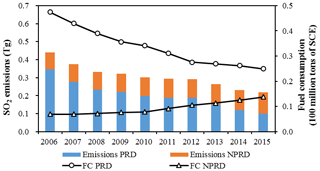
Figure 6Trends of SO2 emissions (left axis) and fuel consumption (right axis) from industrial combustion from 2006 to 2015. SCE represents standard coal equivalent; FC represents fuel consumption (i.e., coal, fuel oil, coke, and natural gas), and all of the FC data are normalized to the year 2006.
The source contribution to SO2 emissions in GD also changed (Fig. 5a). In GD, the contribution of power plants dropped from 43 % to 27 %. By contrast, the contribution of industrial combustion remained stable, in the range of 36 % to 41 %. Therefore, industrial combustion replaced power plants and became the largest SO2 emission source in GD. The PRD and NPRD regions also had similar changes (Fig. S6a–b). Note that the contribution of the non-road mobile source to SO2 emissions increased in both PRD (from 13 % to 29 %) and NPRD (from 8 % to 17 %), due to the absence of effective emission control measures. Also, the contribution of the industrial process source slightly increased in NPRD, from 5 % to 11 %.
3.3.2 NOx
As with SO2 emissions, the evolution of NOx emissions also differed between PRD and NPRD. In PRD, NOx emissions dropped overall from 0.926 Tg in 2006 to 0.797 Tg in 2015, especially after 2011, when NOx was put on the list of pollutant controls, and NOx emissions saw a noticeable downturn (Fig. 3c). Power plants were the primary sources leading to emission reduction. This was expected because the local government had released the implementation plan for nitrogen reduction and denitrification project for power plants in Guangdong province to respond to the reduction target of NOx emissions in the 12th FYP (2011–2015). As a result, a large number of power plants have installed NOx removal equipment since 2011. By the end of 2015, NOx emissions from power plants had declined by 54.0 % compared with 2006 due to the increasing installment of the flue-gas denitration facility since 2011. However, a part of the NOx emission reductions from power plants in PRD was canceled out by the emission growth from non-road mobile sources, which increased by 65.7 % during 2006–2015. Despite the intensive control measures for urban vehicle exhaust, NOx emissions from on-road mobile sources still kept a slowly growing trend, indicating that these control measures were unlikely to offset the increasing vehicle population, which increased by 88 % during 2006–2015. Consequently, on-road mobile sources (growing from 32 % in 2006 to 38 % in 2015) overtook power plants (growing from 43 % to 23 %) as the largest contributor to NOx emissions in PRD since 2010 (Fig. S6c).
Unlike in PRD, NOx emissions in NPRD rebounded in 2008 and grew sharply until 2012. The emission changes were mainly from industrial combustion, non-road mobile sources, and power plants (Fig. 3d). Among these three sources, NOx emissions from industrial combustion and non-road mobile sources both showed an upward trend, increasing by 159 % and 84 %, respectively, during 2006–2015. These trends can be explained by the shift of energy-intensive industries from PRD to NPRD and the absence of catch-up emission controls for industries and non-road mobile sources. In fact, most of the control measures for non-road mobile sources were released after 2015. Since 2011, possibly due to the implementation of denitrification technology, NOx emissions from power plants steadily went down and offset the slight increase in emissions from industrial combustion and non-road mobile sources. Consequently, the contribution of power plants to the total NOx emissions in NPRD, which was once a large contributor, decreased from 44 % in 2006 to 28 % in 2015. By contrast, contributions of industrial combustion and non-road mobile sources increased from 14 % to 29 % and from 16 % to 23 %, respectively (Fig. S6d).
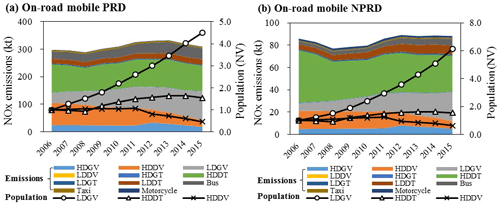
Figure 7Trends of NOx emissions from the on-road mobile source (left axis) and its activity data (right axis) from 2006 to 2015 in (a) PRD and (b) NPRD. Emissions are presented by sub-category in the on-road mobile source.
The on-road mobile source was also a major contributor to NOx emissions in GD (Fig. 5b). Although the total NOx emissions of on-road mobile sources changed slightly in 2006–2015, their sectoral contribution showed significant change, especially in PRD (Fig. S6c). Here, we further analyze the trends of NOx emissions from on-road mobile sources and the vehicle population from 2006 to 2015 for PRD (Fig. 7a) and NPRD (Fig. 7b). Overall, on-road mobile NOx emissions in PRD were approximately 3 times higher than those in NPRD, but their trends were similar. Although the heavy-duty diesel truck (HDDT) population slightly grew until 2014 in both PRD and NPRD, NOx emissions from HDDT still dropped by 10.0 % and 30.8 % during 2006–2015, respectively, which was closely related to the improvement in vehicular emission and fuel standards. During the study period, the emission standard for vehicles improved from China III (2008) to China IV (2013), while the oil standard improved from China III (2009) to China V (2014). The proportion of China III HDDT vehicles increased by over 50 % in both PRD and NPRD (Liu et al., 2017). NOx emissions from heavy-duty diesel vehicles (HDDVs) also dropped, partly due to the decreased HDDV vehicle population. Unlike the HDDT and HDDV, the population of light-duty gasoline vehicle (LDGV) increased significantly, by factors of 5 and 6 in PRD and NPRD, respectively. This inevitably led to the obvious growth of NOx emissions from LGDV, despite the enhancement of the vehicle emission standard and weed-out of yellow-label vehicles (PGGP, 2009).
Based on the above analysis, it can be concluded that the NOx emission trend in GD is dominated by the decline of power plants in PRD and the increase in non-road mobile sources and industrial combustion in PRD and NPRD. Particularly, the contribution of non-road mobile sources and industrial combustion to NOx emissions in GD increased from 13 % in 2006 to 23 % in 2015 and from 12 % to 20 %, respectively (Fig. 5b), indicating that these two sources should receive more attention in future emission-control measures, especially industrial combustion in NPRD. Regarding on-road mobile sources, the largest contributor to NOx emissions in PRD, LDGVs, should require more attention in the future.
3.3.3 PM10∕PM2.5
As shown in Fig. 3e–h, the main sectoral changes between PM10 and PM2.5 emissions were somewhat similar to each other. Therefore, we focused mainly on the analysis of PM10 emissions. PM10 emissions in PRD and NPRD showed similar variations regarding emission trends and source contributions. They both topped out in 2009 and then decreased monotonically. Compared with 2006, PM10 emissions in 2015 dropped by 27 % and 6 % in PRD and NPRD, respectively. Dust source, power plants, and industrial process source were the major contributors to the change in PM10 emissions in PRD and NPRD. However, emission trends of these three sources in PRD and NPRD were slightly different, particularly the industrial process source. In PRD, PM10 emissions from the industrial process source steadily declined after 2010, while in NPRD, PM10 emissions from the industrial process source kept an upward trend during 2006–2015. One possible reason for the difference is that control measures for PM10 emissions in PRD are stricter than those in NPRD. In 2010, the local government issued two air action plans and regulated emission control measures for non-metallic mineral industries (Table S1), one of the major PM2.5 and PM10 emission sources in GD. For instance, all cement plants, glass manufacturers, and other non-metallic mineral industries with still outdated production processes were eliminated by the end of 2012. However, these control measures were limited to the PRD region. In PRD and NPRD, the dust source increased during 2006–2010 and showed a decrease during 2011–2015. The downturn in the later years was due to the implementation of emission control technologies of the dust source in response to the release of the clean air action plan for the Pearl River Delta in Guangdong province in 2010.
As emissions from the industrial process source, dust source, and power plants changed dramatically, the major sources that contributed to PM2.5 and PM10 emissions also changed accordingly (Figs. 5c–d, S6e–h). For PM2.5 emissions in GD, contributions from dust source and power plants declined slightly, from 17 % in 2006 to 11 % in 2015, and from 12 % to 7 %, respectively. The contribution from the industrial process source, the largest contributor, also slightly decreased from 38 % to 33 %. For PM10 emissions in GD, the contribution of the industrial process source increased, from 28 % to 34 %. Particularly in NPRD, it replaced the dust source as the largest contributor since 2012. In PRD, fugitive dust was still the largest PM10 emission source. Based on the above-mentioned analysis, PM emission controls for the dust source, especially in PRD, and for the industrial process source, especially in NPRD, should be a priority of the agenda in the next stage of emission controls. Also, PM2.5 emissions from cooking cannot be neglected in GD; this is because the removal of cooking oil fumes from homes and restaurants was not strictly enforced, although some regulations and emission standards regarding cooking emissions were enacted gradually.
3.3.4 VOCs
As shown in Fig. 3i–j, the sectoral changes in VOC emissions in PRD and NPRD were similar. The total emissions in these two regions both showed a rising trend during 2006–2015, increasing by 35 % and 30 % in PRD and NPRD, respectively. The steady increase mainly originated from the growth of industrial-solvent use and non-industrial-solvent use, whose emissions in GD, respectively, increased by 99 % and 69 % during 2006–2015 (Fig. 5e). Industrial-solvent use was a large increasing source, especially in PRD, where most industrial sources are concentrated. This is expected because solvent use required by industrial sources was growing but control measures were insufficient. Several VOC control technologies had been adopted since 2010. For instance, the use of low VOC-containing raw materials for printing, shoemaking, furniture manufacturing, and other industries was first proposed at the clean air action plan for the Pearl River Delta in Guangdong province in 2010. Although these measures slowed an increasing trend of VOC emissions in PRD (VOC emissions from industrial-solvent use in PRD increased by 18 % during 2006–2010, while they increased by 6 % during 2011–2015), the control efficiencies were still low, and only 40 % of VOC-emitting industries had removal equipment in GD in 2014 according to a field survey (Wang et al., 2019).
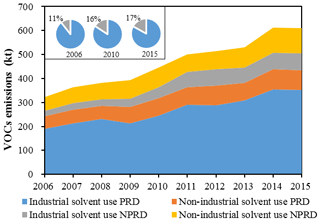
Figure 8Trends of VOC emissions from solvent use by year. The blue, orange, gray, and yellow colors denote industrial source in PRD, non-industrial source in PRD, industrial source in NPRD, and non-industrial source in NPRD, respectively. The proportions of VOC emissions from industrial-solvent use in PRD to the total counterpart in GD are also shown using the pie graph in the top left corner.
The contribution from the industrial-solvent use in NPRD increased from 11 % in 2006 to 17 % in 2015, partly driven by the electronics coating (0.5 % of industrial solvent in 2008 rise to 17 % in 2011) and appliance coating (14 % to 30 %) (Fig. 8). This coincided with the Yang and Mao (2014) study that electronic equipment and appliance manufacturing accounted for 23 % of 457 transferred enterprises until 2011. The increasing VOC emissions from industrial-solvent use made it become the largest contributor to VOC emissions in GD in 2015 (Fig. 5e), with a percentage of 32 %. Therefore, the implementation of policy and upgrade of control technologies are still required to reduce VOC emissions. In NPRD, non-industrial-solvent use was also a major contributor to the increase in VOC emissions. In particular, it became the largest contributor to VOC emissions in NPRD in 2015, with a percentage of 22 %, slightly larger than the on-road mobile source (21 %) (Fig. S6j).
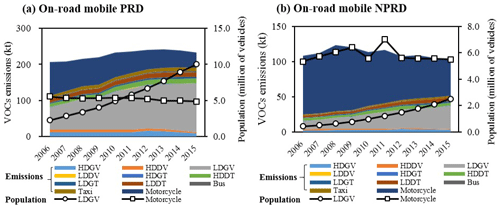
Figure 9Trends of VOC emissions from the on-road mobile source (left axis) and its activity data (right axis) from 2006 to 2015 in (a) PRD and (b) NPRD. Emissions are presented by sub-category in the on-road mobile source.
Since the on-road mobile source was also a major contributor to VOC emissions, the evolution of their VOC emissions is also discussed here (Fig. 9). In both PRD and NPRD, VOC emissions from motorcycles, the largest contributors to VOC emissions from on-road mobile sources, went down in the past decade due to the relatively strict ban on motorcycles. They decreased by 55 % and 38 % in PRD and NPRD, respectively. By contrast, VOC emissions from LDGV increased by 118 % and 197 % in PRD and NPRD, respectively, likely due to the upsurge of the LDGV vehicle population. Particularly in PRD, LDGVs became the largest contributor to vehicle-related VOC emissions since 2008, which might also happen in NPRD according to the current trend.
3.3.5 CO/NH3
As shown in Fig. 3k–l, CO emissions in PRD and NPRD both increased steadily during 2006–2013 and then decreased after 2013. However, the sectoral changes were different in these two regions. In PRD, the growth of CO emissions during 2006–2013 was mainly attributed to the industrial combustion and on-road mobile source, while in NPRD, it was from the industrial combustion and industrial process source. The difference exists because the on-road mobile source was primarily concentrated in PRD, while the iron and steel sectors, the largest CO emitters among industrial process sources, were located mainly in NPRD. Notably, production of the iron and steel sectors soared during 2006–2015, increasing by almost 95 % in GD (GDPBS, 2007–2016), but emission controls fell behind. As to the decline of CO emissions during 2013–2015 in PRD, the on-road mobile source was the major reason. By contrast, the slight downturn in NPRD was mostly due to declining emissions from the on-road mobile source and biomass burning. All these sectoral changes made industrial combustion (35 % in 2015) become the largest contributor to CO emissions in GD (Fig. 5f). In NPRD, the contribution of the industrial process source also increased. In contrast, the contribution of the on-road mobile source to CO emissions in NPRD decreased by 19 % in 2015 compared with that in 2006.
As shown in Fig. 5g and Fig. 3m–n, agricultural sources constituted most to the change in NH3 emissions, as they accounted for 86 %–87 % of the total NH3 emissions in GD. However, their annual changes were different in PRD and NPRD (Fig. 3m–n). In NPRD, NH3 emissions by agricultural sources increased by 11 % during 2006–2015, partly as the result of the growth of fertilizing and livestock to meet the increasing demand for food. Another reason was the absence of effective emission controls on agricultural sources in China. By contrast, in PRD, NH3 emissions by agricultural sources remained stable.
3.4 Evaluation of emission control measures and policy implications
To evaluate the efficiencies of the control measures enacted in GD and to provide implications for future policies, we decomposed the emission changes into two categories: (1) changes resulting from change in activity level (activity-driven emission) in the absence of control measures and (2) changes due to the implementation of pollution controls (control-driven emission reduction) (Zheng et al., 2018). We estimated the unabated emissions if pollution control had been frozen at the 2006 level. In other words, we assumed that there were no new control measures adopted since 2006. Then the control-driven emission reduction was estimated by comparing the unabated emissions and the actual emissions, and the activity-driven emission was estimated by calculating the annual changes in unabated emissions. Also, we projected the actual emission to 2020 to help understand the potential for more emission control. Here, the planned emission controls before 2020 were assumed to be completely implemented in 2020. These related regulations for SO2, NOx, PM10, and VOC emissions controls are summarized in Table S1. The control-driven and activity-driven emissions of SO2, NOx, PM10, and VOCs in 2007, 2009, 2011, 2013, and 2015 in addition to the predicted emissions in 2020 in PRD and NPRD are presented in Fig. 10.
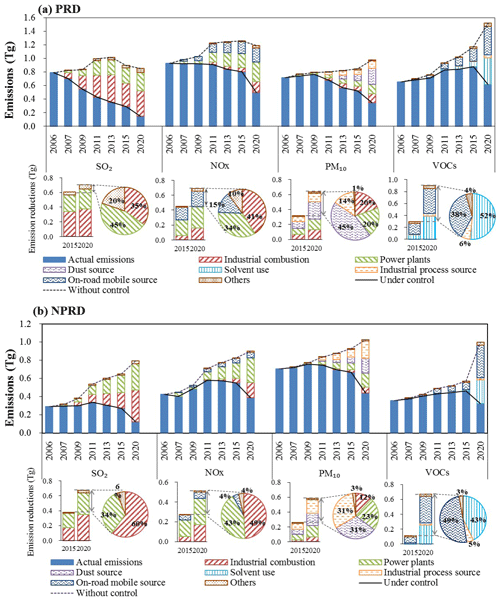
Figure 10Control- and activity-driven emissions of SO2, NOx, PM10, and VOCs in 2007, 2009, 2011, 2013, and 2015 in addition to emission predictions in 2020 for the (a) PRD and (b) NPRD. Solvent use here includes industrial-solvent use and non-industrial-solvent use. The solid black line and the solid blue bar denote the actual emissions we estimated under control (i.e., the results under the interaction of control- and activity-driven emissions; if control-driven emission dominated, the actual emissions would drop, and vice versa), and the dotted black line denotes the hypothetical emissions without control (i.e., activity-driven emission, if no new control measures were adopted after 2006). The non-solid chromatic bars and pies illustrate the emission reductions for multiple sources (i.e., control-driven emission). The dotted box represents extra emission reductions in 2020 compared to 2015.
3.4.1 SO2
During 2007–2011, the decline of SO2 emissions in PRD was driven by emission controls. The control-driven SO2 emission reductions in PRD dramatically grew from 0.120 Tg in 2007 to 0.568 Tg in 2011 (Fig. 10a), mainly attributable to stringent SO2 emission control regulations on industrial combustion and power plants – e.g., shutting down small coal-fired thermal power units, phasing out small boilers, installing flue-gas desulfurization (FDG) equipment and limiting the sulfur contents of fuel. SO2 emission reductions from industrial combustion and power plants account for 57 %–75 % and 25 %–37 % of the total emission reductions, respectively. The activity-driven emissions increased by 0.178 Tg during 2007–2011, but their increments were far less than the control-driven emission reductions. The control-driven emission reductions flattened out in recent years. During 2013–2015, the control-driven emission reductions only increased by 4 %, which owes much to the effectiveness of SO2 emission controls in earlier years and the shrinking of control measures in recent years. Nonetheless, SO2 emissions in PRD still steadily declined, partly due to the decrease in activity-driven emissions. By contrast, activity-driven emissions in NPRD kept rising, similar to Fig. 6, which also is associated with the transfer of energy-intensive industries from PRD to NPRD (Fig. 10b). Even so, the control-driven emission reductions dramatically increased and outweighed the activity-driven emissions since 2011, when stricter control measures were implemented. Similar to the situation in PRD, pollution control-driven emission reductions in NPRD were mainly attributed to industrial combustion and power plants.
Although SO2 emissions dramatically decreased since 2006, there is potential for further reduction in 2020. On the basis of control-driven emission reductions in 2015, SO2 emission reduction potentials in PRD and NPRD in 2020 are projected to be 0.10 Tg (34 % of the total SO2 emissions in 2015) and 0.29 Tg (approximately equal to the total SO2 emissions in 2015), respectively. These reductions can be achieved by technical innovations, including ultra-low-emission measures in power plants, a series of actions regarding boiler management, sulfur content controls in fuels, and flue-gas desulfurization in industries. Most of these emission reductions are from industrial combustion and power plants. Particularly for NPRD, 60 % of the reductions can come from industrial combustion, more than that in PRD. This is because SO2 removal efficiencies in industries are relatively low in NPRD. SO2 emission contribution from a non-road mobile source in GD previously presented an increasing trend (Fig. 5a). This reminds us that a non-road mobile source still has a high potential for SO2 emission reduction. In PRD, it could account for approximately 20 % of the total SO2 emission reductions in 2020. Thus, future measures should be focused on industrial combustion and non-road mobile sources for controlling SO2 and NOx emissions (NOx also presented a similar result in the subsequent analysis).
3.4.2 NOx
In PRD, the decline of NOx emissions was driven by emission controls and was significantly enhanced in 2011 when the 12th Five Year Plan was enacted in GD, including the application of technology for flue-gas denitrification and low NOx combustion (LNB) in industries and power plants (Kurokawa et al., 2013), the elimination of yellow-label cars, and progressive advancements in vehicle emission and fuel standards. These mitigation measures yielded 0.315 Tg NOx emission reductions in 2011 and offset the growth of activity-driven emissions. During 2007–2015, power plants and on-road mobile sources were the two major contributors, accounting for 34 %–59 % and 38 %–60 % of the total control-driven emission reductions, respectively. Industrial combustion also contributed 2 %–14 % of the total control-driven emission reductions. Unlike in PRD, NPRD's new clean-air actions mostly focused on power plants, and these measures were not stringent enough to cover the growth of NOx emissions before 2011. After 2011, the control measures of NOx emissions from power plants in NPRD were strengthened, leading to a significant increase in control-driven NOx emission reductions. Consequently, the total NOx emissions slightly declined. Apart from power plants, on-road mobile sources and industrial combustion also partly contributed to the control-driven emission reductions in NPRD in recent years.
On the basis of control-driven emission reductions in 2015, NOx emissions in 2020 could be further reduced by 0.24 Tg in both PRD (30 % of the total NOx emissions in 2015) and NPRD (43 % of the total NOx emissions in 2015). Most of these projected reductions could come from industrial combustion and power plants as a result of the implementation of stricter regulations, e.g., ultra-low emissions for power plants, boiler management, and flue-gas denitrification for industries. In PRD, on-road and non-road mobile sources also have a relatively high potential for NOx emission reduction. Particularly, an on-road mobile source, especially LDGV, requires more effective control measures. Although current control measures have alleviated the number of vehicle emissions in recent decades, they still cannot cover the increased emissions driven by the rapid growth in the vehicle population, as shown in Fig. 3c. Further reduction of on-road mobile NOx emissions can be achieved by the implementation of control regulations.
3.4.3 PM10
The activity-driven emissions of PM10 in PRD and NPRD both steadily increased during 2006–2015 due to growth in activity. From 2006 to 2015, the gross industrial output value increased by 48 % (GDPBS, 2007–2016), but control measures were not implemented until 2009, leading to a slight increase in PM10 emissions during 2006–2009. After 2009, the installation of dust removal equipment dramatically increased with the stricter implementation of PM control measures, such as special requirements limiting soot emission in power plants, boiler management with smaller capacity, and a series of pollution controls for non-metallic mineral industries. These measures boost the control-driven emission reductions, which can counterbalance the growth of activity-driven emission of PM10 in PRD and NPRD. In PRD, the control-driven emission reductions dramatically improved, from 0.024 Tg in 2009 to 0.318 Tg in 2015, while in NPRD, they improved from 0.014 Tg in 2009 to 0.258 Tg in 2015. In both the PRD and NPRD regions, industrial combustion, power plants, and dust sources were the three major contributors to the control-driven emission reductions.
Compared with control-driven emission reductions in 2015, PM10 emissions in 2020 in PRD and NPRD could be further reduced by 0.31 Tg (60 % of the total PM10 emissions in 2015) and 0.33 Tg (49 % of the total PM10 emissions in 2015), respectively. Fugitive dust is the most significant contributor, accounting for 45 % and 31 % of the total reductions in PRD and NPRD, respectively. This can be achieved by applying online monitoring technology for supervising construction dust (Sun et al., 2016) and more advanced measures, such as achieving a “6 100 %” target for construction sites and increasing the machine cleaning ratio for road dust. Industrial process sources, power plants, and industrial combustion also have major potential to achieve emission reduction, especially industrial process sources in the NPRD.
3.4.4 VOCs
For VOCs, control-driven emission reductions in PRD and NPRD were slight in the past decade. Although VOC emission-control measures, such as promoting emphasis on strict end-of-pipe controls and leak detection and repair (LDAR) technology in VOC-emitting industries, and strengthening oil and gas recovery in gas stations, have been gradually highlighted since 2014 when the action plan for air pollution prevention and control in Guangdong province (2014–2017) (PGGP, 2014) was released, the regulation has not been well executed. Emission reductions from solvent sources, the largest contributor to VOC emissions in PRD (Fig. S6i), were 0.075 Tg in 2015, highly associated with the use of low-VOC products and environmentally friendly paints that contain low or even no VOCs. However, these emission reductions only accounted for 10 %–35 % of the total VOC emission reductions. In fact, 65 %–86 % of the control-driven VOC emission reductions were from on-road mobile sources, which is mainly attributed to the improvement of emission standards and oil quality for vehicles, management of yellow-label cars, and the popularization of green traffic. Even so, the control-driven VOC emission reductions (from 0.016 Tg in 2007 to 0.294 Tg in 2015) were far outweighed by the activity-driven growth in emissions (from 0.699 Tg in 2007 to 1.172 Tg in 2015), resulting from the growth of vehicle populations and increasing use of solvents, which consequently drove up VOC emissions in PRD and NPRD (Fig. 3i–j).
In 2020, if the existing emission control regulations were fully implemented, VOC emissions in PRD would decrease by 30 % relative to the emission level in 2015. The emission reduction potentials are 0.61 Tg in PRD (69 % of the total VOC emissions in 2015) and 0.56 Tg in NPRD (0.2 times higher than total VOC emissions in 2015), respectively, much larger than the emission reduction potentials of SO2, NOx, and PM10. Reduced solvent use is the largest factor. This is because current VOC end-of-pipe removal efficiency in GD is still low. Therefore, VOC emissions from solvent use could be greatly reduced by improving end-of-pipe removal efficiency. In fact, VOC emission controls on solvent use and industrial process sources were particularly prioritized during the 13th FYP (2016–2020). If the VOCs end-of-pipe removal efficiencies achieve their control targets in the 13th FYP, VOCs emission reductions from solvent use will be 0.388 and 0.257 Tg in PRD and NPRD, respectively, accounting for 43 % and 38 % of the total VOC emission reductions. Another source with large potential for emission is on-road mobile source.
This study provides the first long-term record of anthropogenic air pollutant emissions in GD, the three largest city clusters in China, and advances our understating of air pollutant emissions and control measures in Guangdong. The emission trends and their spatial variation were validated by ground-based observations and satellite data. Anthropogenic emissions of most pollutants in GD generally saw downward trends over the 2006–2015 decade, with NH3 and VOC emissions being the exceptions. In that decade, emissions of SO2, PM10, PM2.5, and NOx decreased by 48 %, 22 %, 16 %, and 0.5 %, respectively, despite the significant growth of economic and anthropogenic activity. The decoupling of anthropogenic emissions from economic and energy consumption growth means that emission regulations and control measures on power plants, industrial combustion, on-road mobile sources, and dust sources enacted over the past decade had alleviated emissions. By contrast, because of the absence of effective control measures, NH3 emissions remained stable, while VOC emissions steadily increased by 33 % during 2006–2015.
Because of their discrepancies in emission control progresses, PRD and NPRD showed different emission evolutions. Overall, emissions of SO2, NOx, PM2.5, and PM10 in the PRD region showed significant downward trends during the period of 2006–2015, while emissions from NPRD remained relatively stable due to the lax control measures. Specifically, industrial combustion and industrial process presented opposite trends between PRD and NPRD. In PRD, emissions from industrial combustion declined consistently during 2006–2015, while these emissions in NPRD continued to show an upward trend. Similar to the industrial combustion, emissions from industrial process source also declined in PRD but increased in NPRD during 2006–2015. As a result, emissions in NPRD were increasingly important in GD. The contribution of NPRD to SO2 emissions in GD dramatically increased from 27 % in 2006 to 48 % in 2015. By the end of 2015, emissions of PM10 (56 %), PM2.5 (54 %), and NH3 (68 %) in NPRD already accounted for more than 50 % of the total emissions in GD. Particularly, industrial emission leaks from PRD to NPRD were observed in this study, which partly enhanced the significance of emissions in NPRD. Although the shift of industries reduced PRD emissions, it might not effectively alleviate the air quality in PRD since industrial emissions also have a certain influence on the RPD region, particularly in the winter season (Yin et al., 2017).
In GD, future emission control works should focus on power plants, industrial combustion, and non-road mobile sources to further reduce emissions of SO2, NOx, and particulate matter. This can be achieved by technical innovations consisting of ultra-low emissions in power plants, a series of actions regarding boiler management, control of sulfur content in fuels, flue-gas desulfurization in industries, and special pollution controls for non-metallic mineral industries. In addition, control measures on agricultural sources, the largest contributors of NH3 emissions, should be highlighted. As revealed by Yin et al. (2017), the chemical region in PRD might transit to an ammonia-rich region with a decrease in SO2 and NOx emissions. In this case, a larger reduction in NH3 emissions would be required to further decrease ambient PM2.5 levels in GD. This is feasible since NH3 emissions in GD still have great potential for further reductions. VOC emission reduction is another concern to achieve co-control of PM2.5 and ozone; future work should also focus on VOC emissions. In fact, the reduction of VOC emissions is promising since stringent controls on solvent use were released in Volatile organic compounds (VOCs) remediation and emission reduction work plan in Guangdong Province (2018–2020) (PGGP, 2018). Apart from regulating solvent use, control measures for on-road mobile sources should be enhanced to cancel out the growth of emissions induced by the increase in vehicle population.
The historical emission inventory developed in this study not only helps to understand the emission evolution in GD, but also could help to reveal the dominant causes of air-quality change in PRD. The annual averaged PM2.5 concentrations in PRD showed a decrease in 2006–2015, from 58 µg m−3 in 2007 to 34 µg m−3 in 2017. By contrast, the 90th percentile daily max 8 h average ozone showed a fluctuating increase from 146 µg m−3 in 2007 to 165 µg m−3 in 2017. Our proposed long-term historical inventory might be able to explain the change in PM2.5 and ozone concentrations.
As shown in Fig. 3, emissions of SO2, NOx, and PM2.5 in PRD all steadily fell in this decade. Particularly, SO2 and NOx emissions, the two major precursors of PM2.5 formations, decreased by 63 % and 14 %, respectively, during 2006–2015. This trend of precursor emissions agreed with the declining trend of ambient PM2.5 concentrations. VOC emissions in PRD showed a rising trend, increasing by 35 % during 2006–2015. Ou et al. (2016) had revealed that most parts of PRD formed a VOC-limited region in fall and winter. This suggests that the growing VOC emissions and the decreasing NOx emissions might contribute to the growth of ozone concentrations in PRD. However, this does not mean that emission changes are the dominant cause. Using numerical simulations and the long-term historical emission inventory developed in this study, we can quantify the effectiveness of emission control measures and the impact of meteorological change on air quality in PRD. Consequently, the dominant cause of the increase in ambient ozone concentrations and the downward trend of PM2.5 concentrations in PRD in the recent decade can be identified.
This study applied a top-down method to develop long-term emission inventories. This method is feasible for two reasons. On the one hand, long-term emission inventories generally focus on emission trend, sectoral evolution, and emission projection, rather than the high-resolution spatial distribution. On the other hand, obtaining long-term activity data for the top-down method is more readily accessible. Therefore, most previous studies (Streets et al., 2006; Zhang et al., 2007; Lu et al., 2013; Zheng et al., 2018) also applied the same method to develop long-term emission inventories. However, this study can be further improved in the following aspects. First, a method that is based on fuel consumption might underestimate marine emissions, due to the absence of passing ships that traveled through the study domain but did not call at any port in mainland China (Li et al., 2018). In China, the passing ships accounted for about 7 % of the total fuel consumption. To fix the underestimation, the AIS data can be used to calibrate the total fuel consumption in GD. Second, the annual average vehicle kilometers traveled and the ratio of diesel vehicles to gasoline vehicles were obtained from a field survey, which only covers eight cities and 111 roads in GD. These survey data cannot well represent each city in GD and thus might bring uncertainties in characterizing the emission evolution of on-road mobile sources. To better reflect the temporal pattern and differentiate emissions between different cities in GD, more field surveys of vehicle types, fuel consumption, and emission standards should be conducted to better differentiate vehicle emissions between different cities in GD.
The data can be accessed upon request to the corresponding author.
The supplement related to this article is available online at: https://doi.org/10.5194/acp-19-11701-2019-supplement.
JZ and ZH provided writing ideas with MS support. ZH, YB, and JO carried them out. ZH, JZ, and JO revised and polished the article. YB, ZZ, XX, XY, CL, and YW developed the decadal emission inventories and contributed to discussions of results. LC, YX, ZZ, and XY helped with verification of satellite data. All the authors have made substantial contributions to the work reported in the manuscript.
The authors declare that they have no conflict of interest.
This study was supported by the National Key R&D Program of China (no. 2018YFC0213902). The authors thank the Institute of Remote Sensing and Digital Earth, Chinese Academy of Sciences, for providing the satellite data.
This research has been supported by the National Key R&D Program of China (grant no. 2018YFC0213902).
This paper was edited by Dominick Spracklen and reviewed by two anonymous referees.
Che, W. W., Zheng, J. Y., and Zhong, L. J.: Vehicle Exhaust Emission Characteristics and Contributions in the Pearl River Delta Region, Research of Environmental Sciences, 22, 456–461, 2009.
China Southern Power Grid (CSPG): Power plants (≥6000 kw ⋅ h) and technology & economy indexes in Guangdong province, Guangdong Provincial Power Grid Development Institute, Guangdong Province, China, 2006–2014.
Chun, Y.: Restructuring the export-oriented industrialization in the Pearl River Delta, China: Institutional evolution and emerging tension, Appl. Geogr., 32, 143–157, https://doi.org/10.1016/j.apgeog.2010.10.013, 2012.
EPGD: Reports on the state of Guangdong Provincial environment, available at: http://gdee.gd.gov.cn/hjzkgb/index.html (last access: 3 September 2019), 2006–2017 (in Chinese).
GDEI: Industrial park transferred from PRD to NPRD in Guangdong province, available at: http://www.gdei.gov.cn/zwgk/bmfw/201212/t20121210_109359.htm (last access: 4 September 2019), 2014 (in Chinese).
GDEMC, EPDHK, and EPBMC: Guangdong-Hong Kong-Macao Pearl River Delta Regional Air Quality Monitoring Network Re-ports of Monitoring Results in 2006–2015, available at: http://gdee.gd.gov.cn/kqjc/index.html (last access: 3 September 2019), 2007–2016.
GDEMC, EPDHK, and EPBMC: Guangdong-Hong Kong-Macao Pearl River Delta Regional Air Quality Monitoring Network Reports of Monitoring Results in 2017, available at: http://www.gdep.gov.cn/hjjce/kqjc/?sTop=0 (last access: 3 September 2019), 2018.
GDMS: Guangdong Meteorological Statistical Yearbook, China Statistics Press, Beijing, 2007–2016.
GDPBS: Guangdong Statistical Yearbook, China Statistics Press, Beijing, 2007–2016.
GDPBS: Guangdong Statistical Yearbook, China Statistics Press, Beijing, 2018.
GDEMC, EPDHK, and EPBMC: Guangdong-Hong Kong-Macao Pearl River Delta Regional Air Quality Monitoring Network Re-ports of Monitoring Results in 2006–2015, available at: http://gdee.gd.gov.cn/kqjc/index.html (last access: 3 September 2019), 2007–2016.
GDPCSY: Guangdong Provincial 21 City Statistical Yearbooks China Statistics Press, Beijing, 2007–2016.
GDPSR: Guangdong provincial pollutant statistical reports, 2006, 2010, 2012, 2014, 2015.
Gurjar, B. R., Van Aardenne, J. A., Lelieveld, J., and Mohan, M.: Emission estimates and trends (1990–2000) for megacity Delhi and implications, Atmos. Environ., 38, 5663–5681, https://doi.org/10.1016/j.atmosenv.2004.05.057, 2004.
He, M., Zheng, J. Y., Yin, S. S., and Zhang, Y. Y.: Trends, temporal and spatial characteristics, and uncertainties in biomass burning emissions in the Pearl River Delta, China, Atmos. Environ., 45, 4051–4059, https://doi.org/10.1016/j.atmosenv.2011.04.016, 2011.
Huang, Z. J., Wang, S. S., Zheng, J. Y., Yuan, Z. B., Ye, S. Q., and Kang, D.: Modeling inorganic nitrogen deposition in Guangdong province, China, Atmos. Environ., 109, 147–160, https://doi.org/10.1016/j.atmosenv.2015.03.014, 2015.
International Maritime Organization (IMO): Information on North American Emission Control Area 25 under MARPOL Annex VI, available at: http://www.imo.org (last access: 4 September 2019), 2009.
International Sustainable Systems Research Center (ISSRC): IVE model user's manual version 2.0, available at: http://www.issrc.org/ive/ (last access: 4 September 2019), 2008.
Kurokawa, J., Ohara, T., Morikawa, T., Hanayama, S., Janssens-Maenhout, G., Fukui, T., Kawashima, K., and Akimoto, H.: Emissions of air pollutants and greenhouse gases over Asian regions during 2000–2008: Regional Emission inventory in ASia (REAS) version 2, Atmos. Chem. Phys., 13, 11019–11058, https://doi.org/10.5194/acp-13-11019-2013, 2013.
Li, K., Jacob, D. J., Liao, H., Shen, L., Zhang, Q., and Bates, K. H.: Anthropogenic drivers of 2013–2017 trends in summer surface ozone in china, Proc. Natl. Acad. Sci., 116, 422–427, https://doi.org/10.1073/pnas.1812168116, 2019.
Li, C., Borken-Kleefeld, J., Zheng, J., Yuan, Z., Ou, J., Li, Y., Wang, Y., and Xu, Y.: Decadal evolution of ship emissions in China from 2004 to 2013 by using an integrated AIS-based approach and projection to 2040, Atmos. Chem. Phys., 18, 6075–6093, https://doi.org/10.5194/acp-18-6075-2018, 2018.
Lin, C. Q., Li, Y., Lau, A., Li, C. C., and Fung, J. C. H.: 15-year PM2.5 trends in the Pearl River Delta region and Hong Kong from satellite observation, Aerosol Air Qual. Res., 18, 2355–2362, https://doi.org/10.4209/aaqr.2017.11.0437, 2018.
Liu, Y. H., Liao, W. Y., Li, L., Huang, Y. T., and Xu, W. J.: Vehicle emission trends in China's Guangdong Province from 1994 to 2014, Sci. Total Environ., 586, 512–521, https://doi.org/10.1016/j.scitotenv.2017.01.215, 2017.
Liu, Y. M., Zhang, S. T., Fan, Q., Wu, D., Chan, P., Wang, X. M., Fan, S. J., Feng, Y. R., and Hong, Y. Y.: Accessing the Impact of Sea-Salt Emissions on Aerosol Chemical Formation and Deposition over Pearl River Delta, China, Aerosol Air Qual. Res., 15, 2232–2245, https://doi.org/10.4209/aaqr.2015.02.0127, 2015.
Lu, Q., Zheng, J. Y., Ye, S. Q., Shen, X. L., Yuan, Z. B., and Yin, S. S.: Emission trends and source characteristics of SO2, NOx, PM10 and VOCs in the Pearl River Delta region from 2000 to 2009, Atmos. Environ., 76, 11–20, https://doi.org/10.1016/j.atmosenv.2012.10.062, 2013.
Mao, L., Liu, R., Liao, W. H., Wang, X. M., Shao, M., Chen Liu, S., and Zhang, Y. H.: An observation-based perspective of winter haze days in four major polluted regions of China, Natl. Sci. Rev., 6, 515–523, https://doi.org/10.1093/nsr/nwy118, 2018.
MEPC: The guideline of air pollutant emission inventory development for Chinese cities, available at: http://www.mee.gov.cn/ (last access: 3 September 2019), 2017.
Ohara, T., Akimoto, H., Kurokawa, J., Horii, N., Yamaji, K., Yan, X., and Hayasaka, T.: An Asian emission inventory of anthropogenic emission sources for the period 1980–2020, Atmos. Chem. Phys., 7, 4419–4444, https://doi.org/10.5194/acp-7-4419-2007, 2007.
Ou, J. Y., Yuan, Z. B., Zheng, J. Y., Huang, Z. J., Shao, M., Li, Z. K., Huang, X. B., Guo, H., and Louie, P. K. K.: Ambient Ozone Control in a Photochemically Active Region: Short-Term Despiking or Long-Term Attainment? Environ. Sci. Technol., 50, 5720–5728, https://doi.org/10.1021/acs.est.6b00345, 2016.
Pan, Y. Y., Li, N., Zheng, J. Y., Yin, S. S., Li, C., Yang, J., Zhong, L. J., Chen, D. H., Deng, S. X., and Wang, S. S.: Emission inventory and characteristics of anthropogenic air pollutant sources in Guangdong Province, Acta Scientiae Circumstantiae, 59, 133–135, https://doi.org/10.13671/j.hjkxxb.2014.1058, 2015.
PCAP: Action Plan on the Prevention and Control of Air Pollution. available at: http://www.jingbian.gov.cn/gk/zfwj/gwywj/41211.htm?from=timeline (last access: 4 September 2019), 2013–2017 (in Chinese).
PGGP: The National III standard was implemented in Guangdong province since July 1, available at: http://www.gd.gov.cn/gdgk/gdyw/200904/t20090424_90255.htm (last access: 4 September 2019), 2009 (in Chinese).
PGGP: Planning for Guangdong province environmental protection and ecological construction in 12th Five-Year, available at: http://zwgk.gd.gov.cn/006939748/201108/t20110805_201861.html (last access: 4 September 2019), 2011 (in Chinese).
PGGP: The action plan for air pollution prevention and control in Guangdong province (2014–2017), available at: http://zwgk.gd.gov.cn/006939748/201402/t20140214_467051.html (last access: 3 September 2019), 2014 (in Chinese).
PGGP: Volatile organic compounds (VOCs) remediation and emission reduction work plan in Guangdong Province (2018–2020), available at: http://zwgk.gd.gov.cn/006940060/201807/t20180709_772857.html, (last access: 4 September 2019), 2018 (in Chinese).
Streets, D. G., Zhang, Q., Wang, L. T., He, K. B., Hao, J. M., Wu, Y., Tang, Y. H., and Carmichael, G. R.: Revisiting China's CO emissions after the Transport and Chemical Evolution over the Pacific (TRACE-P) mission: Synthesis of inventories, atmospheric modeling, and observations, J. Geophys. Res.-Atmos., 111, D14306, https://doi.org/10.1029/2006JD007118, 2006.
Sun, M., Gao, X., Liu, M. H., Zhang, J., Zhan, X. H., Liu, J. H., and Xu, Y.: Application of On-line Monitoring in Construction Site Dust Supervision, Environmental Science and Management, 41, 142–145, 2016.
Tao, J., Zhang, L., Cao, J., and Zhang, R.: A review of current knowledge concerning PM2.5 chemical composition, aerosol optical properties and their relationships across China, Atmos. Chem. Phys., 17, 9485–9518, https://doi.org/10.5194/acp-17-9485-2017, 2017.
Wang, X. L., Yu, Y. F., Huang, Z. J., Cai, H. H., Li, C., Zhou, M., Liu, L. Y., Luo, C., Zhuang, Y. J., and Zheng, J. Y.: Improvement and Research on Industrial VOC Emission Inventory in Guangdong Province Based on the Field Investigation, Acta Scientiae Circumstantiae, 39, 1013–1024, https://doi.org/10.13671/j.hjkxxb.2018.0431, 2019.
World Health Organization (WHO): Air Quality Guidelines, available at: https://www.who.int/phe/health_topics/outdoorair/outdoorair_aqg/en/ (last access: 4 September 2019), 2006.
Yang, B. J. and Mao, Y. H.: Industrial Relocation Policy and Firm Migration: an Empirical Analysis from Guangdong Industrial Relocation Survey Data, South China Journal of Economics, 3, 1000–6249, https://doi.org/10.19592/j.cnki.scje.2014.03.001, 2014.
Yang, C.: Restructuring the export-oriented industrialization in the Pearl River Delta, China: institutional evolution and emerging tension, Appl. Geogr., 32, 143–157, https://doi.org/10.1016/j.apgeog.2010.10.013, 2012.
Yang, L., Luo, H., Yuan, Z., Zheng, J., Huang, Z., Li, C., Lin, X., Louie, P. K. K., and Chen, D.: Quantitative impacts of meteorology and precursor emission changes on the long-term trend of ambient ozone over the Pearl River Delta, China and implications for ozone control strategy, Atmos. Chem. Phys. Discuss., https://doi.org/10.5194/acp-2019-355, in review, 2019.
Yang, Y.: Character, Level and Regulatory Measures Study of Fugitive Dust Emissions from Building Construction Sites in PRD, South China University of Technology, Guangzhou, China, 2014.
Yin, S. S., Zheng, J. Y., Lu, Q., Yuan, Z. B., Huang, Z. J., Zhong, L. J., and Lin, H.: A refined 2010-based VOC emission inventory and its improvement on modeling regional ozone in the Pearl River Delta Region, China, Sci. Total Environ., 514, 426–438, https://doi.org/10.1016/j.scitotenv.2015.01.088, 2015.
Yin, X. H., Huang, Z. J., Zheng, J. Y., Yuan, Z. B., Zhu, W. B., Huang, X. B., and Chen, D. H.: Source contributions to PM2.5 in Guangdong province, China by numerical modeling: Results and implications, Atmos. Res., 186, 63–71, https://doi.org/10.1016/j.atmosres.2016.11.007, 2017.
Zhang, Q., Streets, D. G., He, K. B., Wang, Y. X., Richter, A., Burrows, J. P., Uno, I., Jang, C. J., Chen, D., and Yao, Z. L.: NOx emission trends for China, 1995–2004: The view from the ground and the view from space, J. Geophys. Res.-Atmos., 112, D22306, https://doi.org/10.1029/2007JD008684, 2007.
Zhang, Y. S., Shao, M., Lin, Y., Luan, S. J., Mao, N., Chen, W. T., and Wang, M.: Emission inventory of carbonaceous pollutants from biomass burning in the Pearl River Delta Region, China, Atmos. Environ., 76, 189–199, https://doi.org/10.1016/j.atmosenv.2012.05.055, 2013.
Zheng, B., Tong, D., Li, M., Liu, F., Hong, C., Geng, G., Li, H., Li, X., Peng, L., Qi, J., Yan, L., Zhang, Y., Zhao, H., Zheng, Y., He, K., and Zhang, Q.: Trends in China's anthropogenic emissions since 2010 as the consequence of clean air actions, Atmos. Chem. Phys., 18, 14095–14111, https://doi.org/10.5194/acp-18-14095-2018, 2018.
Zheng, J. Y., Shao, M., Che, W. W., Zhang, L. J., Zhong, L. J., Zhang, Y. H., and Streets, D.: Speciated VOC emission inventory and spatial patterns of ozone formation potential in the Pearl River Delta, China, Environ. Sci. Technol., 43, 8580–8586, https://doi.org/10.1021/es901688e, 2009a.
Zheng, J. Y., Zhang, L. J., Che, W. W., Zheng, Z. Y., and Yin, S. S.: A highly resolved temporal and spatial air pollutant emission inventory for the Pearl River Delta region, China and its uncertainty assessment, Atmos. Environ., 43, 5112–5122, https://doi.org/10.1016/j.atmosenv.2009.04.060, 2009b.
Zheng, J. Y., Zheng, Z. Y., Yu, Y. F., and Zhong, L. J.: Temporal, spatial characteristics and uncertainty of biogenic VOC emissions in the Pearl River Delta region, China, Atmos. Environ., 44, 1960–1969, https://doi.org/10.1016/j.atmosenv.2010.03.001, 2010.
Zhong, L. J., Louie, P. K. K., Zheng, J. Y., Yuan, Z. B., Yue, D. L., Ho, J. W. K., and Lau, A. K. H.: Science-policy interplay: Air quality management in the Pearl River Delta region and Hong Kong, Atmos. Environ., 76, 3–10, https://doi.org/10.1016/j.atmosenv.2013.03.012, 2013.
Zhong, Z. M., Zheng, J. Y., Zhu, M. N., Huang, Z. J., Zhang, Z. W., Jia, G. L., Wang, X. L., Bian, Y. H., Wang, Y. L., and Li, N.: Recent developments of anthropogenic air pollutant emission inventories in Guangdong province, China, Sci. Total Environ., 627, 1080–1092, https://doi.org/10.1016/j.scitotenv.2018.01.268, 2018.